The architecture of different neural networks
Neural networks come in various types, each with a specific architecture suited to a different kind of task. The following list contains general descriptions of some of the most common types:
- Feedforward neural network (FNN): This is the most straightforward type of neural network. Information in this network moves in one direction only, from the input layer through any hidden layers to the output layer. There are no cycles or loops in the network; it’s a straight, “feedforward” path.
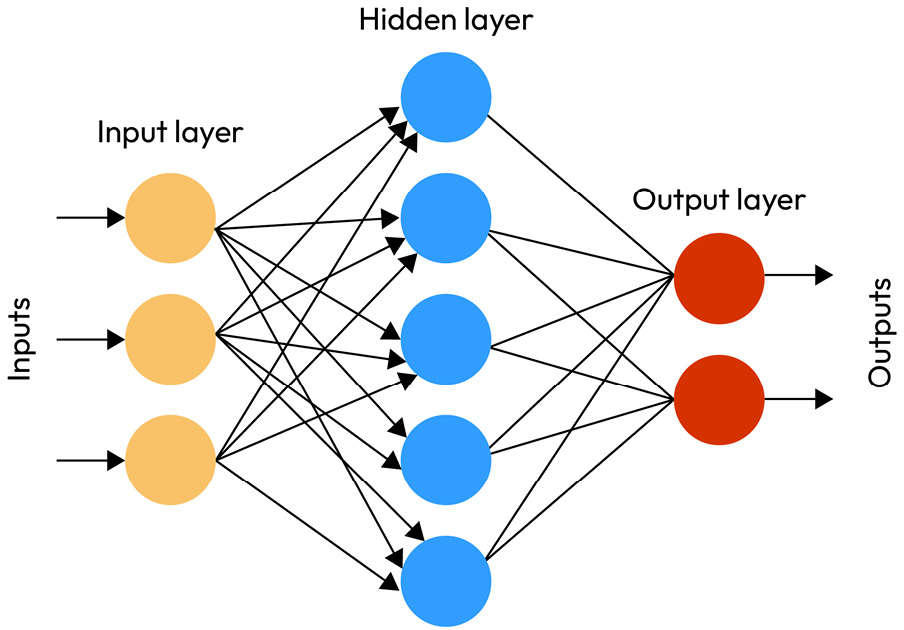
Figure 6.2 – Feedforward neural network
- Multilayer perceptron (MLP): An MLP is a type of feedforward network that has at least one hidden layer in addition to its input and output layers. The layers are fully connected, meaning each neuron in a layer connects with every neuron in the next layer. MLPs can model complex patterns and are widely used for tasks such as image recognition, classification, speech recognition, and other types of machine learning tasks. The MLP is a feedforward network with layers of neurons arranged sequentially. Information flows from the input layer through hidden layers to the output layer in one direction:
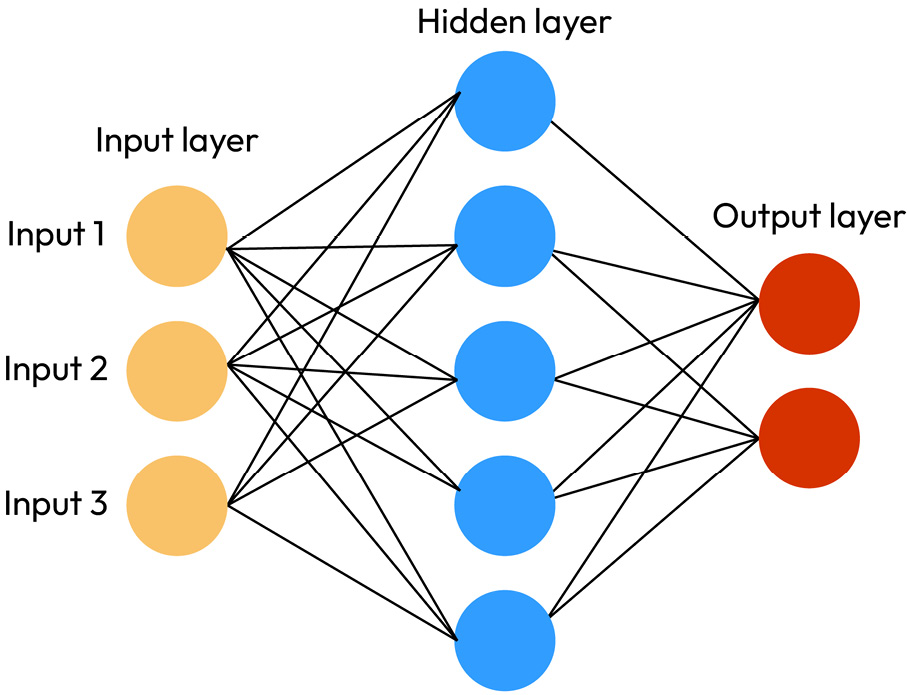
Figure 6.3 – Multilayer perceptron
- CNN: A CNN is particularly well-suited to tasks involving spatial data, such as images. Its architecture includes three main types of layers: convolutional layers, pooling layers, and fully connected layers. The convolutional layers apply a series of filters to the input, which allows the network to automatically and adaptively learn spatial hierarchies of features. Pooling layers decrease the spatial size of the representation, thereby reducing parameters and computation in the network to control overfitting and decrease the computation cost in the following layers. Fully connected layers get the output of the pooling layer and conduct high-level reasoning on the output.
