In this chapter, we introduced recurrent neural networks and demonstrated how to use an RNN on the MNIST dataset. RNNs are particularly useful for working with time series data, since they are essentially feedforward networks that are unrolled over time. This makes them very suitable for tasks such as handwriting and speech recognition, as they operate on sequences of data. We also looked at a more powerful variant of the RNN, the LSTM. The LSTM uses four gates to decide what information to pass on to the next time step, enabling it to uncover long-term dependencies in data. Finally, in this chapter we built a simple language model, enabling us to generate text from sample input text. We used a model based on the GRU. The GRU is a slightly simplified version of the LSTM, containing three gates and combining the input and forget gates of the LSTM. This model used probability...




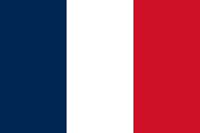


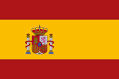




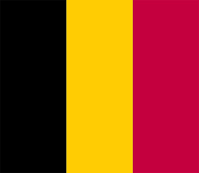
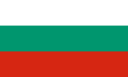
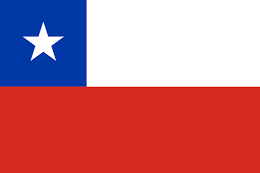
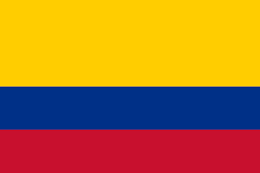
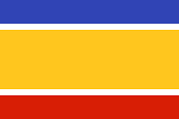

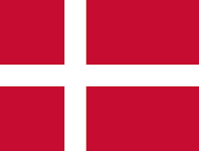



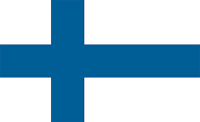

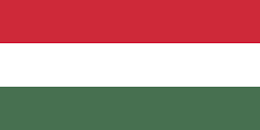






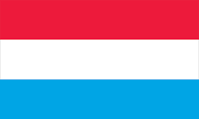
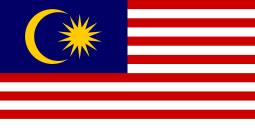

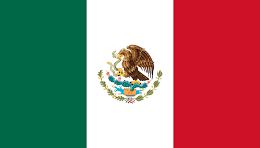

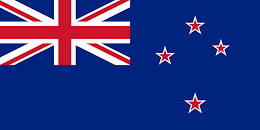







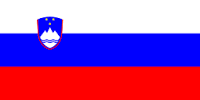




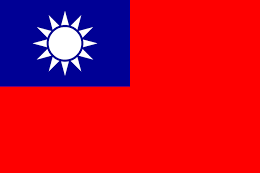


