Understanding the concept of overfitting
Overfitting occurs when the trained ML model learns too much from the given training data. In this situation, the trained model successfully gets a high evaluation score on the training data but a far lower score on new, unseen data. In other words, the trained ML model fails to generalize the knowledge learned from the training data to the unseen data.
So, how exactly does the trained ML model get decent performance on the training data but fail to give a reasonable performance on unseen data? Well, that happens when the model tries too hard to achieve high performance on the training data and has picked up knowledge that is only applicable to that specific training data. Of course, this will negatively impact the model's ability to generalize, which results in bad performance when the model is evaluated on unseen data.
To detect whether our trained ML model faces an overfitting issue, we can monitor the performance of our model on the training data versus unseen data. Performance can be defined as the loss value of our model or metrics that we care about, for example, accuracy, precision, and the mean absolute error. If the performance of the training data keeps getting better, while the performance on the unseen data starts to become stagnant or even gets worse, then this is a sign of an overfitting issue (see Figure 1.1):
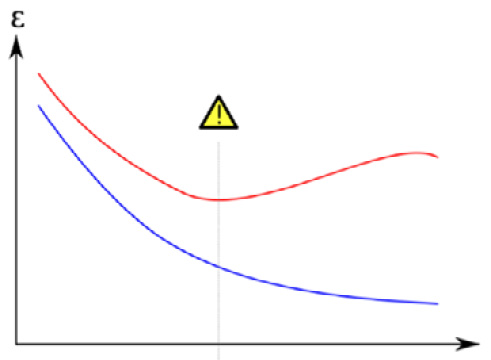
Figure 1.1 – The model's performance on training data versus unseen data (overfitting)
Note
The preceding diagram image has been reproduced according to the license specified: https://commons.wikimedia.org/wiki/File:Overfitting_svg.svg.
Now that you are aware of the overfitting problem, we need to learn how to prevent this from happening in our ML development life cycle. We will discuss this in the following sections.