In this chapter, we looked at a fundamental component of RL, and that is rewards. We learned that, when building training environments, it was best that we defined a set of reward functions our agent will live by. By understanding these equations, we get a better sense of how frequent or sparse rewards can negatively affect training. We then looked at a few methods, the first of which is called Curriculum Learning, that could be used to ease or step the agent's extrinsic rewards. After that, we explored another technique, called Backplay, that used a reverse play technique and Curriculum Training to enhance an agent's training. Finally, we looked at internal or intrinsic rewards, and the concept of Motivated Reinforcement Learning. We then learned that the first intrinsic reward system developed into ML-Agents was to give an agent a motivation for curiosity....




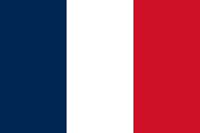


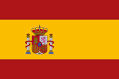




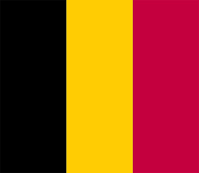
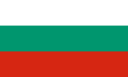
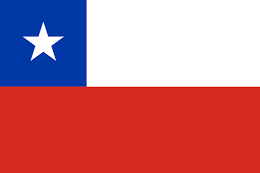
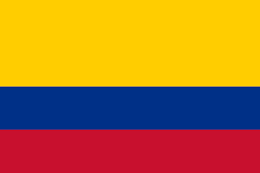
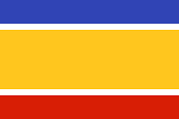

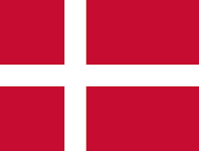



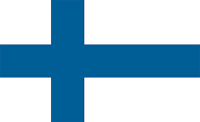

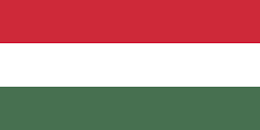






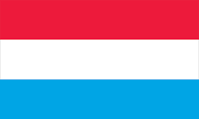
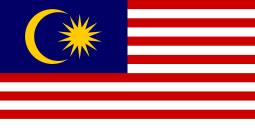

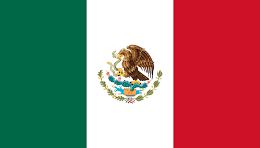

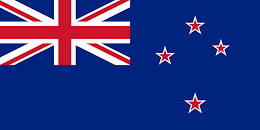







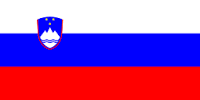




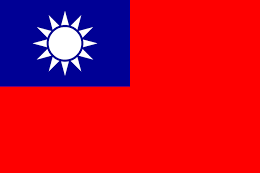


