Machine learning (ML) is a sub-field of AI and has become popular in the last 10 years and, at times, the two are used interchangeably. AI has a lot of other sub-fields aside from machine learning. ML systems are built by showing lots of examples, unlike symbolic AI, where we hard code rules to build the system. At a high level, machine learning systems look at tons of data and come up with rules to predict outcomes for unseen data:
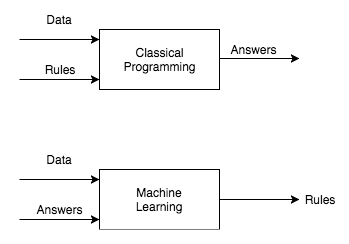
Most ML algorithms perform well on structured data, such as sales predictions, recommendation systems, and marketing personalization. An important factor for any ML algorithm is feature engineering and data scientists need to spend a lot of time to get the features right for ML algorithms to perform. In certain domains, such as computer vision and natural language processing (NLP), feature engineering is challenging as they suffer from high dimensionality.
Until recently, problems like this were challenging for organizations to solve using typical machine-learning techniques, such as linear regression, random forest, and so on, for reasons such as feature engineering and high dimensionality. Consider an image of size 224 x 224 x 3 (height x width x channels), where 3 in the image size represents values of red, green, and blue color channels in a color image. To store this image in computer memory, our matrix will contain 150,528 dimensions for a single image. Assume you want to build a classifier on top of 1,000 images of size 224 x 224 x 3, the dimensions will become 1,000 times 150,528. A special branch of machine learning called deep learning allows you to handle these problems using modern techniques and hardware.