The stacked hourglass model was developed in 2016 by Alejandro Newell, Kaiyu Yang, and Jia Deng in their paper titled Stacked Hourglass Networks for Human Pose Estimation. The details of the model can be found at https://arxiv.org/abs/1603.06937.
The architecture of the model is illustrated in the following diagram:
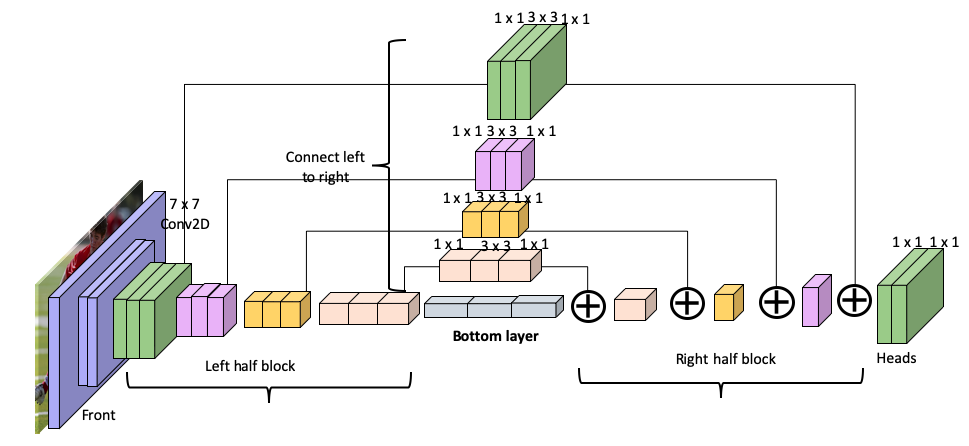
The key features of this model are as follows:
- Bottom-up and top-down processing of the feature is repeated across all scales by stacking multiple hourglasses together. This method results in being able to verify the initial estimates and features across the whole image.
- The network uses multiple convolutions and a max pooling layer, which results in a low final resolution, before upsampling to bring the resolution back up.
- At each max pooling step, additional convolutional layers are added parallel to the main...