Improving efficiency of sequence-to-sequence network
A first interesting point to notice in the chatbot example is the reverse ordered input sequence: such a technique has been shown to improve results.
For translation, it is very common then to use a bidirectional LSTM to compute the internal state as seen in Chapter 5, Analyzing Sentiment with a Bidirectional LSTM: two LSTMs, one running in the forward order, the other in the reverse order, run in parallel on the sequence, and their outputs are concatenated:
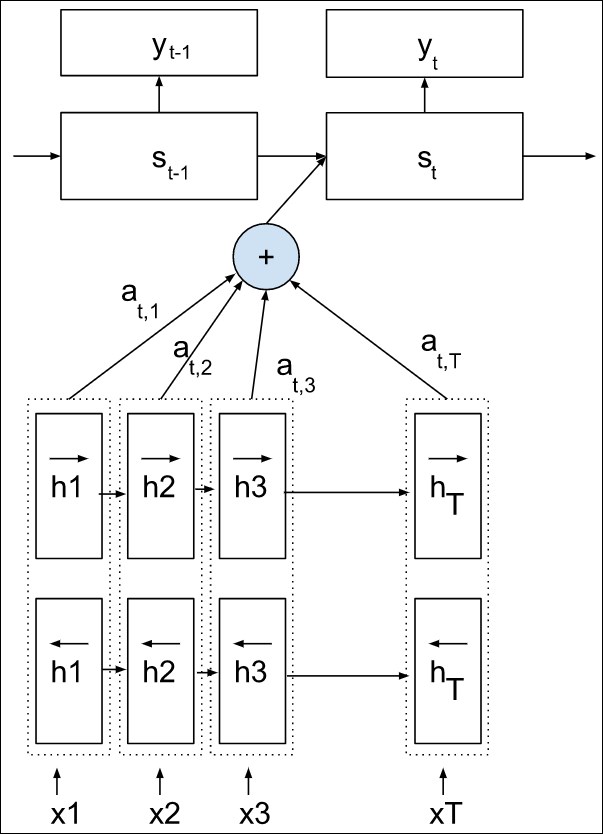
Such a mechanism captures better information given future and past.
Another technique is the attention mechanism that will be the focus of the next chapter.
Lastly, refinement techniques have been developed and tested with two-dimensional Grid LSTM, which are not very far from stacked LSTM (the only difference is a gating mechanism in the depth/stack direction):
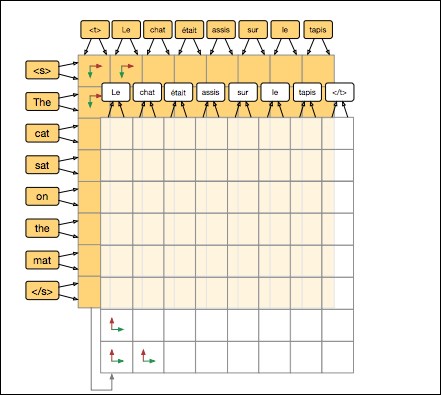
Grid long short-term memory
The principle of refinement is to run the stack in both orders on the input sentence as well, sequentially...