Robust regression with random sample consensus
A common problem with linear regressions is caused by the presence of outliers. An ordinary least square approach will take them into account and the result (in terms of coefficients) will be therefore biased. In the following figure, there's an example of such a behavior:
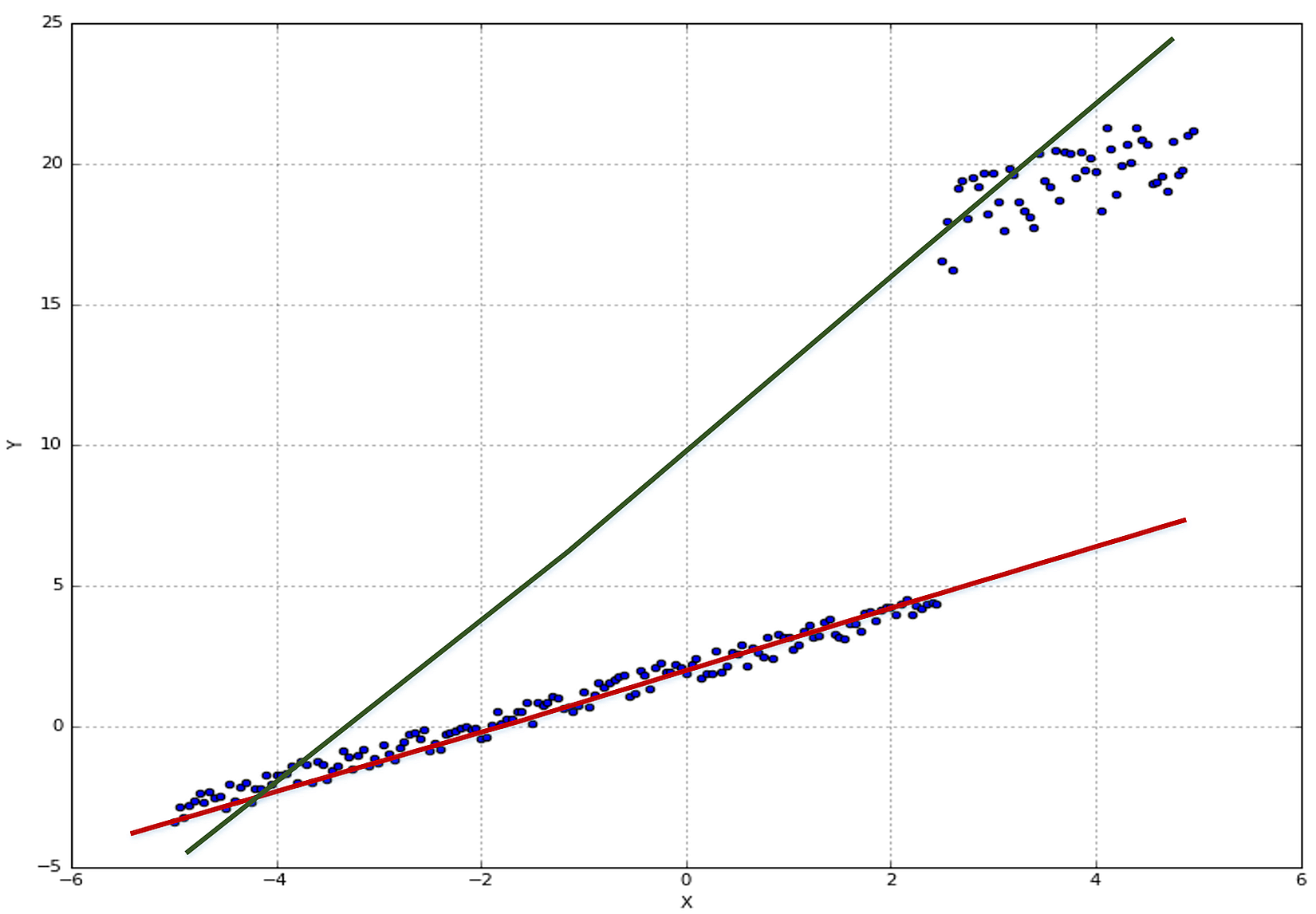
The less sloped line represents an acceptable regression which discards the outliers, while the other one is influenced by them. An interesting approach to avoid this problem is offered by random sample consensus (RANSAC), which works with every regressor by subsequent iterations, after splitting the dataset into inliers and outliers. The model is trained only with valid samples (evaluated internally or through the callable is_data_valid()
) and all samples are re-evaluated to verify if they're still inliers or they have become outliers. The process ends after a fixed number of iterations or when the desired score is achieved.
In the following snippet, there's an example of simple...