From Bag-of-Words to the Transformers
Over the past two decades, there have been significant advancements in the field of natural language processing (NLP). We have gone through various paradigms and have now arrived at the era of the Transformer architecture. These advancements have helped us represent words or sentences more effectively in order to solve NLP tasks. On the other hand, different use cases of merging textual inputs to other modalities, such as images, have emerged as well. Conversational artificial intelligence (AI) has seen the dawn of a new era. Chatbots were developed that act like humans by answering questions, describing concepts, and even solving mathematical equations step by step. All of these advancements happened in a very short period. One of the enablers of this huge advancement, without a doubt, was Transformer models.
Finding a cross-semantic understanding of different natural languages, natural languages and images, natural languages, and programming languages, and even in a broader sense, natural languages and almost any other modality, has opened a new gate for us to be able to use natural language as our primary input to perform many complex tasks in the field of AI. The easiest imaginable way is to just describe what we are looking for in a picture so the model will give us what we want (https://huggingface.co/spaces/CVPR/regionclip-demo):
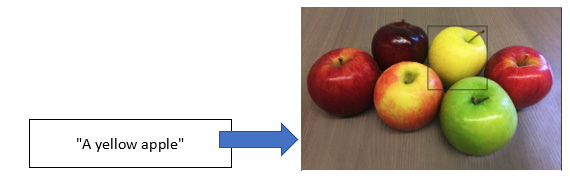
Figure 1.1 – Zero-shot object detection with the prompt “A yellow apple”
The models have developed this skill through a process of ongoing learning and improvement. At first, distributional semantics and n-gram language models were traditionally utilized to understand the meanings of words and documents for years. It has been seen that these approaches had several limitations. On the other hand, with the rise of newer approaches for diffusing different modalities, modern approaches for training language models, especially large language models (LLMs), enabled many different use cases to come to life.
Classical deep learning (DL) architectures have significantly enhanced the performance of NLP tasks and have overcome the limitations of traditional approaches. Recurrent neural networks (RNNs), feed-forward neural networks (FFNNs), and convolutional neural networks (CNNs) are some of the widely used DL architectures for the solution. However, these models have also faced their own challenges. Recently, the Transformer model became standard, eliminating all the shortcomings of other models. It differed not only in solving a single monolingual task but also in the performance of multilingual, multitasking tasks. These contributions have made transfer learning (TL) more viable in NLP, which aims to make models reusable for different tasks or languages.
In this chapter, we will begin by examining the attention mechanism and provide a brief overview of the Transformer architecture. We will also highlight the distinctions between Transformer models and previous NLP models.
In this chapter, we will cover the following topics:
- Evolution of NLP approaches
- Recalling traditional NLP approaches
- Leveraging DL
- Overview of the Transformer architecture
- Using TL with Transformers
- Multimodal learning