One of the ways to compute the expectation of a joint probability distribution is to generate a lot of samples from the joint probability distribution by Gibbs sampling and then take the mean value of the samples as the expected value. In Gibbs sampling, each of the variables in the joint probability distribution can be sampled, conditioned on the rest of the variables. Since the visible units are independent, given the hidden units and vice versa, you can sample the hidden unit as and then the visible unit activation given the hidden unit as
. We can then take the sample
as one sampled from the joint probability distribution. In this way, we can generate a huge number of samples, say M, and take their mean to compute the desired expectation. However, doing such extensive sampling in each step of gradient descent is going to make the training process unacceptably...




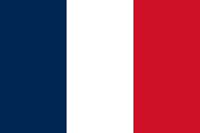


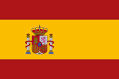



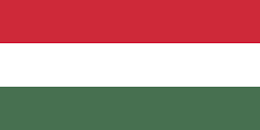

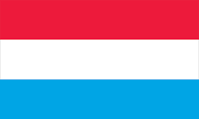





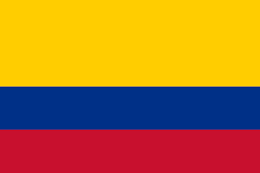
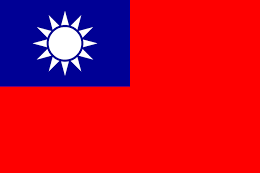
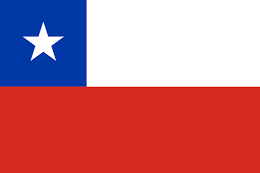



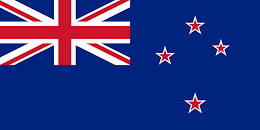
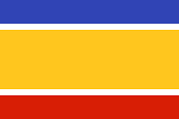
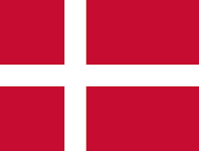
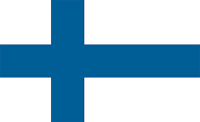







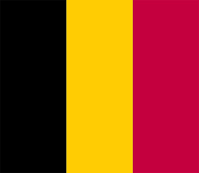

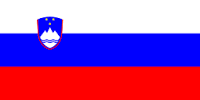





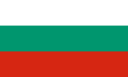


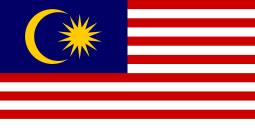



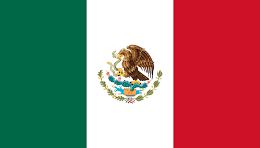
