Error modeling is another form of meta-level modeling but in this case we will be modeling cases where there were errors in our predictions. In this way, we can increase the accuracy of that prediction. Using an example, we will walk through how to do error modeling.
Consider the following scenario, for example:
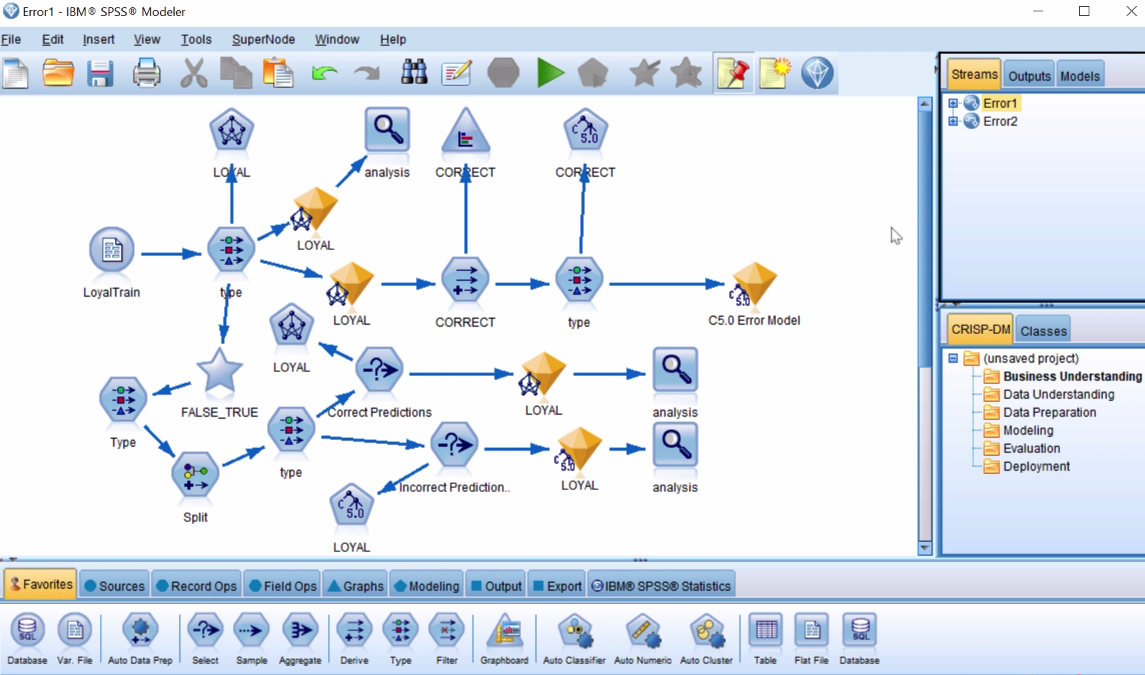
Here, we have a dataset named LoyalTrain. This is just a training dataset; we have our testing and validation dataset at a different place and will build a model only on the training dataset. Theer is also a Type node and a Neural Net model, where we are predicting the variable loyal. Run the Analysis node to see the results as shown in the following screenshot:
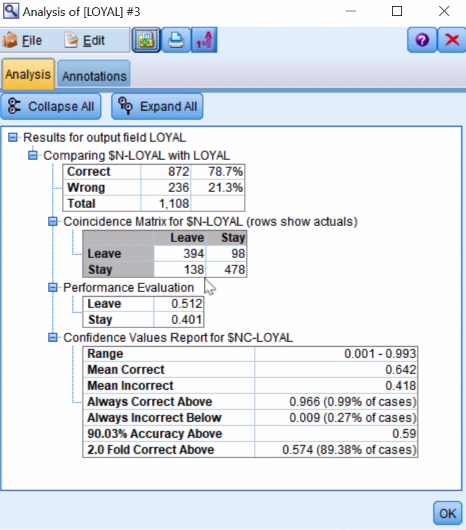
You can see that there are two categories in the outcome variable: people are either predicted to stay or to leave. You can also see that correct predictions were made in 79% of the cases. Mistakes...