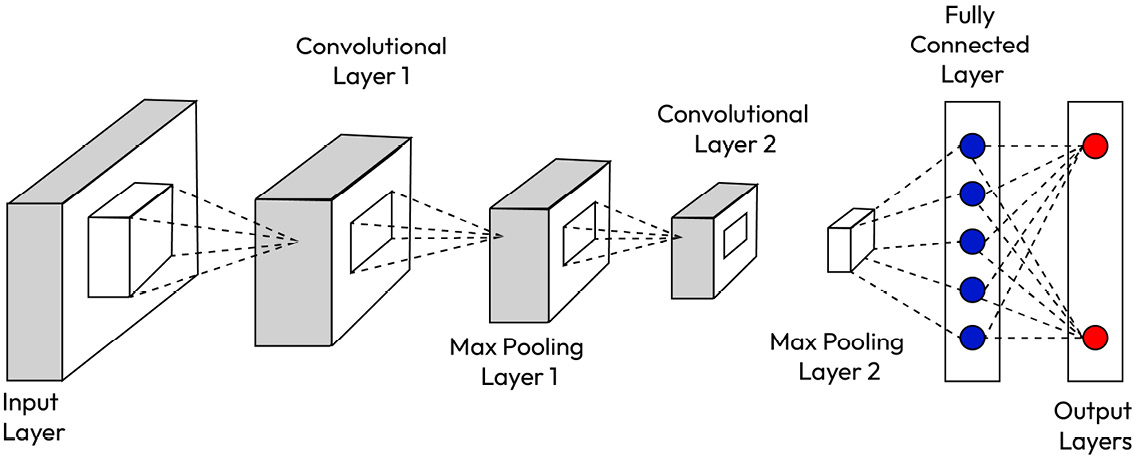
Figure 6.4 – Convolutional neural network
- Recurrent neural network (RNN): Unlike feedforward networks, RNNs have connections that form directed cycles. This architecture allows them to use information from their previous outputs as inputs, making them ideal for tasks involving sequential data, such as time series prediction or NLP. A significant variation of RNNs is the LSTM network, which uses special units in addition to standard units. RNN units include a "memory cell" that can maintain information in memory for long periods of time, a feature that is particularly useful for tasks that require learning from long-distance dependencies in the data, such as handwriting or speech recognition.
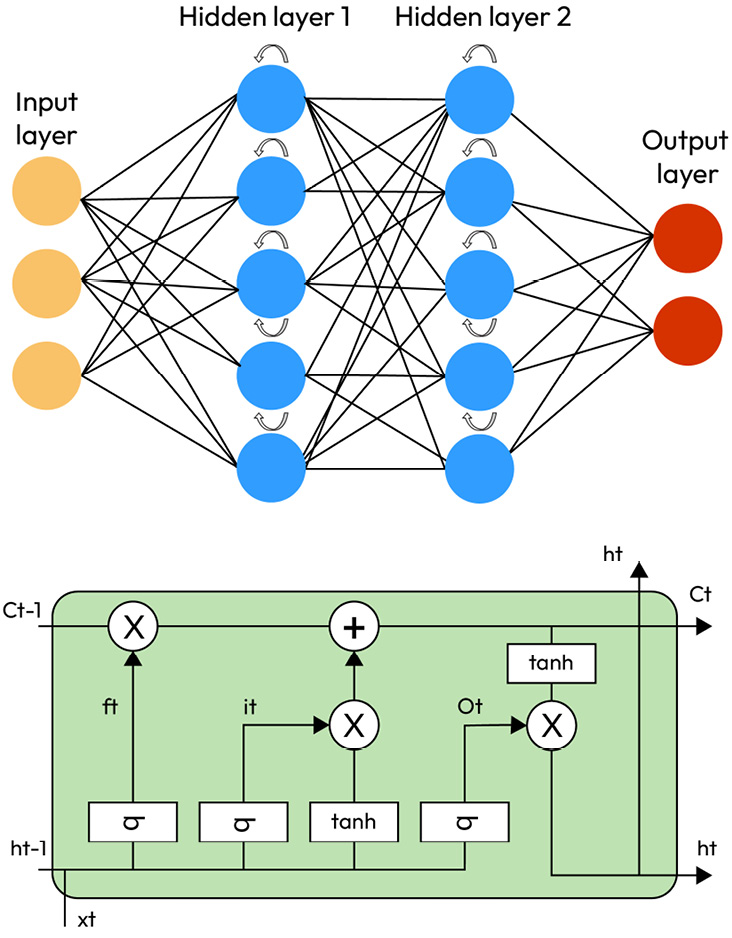
Figure 6.5 – Recurrent neural network
- Autoencoder (AE): An AE is a type of neural network used to learn the efficient coding of input data. It has a symmetrical architecture and is designed to apply backpropagation, setting the target values to be equal to the inputs. Autoencoders are typically used for feature extraction, learning representations of data, and dimensionality reduction. They’re also used in generative models, noise removal, and recommendation systems.
