Overview of spaCy
Before getting started with the spaCy code, we will first have an overview of NLP applications in real life, NLP with Python, and NLP with spaCy. In this section, we'll find out the reasons to use Python and spaCy for developing NLP applications. We will first see how Python goes hand-in-hand with text processing, then we'll understand spaCy's place in the Python NLP libraries. Let's start our tour with the close-knit relationship between Python and NLP.
Rise of NLP
Over the past few years, most of the branches of AI created a lot of buzz, including NLP, computer vision, and predictive analytics, among others. But just what is NLP? How can a machine or code solve human language?
NLP is a subfield of AI that analyzes text, speech, and other forms of human-generated language data. Human language is complicated – even a short paragraph contains references to the previous words, pointers to real-world objects, cultural references, and the writer's or speaker's personal experiences. Figure 1.1 shows such an example sentence, which includes a reference to a relative date (recently), phrases that can be resolved only by another person who knows the speaker (regarding the city that the speaker's parents live in) and who has general knowledge about the world (a city is a place where human beings live together):
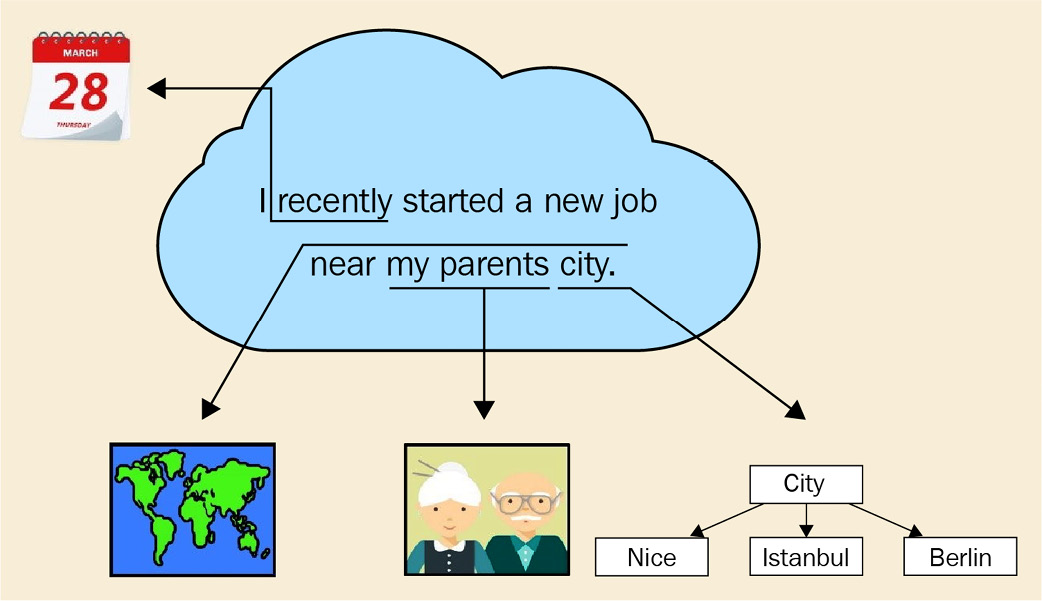
Figure 1.1 – An example of human language, containing many cognitive and cultural aspects
How do we process such a complicated structure then? We have our weapons too; we model natural language with statistical models, and we process linguistic features to turn the text into a well-structured representation. This book provides all the necessary background and tools for you to extract the meaning out of text. By the end of this book, you will possess statistical and linguistic knowledge to process text by using a great tool – the spaCy library.
Though NLP gained popularity recently, processing human language has been present in our lives via many real-world applications, including search engines, translation services, and recommendation engines.
Search engines such as Google Search, Yahoo Search, and Microsoft Bing are an integral part of our daily lives. We look for homework help, cooking recipes, information about celebrities, the latest episodes of our favorite TV series; all sorts of information that we use in our daily lives. There is even a verb in English (also in many other languages), to google, meaning to look up some information on the Google search engine.
Search engines use advanced NLP techniques including mapping queries into a semantic space, where similar queries are represented by similar vectors. A quick trick is called autocomplete, where query suggestions appear on the search bar when we type the first few letters. Autocomplete looks tricky but indeed the algorithm is a combination of a search tree walk and character-level distance calculation. A past query is represented by a sequence of its characters, where each character corresponds to a node in the search tree. The arcs between the characters are assigned weights according to the popularity of this past query.
Then, when a new query comes, we compare the current query string to past queries by walking on the tree. A fundamental Computer Science (CS) data structure, the tree, is used to represent a list of queries, who would have thought that? Figure 1.2 shows a walk on the character tree:
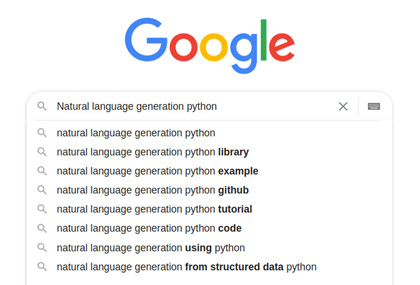
Figure 1.2 – An autocomplete example
This is a simplified explanation; the real algorithms blend several techniques usually. If you want to learn more about this subject, you can read the great articles about the data structures: http://blog.notdot.net/2010/07/Damn-Cool-Algorithms-Levenshtein-Automata and http://blog.notdot.net/2007/4/Damn-Cool-Algorithms-Part-1-BK-Trees.
Continuing with search engines, search engines also know how to transform unstructured data to structured and linked data. When we type Diana Spencer
into the search bar, this is what comes up:
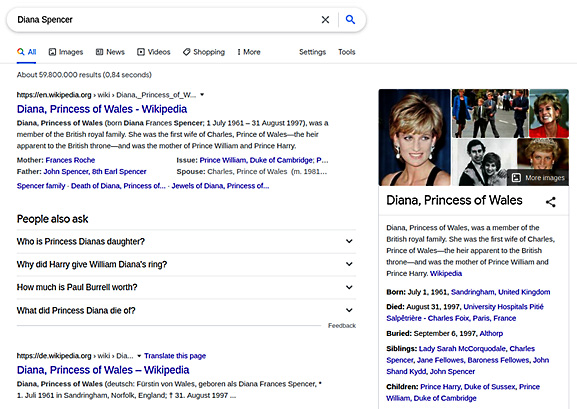
Figure 1.3 – Search results for the query "Diana Spencer"
How did the search engine link Diana Spencer
to her well-known name Princess Diana? This is called entity linking. We link entities that mention the same real-world entity. Entity-linking algorithms concern representing semantic relations and knowledge in general. This area of NLP is called the Semantic Web. You can learn more about this at https://www.cambridgesemantics.com/blog/semantic-university/intro-semantic-web/. I worked as a knowledge engineer at a search engine company at the beginning of my career and really enjoyed it. This is a fascinating subject in NLP.
There is really no limit to what you can develop: search engine algorithms, chatbots, speech recognition applications, and user sentiment recognition applications. NLP problems are challenging yet fascinating. This book's mission is to provide you a toolbox with all the necessary tools. The first step of NLP development is choosing the programming language we will use wisely. In the next section, we will explain why Python is the weapon of choice. Let's move on to the next section to see the string bond of NLP and Python.
NLP with Python
As we remarked before, NLP is a subfield of AI that analyzes text, speech, and other forms of human-generated language data. As an industry professional, my first choice for manipulating text data is Python. In general, there are many benefits to using Python:
- It is easy to read and looks very similar to pseudocode.
- It is easy to produce and test code with.
- It has a high level of abstraction.
Python is a great choice for developing NLP systems because of the following:
- Simplicity: Python is easy to learn. You can focus on NLP rather than the programming language details.
- Efficiency: It allows for easier development of quick NLP application prototypes.
- Popularity: Python is one of the most popular languages. It has huge community support, and installing new libraries with pip is effortless.
- AI ecosystem presence: A significant number of open source NLP libraries are available in Python. Many machine learning (ML) libraries such as PyTorch, TensorFlow, and Apache Spark also provide Python APIs.
- Text methods: String and file operations with Python are effortless and straightforward. For example, splitting a sentence at the whitespaces requires only a one-liner,
sentenc.split()
, which can be quite painful in other languages, such as C++, where you have to deal with stream objects for this task.
When we put all the preceding points together, the following image appears – Python intersects with string processing, the AI ecosystem, and ML libraries to provide us the best NLP development experience:
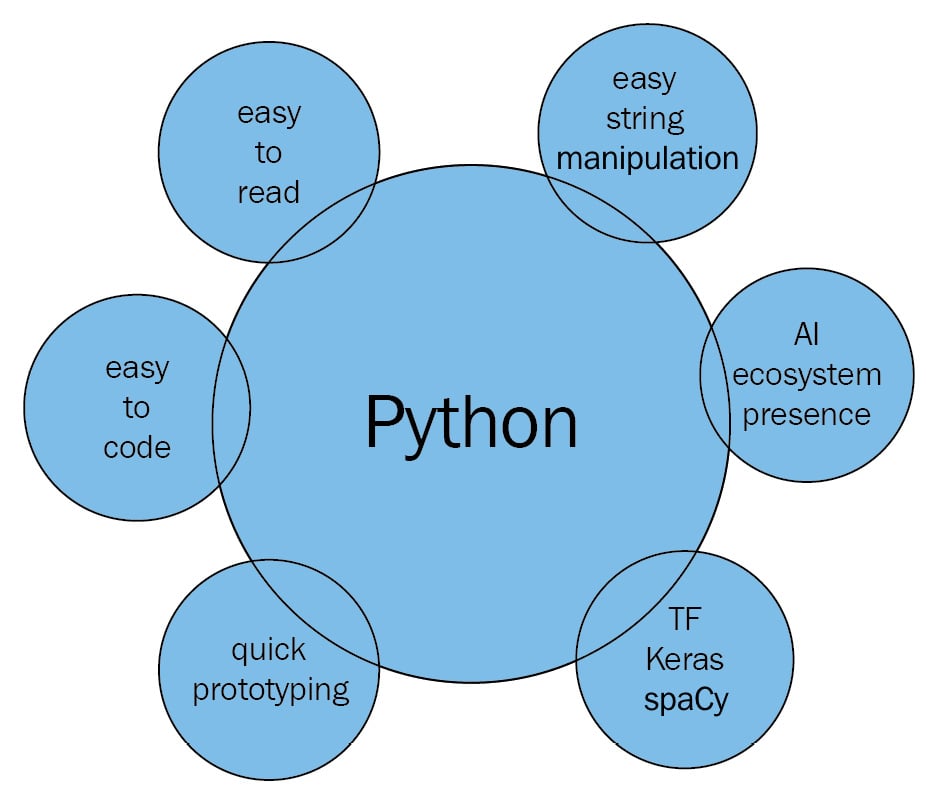
Figure 1.4 – NLP with Python overview
We will use Python 3.5+ throughout this book. Users who do not already have Python installed can follow the instructions at https://realpython.com/installing-python/. We recommend downloading and using the latest version of Python 3.
In Python 3.x, the default encoding is Unicode, which means that we can use Unicode text without worrying much about the encoding. We won't go into details of encodings here, but you can think of Unicode as an extended set of ASCII, including more characters such as German-alphabet umlauts and the accented characters of the French alphabet. This way we can process German, French, and many more languages other than English.
Reviewing some useful string operations
In Python, the text is represented by strings, objects of the str
class. Strings are immutable sequences of characters. Creating a string object is easy – we enclose the text in quotation marks:
word = 'Hello World'
Now the word
variable contains the string Hello World
. As we mentioned, strings are sequences of characters, so we can ask for the first item of the sequence:
print (word [0]) H
Always remember to use parentheses with print
, since we are coding in Python 3.x. We can similarly access other indices, as long as the index doesn't go out of bounds:
word [4] 'o'
How about string length? We can use the len
method, just like with list
and other sequence types:
len(word) 11
We can also iterate over the characters of a string with sequence methods:
for ch in word: print(ch) H e l l o W o r l d
Pro tip
Please mind the indentation throughout the book. Indentation in Python is the way we determine the control blocks and function definitions in general, and we will apply this convention in this book.
Now let's go over the more string methods such as counting characters, finding a substring, and changing letter case.
count
counts the number of occurrences of a character in the string, so the output is 3
here:
word.count('l') 3
Often, you need to find the index of a character for a number of substring operations such as cutting and slicing the string:
word.index(e) 1
Similarly, we can search for substrings in a string with the find
method:
word.find('World') 6
find
returns –1
if the substring is not in the string:
word.find('Bonjour') -1
Searching for the last occurrence of a substring is also easy:
word.rfind('l') 9
We can change letter case by the upper
and lower
methods:
word.upper() 'HELLO WORLD'
The upper
method changes all characters to uppercase. Similarly, the lower
method changes all characters to lowercase:
word.lower() 'hello world'
The capitalize
method capitalizes the first character of the string:
'hello madam'.capitalize() 'Hello madam'
The title
method makes the string title case. Title case literally means to make a title, so each word of the string is capitalized:
'hello madam'.title() 'Hello Madam'
Forming new strings from other strings can be done in several ways. We can concatenate two strings by adding them:
'Hello Madam!' + 'Have a nice day.' 'Hello Madam!Have a nice day.'
We can also multiply a string with an integer. The output will be the string concatenated to itself by the number of times specified by the integer:
'sweet ' * 5 'sweet sweet sweet sweet '
join
is a frequently used method; it takes a list of strings and joins them into one string:
' '.join (['hello', 'madam']) 'hello madam'
There is a variety of substring methods. Replacing a substring means changing all of its occurrences with another string:
'hello madam'.replace('hello', 'good morning') 'good morning madam'
Getting a substring by index is called slicing. You can slice a string by specifying the start index and end index. If we want only the second word, we can do the following:
word = 'Hello Madam Flower' word [6:11] 'Madam'
Getting the first word is similar. Leaving the first index blank means the index starts from zero:
word [:5] 'Hello'
Leaving the second index blank has a special meaning as well – it means the rest of the string:
word [12:] 'Flower'
We now know some of the Pythonic NLP operations. Now we can dive into more of spaCy.
Getting a high-level overview of the spaCy library
spaCy is an open source Python library for modern NLP. The creators of spaCy describe their work as industrial-strength NLP, and as a contributor I can assure you it is true. spaCy is shipped with pretrained language models and word vectors for 60+ languages.
spaCy is focused on production and shipping code, unlike its more academic predecessors. The most famous and frequently used Python predecessor is NLTK. NLTK's main focus was providing students and researchers an idea of language processing. It never put any claims on efficiency, model accuracy, or being an industrial-strength library. spaCy focused on providing production-ready code from the first day. You can expect models to perform on real-world data, the code to be efficient, and the ability to process a huge amount of text data in a reasonable time. The following table is an efficiency comparison from the spaCy documentation (https://spacy.io/usage/facts-figures#speed-comparison):
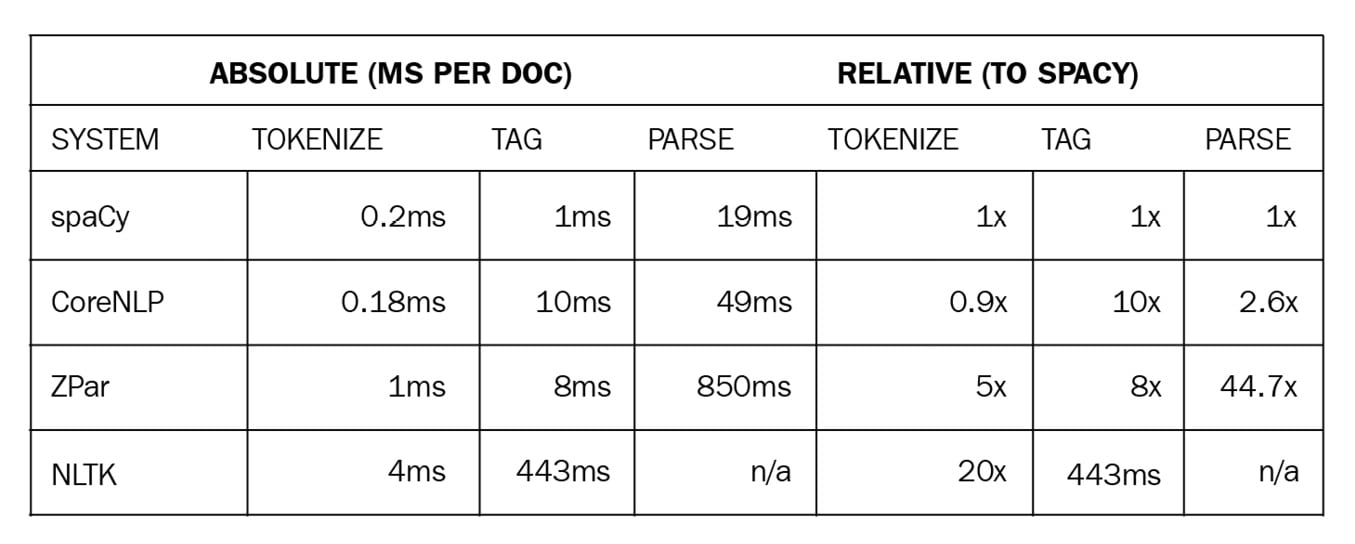
Figure 1.5 – A speed comparison of spaCy and other popular NLP frameworks
The spaCy code is also maintained in a professional way, with issues sorted by labels and new releases covering as many fixes as possible. You can always raise an issue on the spaCy GitHub repo at https://github.com/explosion/spaCy, report a bug, or ask for help from the community.
Another predecessor is CoreNLP (also known as StanfordNLP). CoreNLP is implemented in Java. Though CoreNLP competes in terms of efficiency, Python won by easy prototyping and spaCy is much more professional as a software package. The code is well maintained, issues are tracked on GitHub, and every issue is marked with some labels (such as bug, feature, new project). Also, the installation of the library code and the models is easy. Together with providing backward compatibility, this makes spaCy a professional software project. Here is a detailed comparison from the spaCy documentation at https://spacy.io/usage/facts-figures#comparison:
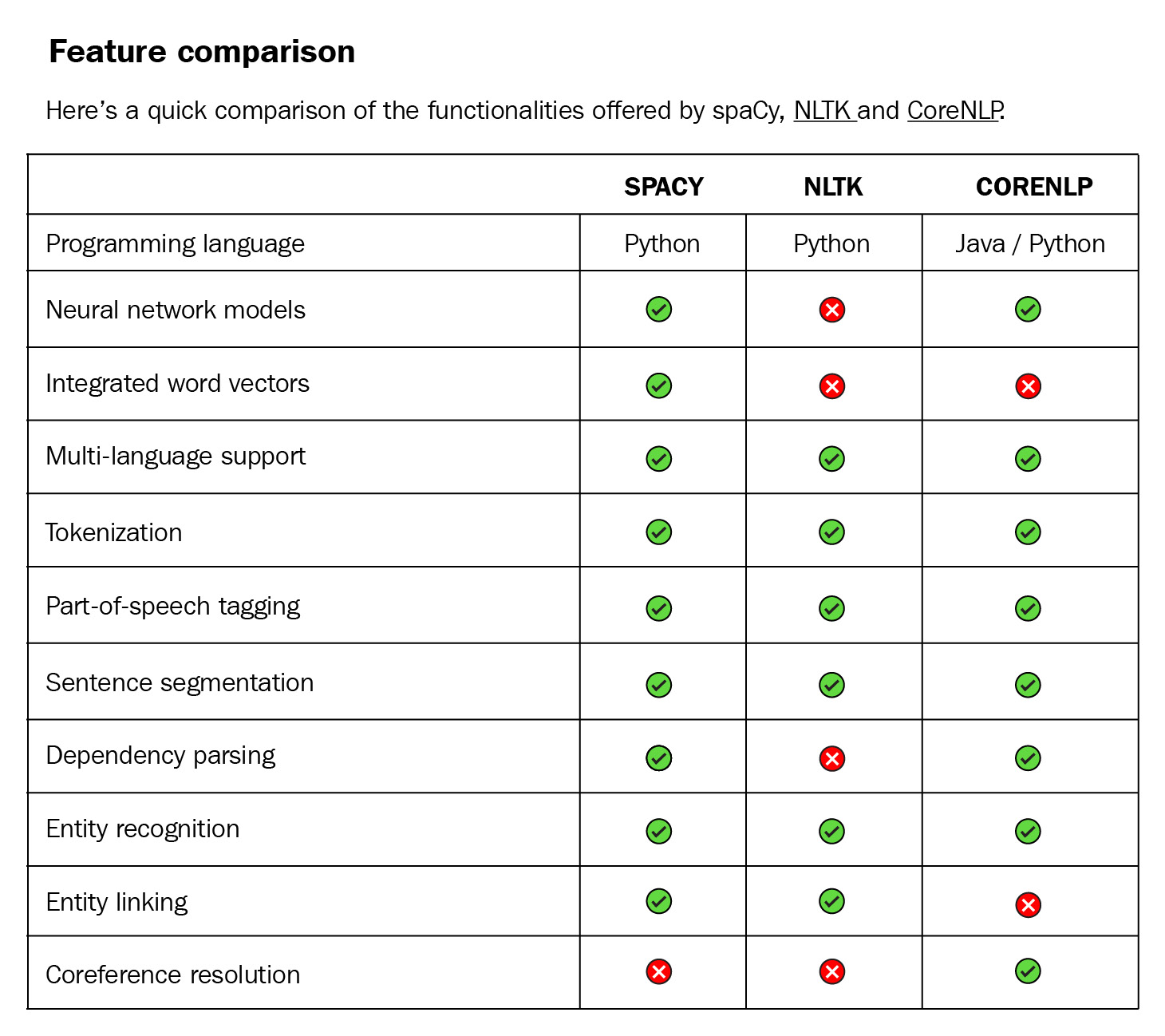
Figure 1.6 – A feature comparison of spaCy, NLTK, and CoreNLP
Throughout this book, we will be using spaCy's latest release v3.1 (the version used at the time of writing this book) for all our computational linguistics and ML purposes. The following are the features in the latest release:
- Original data preserving tokenization.
- Statistical sentence segmentation.
- Named entity recognition.
- Part-of-Speech (POS) tagging.
- Dependency parsing.
- Pretrained word vectors.
- Easy integration with popular deep learning libraries. spaCy's ML library
Thinc
provides thin wrappers around PyTorch, TensorFlow, and MXNet. spaCy also provides wrappers forHuggingFace
Transformers byspacy-transformers
library. We'll see more of theTransformers
in Chapter 9, spaCy and Transformers. - Industrial-level speed.
- A built-in visualizer, displaCy.
- Support for 60+ languages.
- 46 state-of-the-art statistical models for 16 languages.
- Space-efficient string data structures.
- Efficient serialization.
- Easy model packaging and usage.
- Large community support.
We had a quick glance around spaCy as an NLP library and as a software package. We will see what spaCy offers in detail throughout the book.
Tips for the reader
This book is a practical guide. In order to get the most out of the book, I recommend readers replicate the code in their own Python shell. Without following and performing the code, it is not possible to get a proper understanding of NLP concepts and spaCy methods, which is why we have arranged the upcoming chapters in the following way:
- Explanation of the language/ML concept
- Application code with spaCy
- Evaluation of the outcome
- Challenges of the methodology
- Pro tips and tricks to overcome the challenges