Software and environment setup
Python is one of the most popular programming languages for data science and machine learning thanks to the large open source community that has driven the development of these libraries. Python’s ease of use and flexible nature made it a prime candidate in the data science world, where experimentation and iteration are key features of the development cycle. While there are new languages in development for data science applications, such as Julia, Python currently remains the key language for data science due to its wide breadth of open source projects, supporting applications from statistical modeling to deep learning. We have chosen to use Python in this book due to its positioning as an important language for data science and its demand in the job market.
Python is available for all major operating systems: Microsoft Windows, macOS, and Linux. Additionally, the installer and documentation can be found at the official website: https://www.python.org/.
This book is written for Python version 3.8 (or higher). It is recommended that you use whatever recent version of Python that is available. It is not likely that the code found in this book will be compatible with Python 2.7, and most active libraries have already started dropping support for Python 2.7 since official support ended in 2020.
The libraries used in this book can be installed with the Python package manager, pip
, which is part of the standard Python library in contemporary versions of Python. More information about pip
can be found here: https://docs.python.org/3/installing/index.html. After pip
is installed, packages can be installed using pip
on the command line. Here is basic usage at a glance:
Install a new package using the latest version:
pip install SomePackage
Install the package with a specific version, version 2.1
in this example:
pip install SomePackage==2.1
A package that is already installed can be upgraded with the --
upgrade
flag:
pip install SomePackage –upgrade
In general, it is recommended to use Python virtual environments between projects and to keep project dependencies separate from system directories. Python provides a virtual environment utility, venv
, which, like pip
, is part of the standard library in contemporary versions of Python. Virtual environments allow you to create individual binaries of Python, where each binary of Python has its own set of installed dependencies. Using virtual environments can prevent package version issues and conflict when working on multiple Python projects. Details on setting up and using virtual environments can be found here: https://docs.python.org/3/library/venv.html.
While we recommend the use of Python and Python’s virtual environments for environment setups, a highly recommended alternative is Anaconda. Anaconda is a free (enterprise-ready) analytics-focused distribution of Python by Anaconda Inc. (previously Continuum Analytics). Anaconda distributions come with many of the core data science packages, common IDEs (such as Jupyter and Visual Studio Code), and a graphical user interface for managing environments. Anaconda can be installed using the installer found at the Anaconda website here: https://www.anaconda.com/products/distribution.
Anaconda comes with its own package manager, conda
, which can be used to install new packages similarly to pip
.
Install a new package using the latest version:
conda install SomePackage
Upgrade a package that is already installed:
conda upgrade SomePackage
Throughout this book, we will make use of several core libraries in the Python data science ecosystem, such as NumPy
for array manipulations, pandas
for higher-level data manipulations, and matplotlib
for data visualization. The package versions used for this book are contained in the following list. Please ensure that the versions installed in your environment are equal to or greater than the versions listed. This will help ensure that the code examples run correctly:
statsmodels 0.13.2
Matplotlib 3.5.2
NumPy 1.23.0
SciPy 1.8.1
scikit-learn 1.1.1
pandas 1.4.3
The packages used for the code in this book are shown here in Figure 1.1. The __version__
method can be used to print the package version in code.
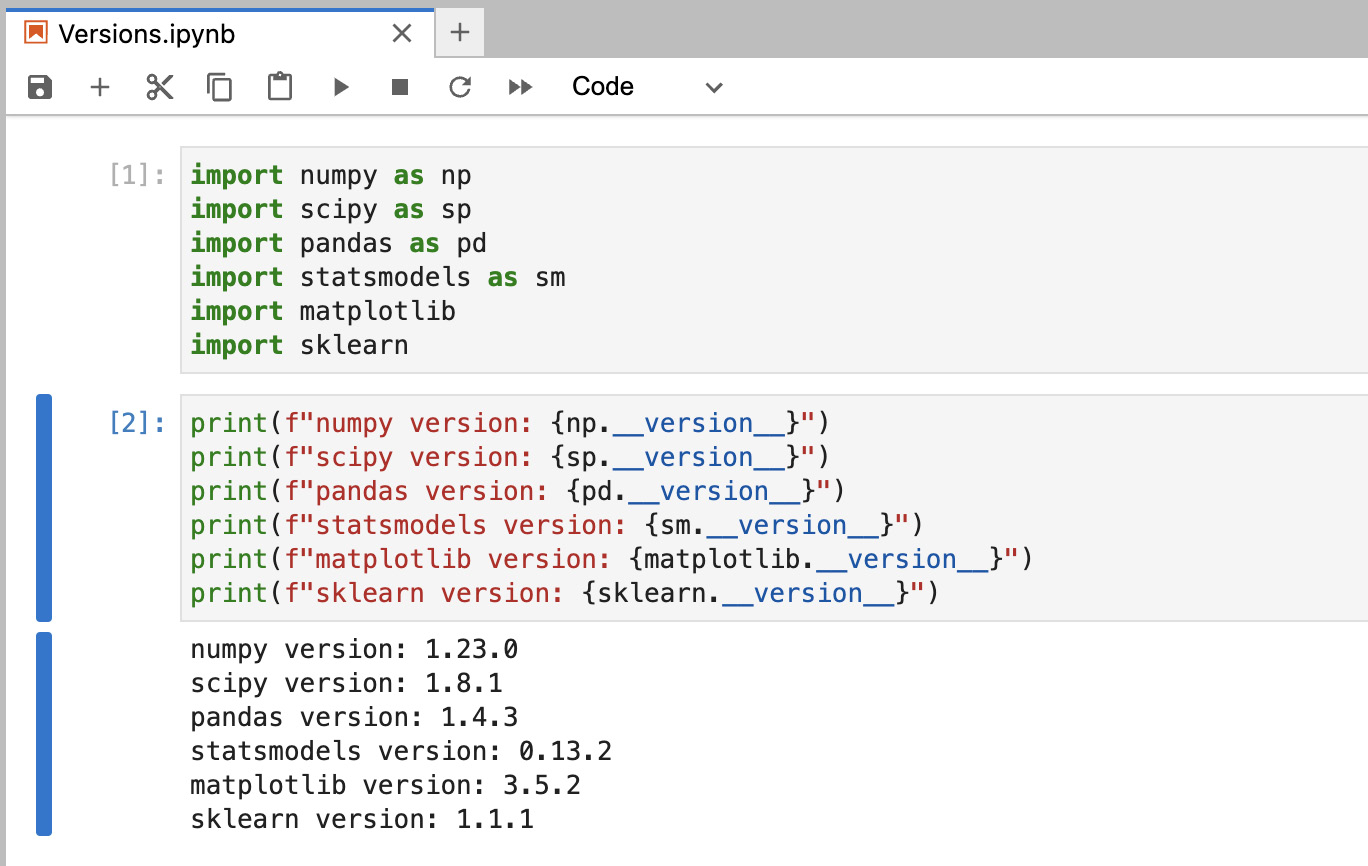
Figure 1.1 – Package versions used in this book
Having set up the technical environment for the book, let’s get into the statistics. In the next sections, we will discuss the concepts of population and sampling. We will demonstrate sampling strategies with code implementations.