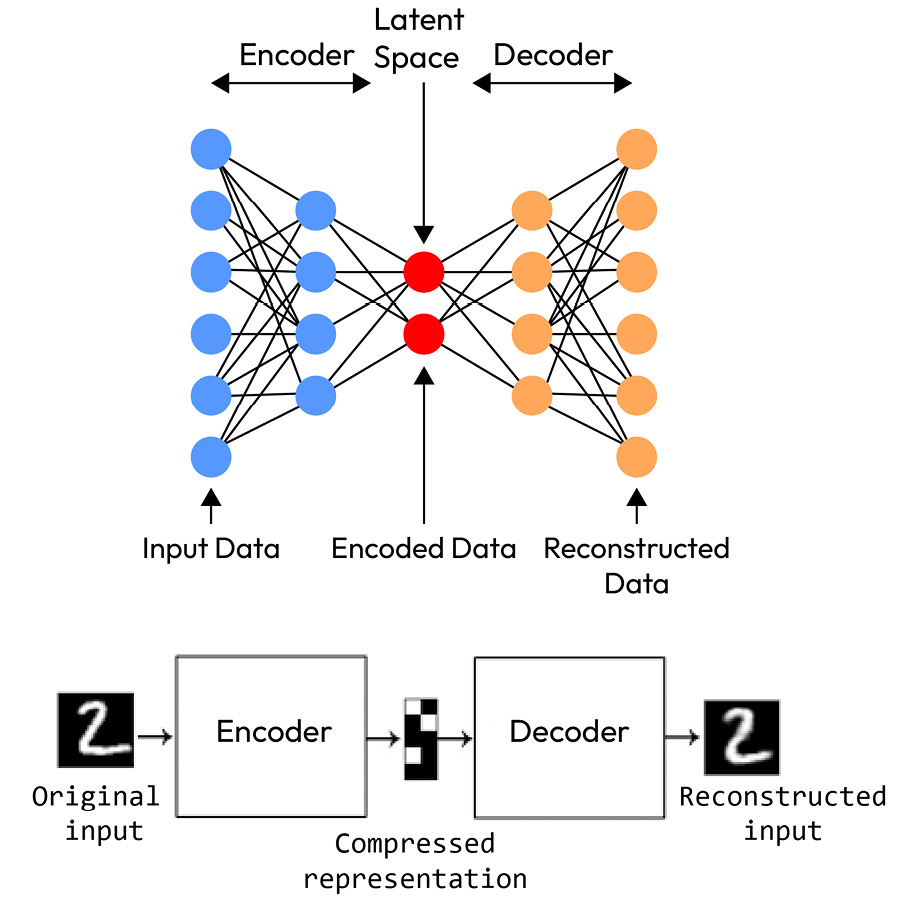
Figure 6.6 – Autoencoder architecture
- Generative adversarial network (GAN): A GAN consists of two parts, a generator and a discriminator, which are both neural networks. The generator creates data instances that aim to come from the same distribution as the training dataset. The discriminator’s goal is to distinguish between instances from the true distribution and instances from the generator. The generator and the discriminator are trained together, with the goal that the generator produces better instances as training progresses, whereas the discriminator becomes better at distinguishing true instances from generated ones.

Figure 6.7 – Generative adversarial network in computer vision
These are just a few examples of neural network architectures, and many variations and combinations exist. The architecture you choose for a task will depend on the specific requirements and constraints of your task.