Data science strategy
If data science is to continue to grow and graduate into a core business activity, companies must find a way to scale it across all layers of the organization and overcome all the difficult challenges we discussed earlier. To get there, we identified three important pillars that architects planning a data science strategy should focus on, namely, data, services, and tools:
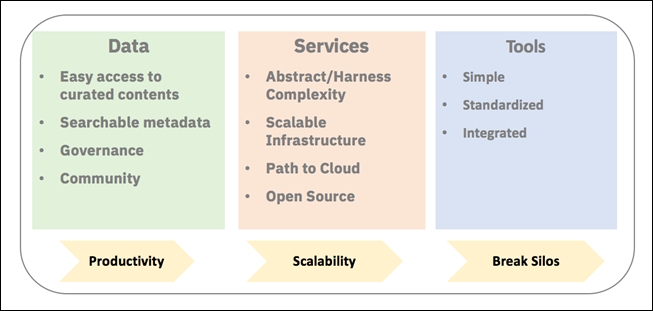
Three pillars of dat science at scale
- Data is your most valuable resource: You need a proper data strategy to make sure data scientists have easy access to the curated contents they need. Properly classifying the data, set appropriate governance policies, and make the metadata searchable will reduce the time data scientists spend acquiring the data and then asking for permission to use it. This will not only increase their productivity, it will also improve their job satisfaction as they will spend more time working on doing actual data science.
Setting a data strategy that enables data scientists to easily access high-quality data that's relevant to them increases productivity and morale and ultimately leads to a higher rate of successful outcomes.
- Services: Every architect planning for data science should be thinking about a service-oriented architecture (SOA). Contrary to traditional monolithic applications where all the features are bundled together into a single deployment, a service-oriented system breaks down functionalities into services which are designed to do a few things but to do it very well, with high performance and scalability. These systems are then deployed and maintained independently from each other giving scalability and reliability to the whole application infrastructure. For example, you could have a service that runs algorithms to create a deep learning model, another one would persist the models and let applications run it to make predictions on customer data, and so on.
The advantages are obvious: high reusability, easier maintenance, reduced time to market, scalability, and much more. In addition, this approach would fit nicely into a cloud strategy giving you a growth path as the size of your workload increases beyond existing capacities. You also want to prioritize open source technologies and standardize on open protocols as much as possible.
Breaking processes into smaller functions infuses scalability, reliability, and repeatability into the system.
- Tools do matter! Without the proper tools, some tasks become extremely difficult to complete (at least that's the rationale I use to explain why I fail at fixing stuff around the house). However, you also want to keep the tools simple, standardized, and reasonably integrated so they can be used by less skilled users (even if I was given the right tool, I'm not sure I would have been able to complete the house fixing task unless it's simple enough to use). Once you decrease the learning curve to use these tools, non-data scientist users will feel more comfortable using them.
Making the tools simpler to use contributes to breaking the silos and increases collaboration between data science, engineering, and business teams.