Since we know that the maximum number of unique words in our entire corpus is 12,000, we can assume that the longest possible review can only be 12,000 in length. Hence, we can make each review a vector of length 12,000, containing binary values. How does this work? Suppose we have a review of two words: bad and movie. A list containing these words in our dataset may look like [6, 49]. Instead, we can represent this same review as a 12,000-dimensional vector populated with 0s, except for the indices of 6 and 49, which would instead be 1s. What you're essentially doing is creating 12,000 dummy features to represent each review. Each of these dummy features represents the presence or absence of any of the 12,000 words in a given review. This approach is also known as one-hot encoding. It is commonly used to encode features and categorical labels alike in various...




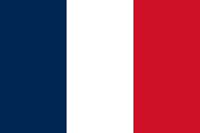


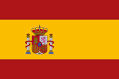



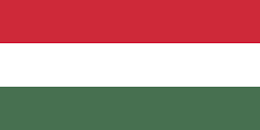

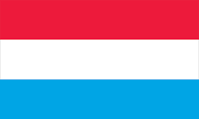





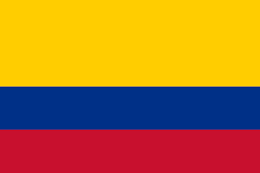
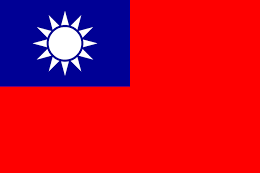
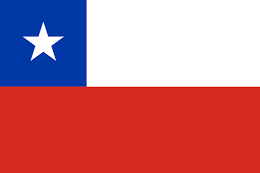



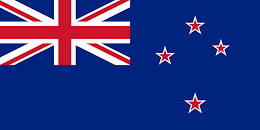
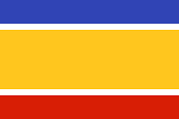
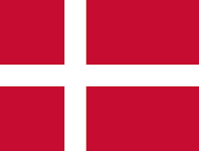
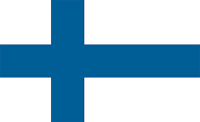







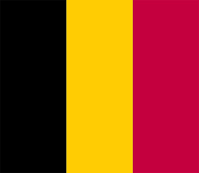

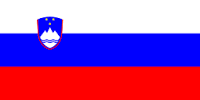





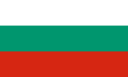


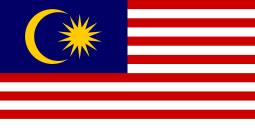



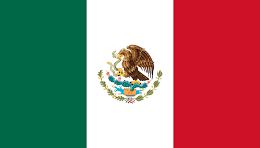
