Using SQL for data transformations
SQL is an essential tool in data analysis and transformations. Many data pipelines you see at workplaces are written in SQL and most data professionals are accustomed to using SQL for analytics work.
The good news is that you can use SQL in Python Polars as well. This opens the door for those who might not be as familiar with a DataFrame library. In this recipe, we’ll cover how to configure Polars to use SQL and how you can implement simple SQL queries such as aggregations.
Getting ready
We’ll use the Contoso dataset for this recipe as well. Run the following code to read the dataset:
df = pl.read_csv('../data/contoso_sales.csv', try_parse_dates=True)
How to do it…
Here’s how to use SQL in Polars:
- Define the SQL context and register your DataFrame:
ctx = pl.SQLContext(eager=True) ctx.register('df', df)
- Create a simple query and execute it:
ctx.execute( """ select `Customer Name`, Brand, Category from df limit 5 """ )
The preceding code will return the following output:
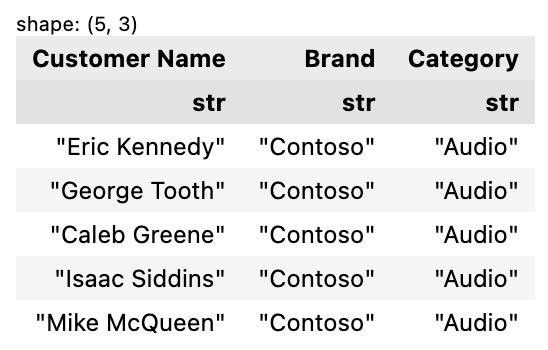
Figure 4.38 – The result of a SQL query
- Apply a group by aggregation, showing the top five average quantities by brand:
ctx.execute( """ select Brand, avg(Quantity) as `Avg Quantity` from df group by Brand order by `Avg Quantity` desc limit 5 """ )
The preceding code will return the following output:
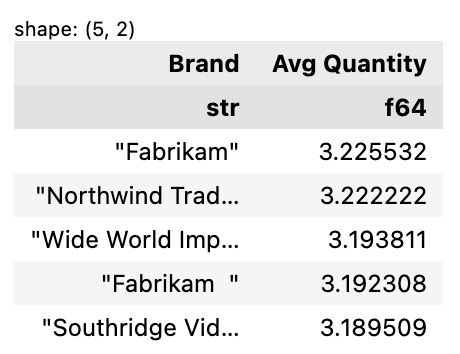
Figure 4.39 – Top five average quantities by brand
- Run a SQL query in one go, showing the first five rows for a few selected columns using a LazyFrame:
pl.SQLContext(lf=df.lazy()).execute( """ select Brand, Category from lf limit 5 """ ).collect()
The preceding code will return the following output:
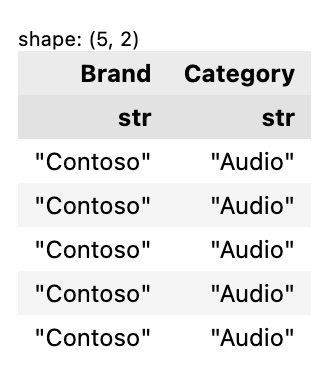
Figure 4.40 – The first five rows with the Brand and Category columns selected
How it works…
By default, your SQL query returns a LazyFrame as its output. You can specify to return a DataFrame by specifying a parameter either when you define your SQL context or execute your query.
Also, know that when your column name contains a space, you need to use a special character, `
(a backtick or grave accent).
One thing to keep in mind when using SQL in Polars is that, as of this writing, not all data operations are available such as rank and row number. I suggest you read Polars’ documentation to learn more about what Polars SQL can and cannot do: https://pola-rs.github.io/polars/user-guide/sql/intro/.
Tip
You can use a library such as DuckDB to run SQL queries on top of Polars’ DataFrames. It basically overcomes the limitation of available SQL transformations in Polars. We’ll cover how to use other Python libraries in combination with Polars in Chapter 10, Interoperability with Other Python Libraries.
See also
Please refer to these resources to learn more about using SQL in Polars: