Now that we have seen how feature engineering techniques help in building predictive models, let's try and improve the performance of these models and evaluate whether the newly built model works better than the previous built model. Then, we will talk about two very important concepts that you must always keep in mind when doing predictive analytics, and these are the reducible and irreducible errors in your predictive models.
Let's first import the necessary modules, as shown in the following screenshot:
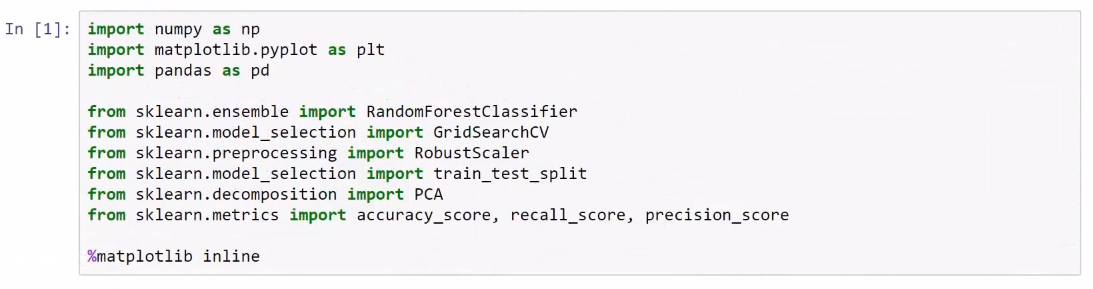
So, let's go to the Jupyter Notebook and take a look at the imported credit card default dataset that we saw earlier in this chapter, but as you can see, some modifications have been made to this dataset:
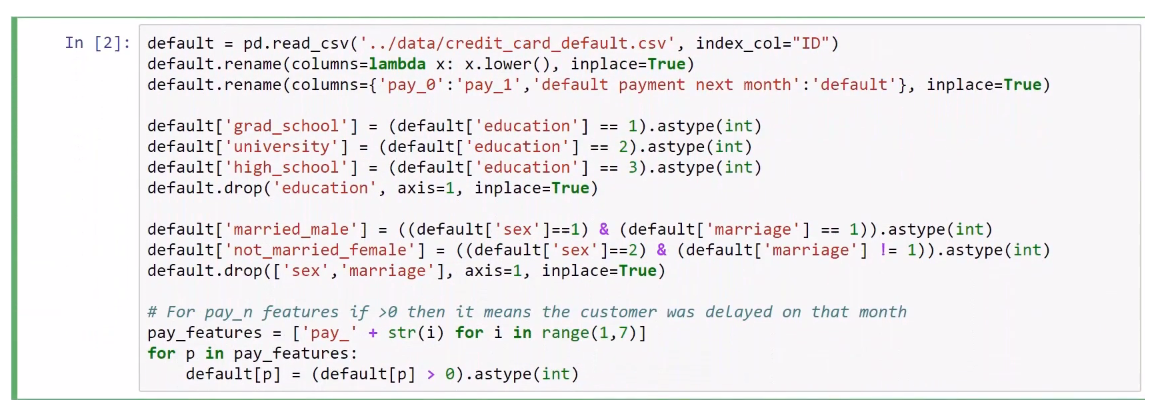
For this model, instead of transforming the sex and marriage features into two dummy features, the ones that we have...