In this chapter, we showed how to use a convolutional neural network (CNN) deep learning model for image recognition and classification. We made use of the popular fashion-MNIST data for training and testing the image classification model. We also went over calculations involving a number of parameters, and were able to contrast this with the number of parameters that would have been needed by a densely connected neural network. CNN models help to significantly reduce the number of parameters needed and thus result in significant savings in computing time and resources. We also used images of fashion items downloaded from the internet to see whether a classification model based on fashion-MNIST data can be generalized to similar items. We did notice that it is important to maintain consistency in the way images are laid out in the training data. Additionally, we also showed...




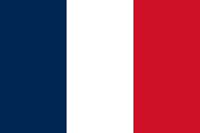


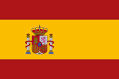



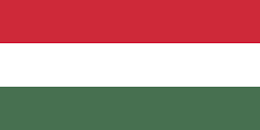

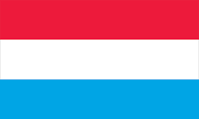





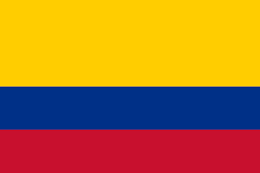
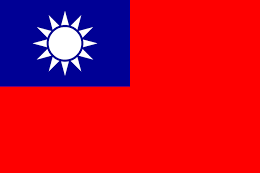
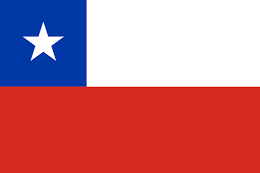



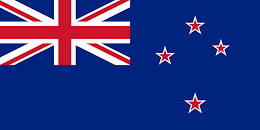
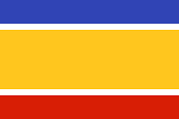
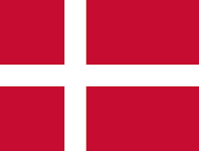
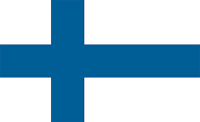







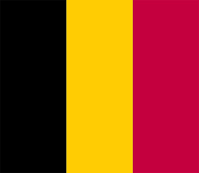

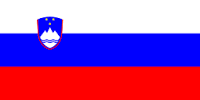





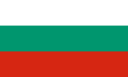


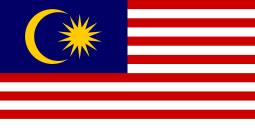



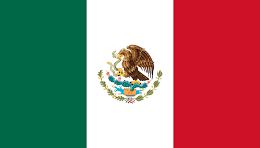
