RAG versus fine-tuning
RAG is not always an alternative to fine-tuning, and fine-tuning cannot always replace RAG. If we accumulate too much data in RAG datasets, the system may become too cumbersome to manage. On the other hand, we cannot fine-tune a model with dynamic, ever-changing data such as daily weather forecasts, stock market values, corporate news, and all forms of daily events.
The decision of whether to implement RAG or fine-tune a model relies on the proportion of parametric versus non-parametric information. The fundamental difference between a model trained from scratch or fine-tuned and RAG can be summed up in terms of parametric and non-parametric knowledge:
- Parametric: In a RAG-driven generative AI ecosystem, the parametric part refers to the generative AI model’s parameters (weights) learned through training data. This means the model’s knowledge is stored in these learned weights and biases. The original training data is transformed into a mathematical form, which we call a parametric representation. Essentially, the model “remembers” what it learned from the data, but the data itself is not stored explicitly.
- Non-Parametric: In contrast, the non-parametric part of a RAG ecosystem involves storing explicit data that can be accessed directly. This means that the data remains available and can be queried whenever needed. Unlike parametric models, where knowledge is embedded indirectly in the weights, non-parametric data in RAG allows us to see and use the actual data for each output.
The difference between RAG and fine-tuning relies on the amount of static (parametric) and dynamic (non-parametric) ever-evolving data the generative AI model must process. A system that relies too heavily on RAG might become overloaded and cumbersome to manage. A system that relies too much on fine-tuning a generative model will display its inability to adapt to daily information updates.
There is a decision-making threshold illustrated in Figure 1.2 that shows that a RAG-driven generative AI project manager will have to evaluate the potential of the ecosystem’s trained parametric generative AI model before implementing a non-parametric (explicit data) RAG framework. The potential of the RAG component requires careful evaluation as well.
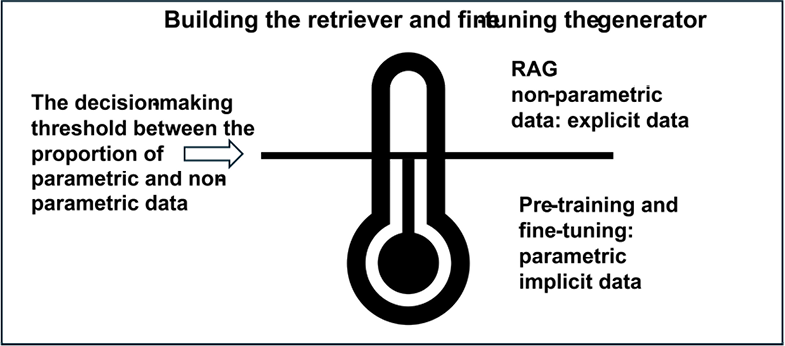
Figure 1.2: The decision-making threshold between enhancing RAG or fine-tuning an LLM
In the end, the balance between enhancing the retriever and the generator in a RAG-driven generative AI ecosystem depends on a project’s specific requirements and goals. RAG and fine-tuning are not mutually exclusive.
RAG can be used to improve a model’s overall efficiency, together with fine-tuning, which serves as a method to enhance the performance of both the retrieval and generation components within the RAG framework. We will fine-tune a proportion of the retrieval data in Chapter 9, Empowering AI Models: Fine-Tuning RAG Data and Human Feedback.
We will now see how a RAG-driven generative AI involves an ecosystem with many components.