Improving the speed of Python code in HFT
The critical components we defined during the previous chapters must run at high speed. Using any of the tools we described previously will help you create C/C++-like code and create performant Python code using libraries. It is essential to begin constructing a new algorithm in Python utilizing NumPy and SciPy while avoiding looping code by leveraging the vectorized idioms of both libraries. In reality, this means attempting to replace any nested for
loops with similar calls to NumPy array functions. The purpose is to prevent the CPU from wasting time on the Python interpreter instead of crunching numbers for trading strategies.
However, there are situations when an algorithm cannot be efficiently expressed in simple vectorized NumPy code. The following is the recommended method in this case:
- Find the primary bottleneck in the Python implementation and isolate it in a dedicated module-level function.
- If there is a small but well-maintained C/C++ version of the same algorithm, you may develop a Cython wrapper for it and include a copy of the library's source code. Or you can use any of the other techniques we talked about previously.
- Place the Python version of the function in the tests and use it to ensure that the built extension's results match the gold standard, easy-to-debug Python version.
- Check whether it is possible to create coarse-grained parallelism for multi-processing by utilizing the
joblib.Parallel
class once the code has been optimized (not a simple bottleneck spottable by profiling).
Figure 10.3 depicts the function calls to C++ when there is a need for low-latency functions for HFT:
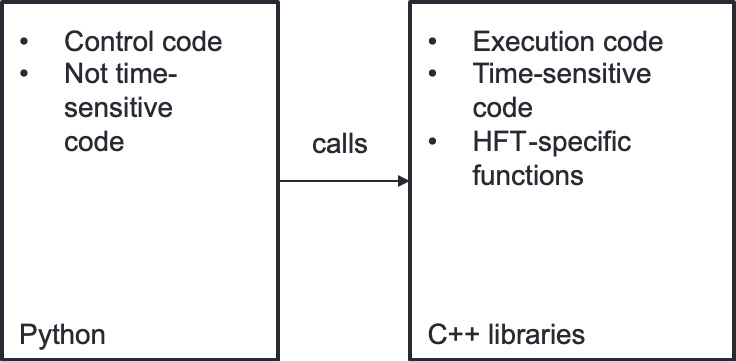
Figure 10.3 – Python and C++ interactions
The control code, which could be used to decide to liquidate a position, can be calculated in Python. C++ will be in charge of the execution of the liquidation by using the speed of these libraries.
The critical components include the following:
- Limit order book
- Order manager
- Gateways
- HFT execution algorithm
All these should be implemented in C/C++ or Cython.
Some companies that have compiler engineers invest in C++ code generation from Python. We will have a tool parsing Python code and generating C++ code in this situation. This C++ code will be compiled and run like any other code, and Python code, in general, can be used for coding trading strategies. Most people in charge of creating trading strategies have more Python skills than C++ knowledge. Therefore, it is easier for them to develop everything in Python than convert Python into C++ libraries used in the C++ trading system.
In this section, we learned how to improve Python code using C++. We will now wrap up this chapter.