Fast R-CNN – fast region-based CNN
Fast R-CNN, or Fast Region-based CNN method, is an improvement over the previously covered R-CNN. To be precise about the improvement statistics, as compared to R-CNN, it is:
- 9x faster in training
- 213x faster at scoring/servicing/testing (0.3s per image processing), ignoring the time spent on region proposals
- Has higher mAP of 66% on the PASCAL VOC 2012 dataset
Where R-CNN uses a smaller (five-layer) CNN, Fast R-CNN uses the deeper VGG16 network, which accounts for its improved accuracy. Also, R-CNN is slow because it performs a ConvNet forward pass for each object proposal without sharing computation:
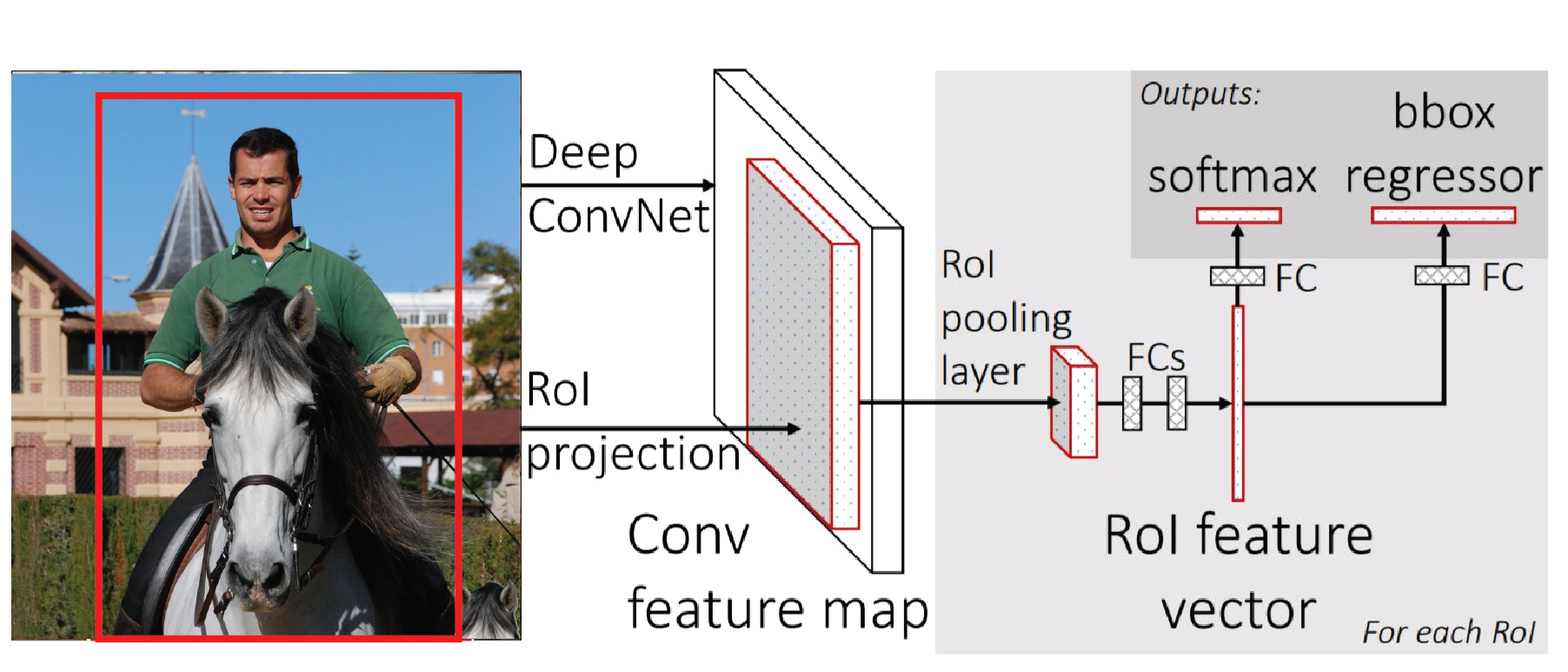
Fast R-CNN: Working
In Fast R-CNN, the deep VGG16 CNN provides essential computations for all the stages, namely:
- Region of Interest (RoI) computation
- Classification Objects (or background) for the region contents
- Regression for enhancing the bounding box
The input to the CNN, in this case, is not raw (candidate) regions from the image, but the (complete) actual image...