Since we have a two-dimensional latent space, we can simply plot out the representations as a 2D manifold where encoded instances of each digit class may be visualized with respect to their proximity to other instances. This allows us to inspect the continuous latent space that we spoke of before and see how the network relates to different features in the 10-digit classes (0 to 9) to each other. To do this, we revisit the encoding module from our VAE, which can now be used to produce a compressed latent space from some given data. Thus, we use the encoder module to make predictions on the test set, thereby encoding these images the latent space. Finally, we can use a scatterplot from Matplotlib to plot out the latent representation. Do note that each individual point represents an encoded instance from the test set. The colors denote the different...




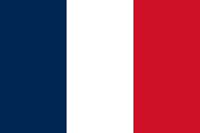


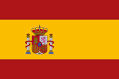



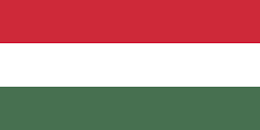

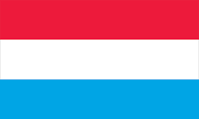





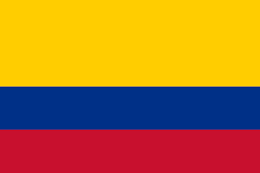
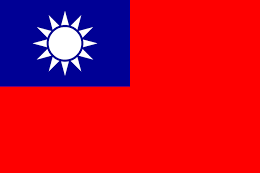
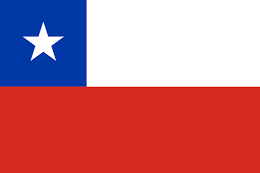



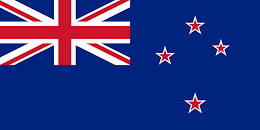
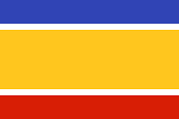
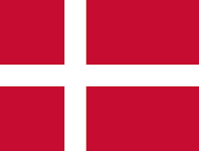
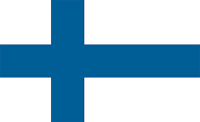







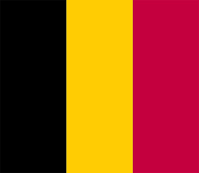

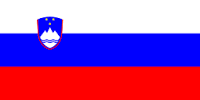





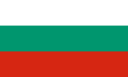


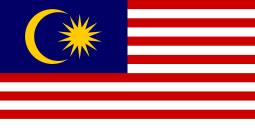



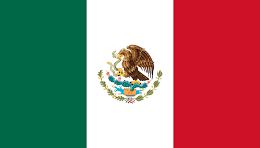
