Understanding the perceptron
First, we need to understand the basics of neural networks. A neural consists of one or multiple layers of neurons, named after the neurons in human brains. We will demonstrate the mechanics of a single neuron by implementing a perceptron. In a perceptron, a single unit (neuron) performs all the computations. Later, we will scale the number of units to create deep neural networks:
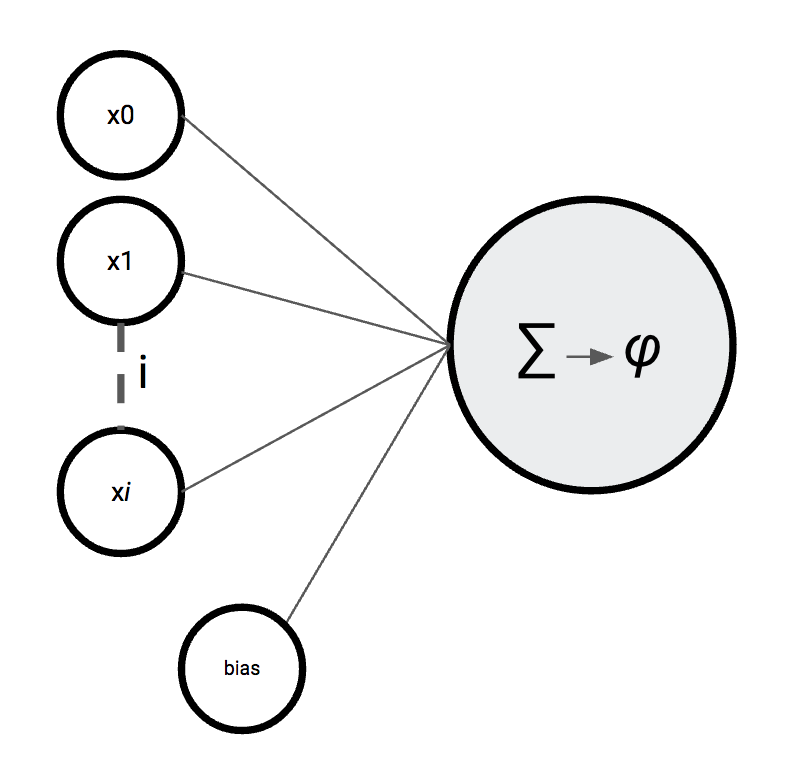
Figure 2.1: Perceptron
A can have multiple inputs. On these inputs, the unit performs some computations and outputs a single value, for example a binary value to classify two classes. The computations performed by the unit are a simple matrix multiplication of the input and the weights. The resulting values are summed up and a bias is added:
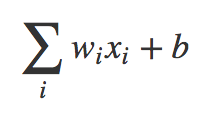
These computations can easily be scaled to high dimensional input. An activation function (φ) determines the final output of the in the forward pass:
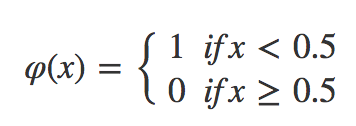
The weights and bias are initialized. After each epoch (iteration over the training data), the...