Most real-world datasets are messy and noisy. Due to their messiness and noise, lots of values are either faulty or missing. pandas offers lots of built-in functions to deal with missing values in DataFrames:
- Check missing values in a DataFrame: pandas' isnull() function checks for the existence of null values and returns True or False, where True is for null and False is for not-null values. The sum() function will sum all the True values and returns the count of missing values. We have tried two ways to count the missing values; both show the same output:
# Count missing values in DataFrame
pd.isnull(df).sum()
The following is the second method:
df.isnull().sum()
This results in the following output:
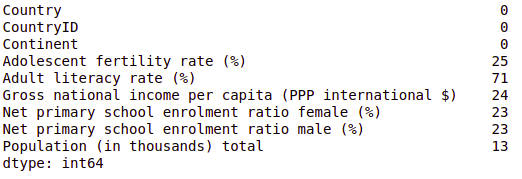
- Drop missing values: A very naive approach to deal with missing values is to drop them for analysis purposes. pandas has the dropna() function to drop or delete such observations from the DataFrame. Here, the inplace=True attribute makes the changes in...