Review of Modeling Results
In order to develop a binary classification model to meet the business requirements of our client, we have now tried several modeling approaches to varying degrees of success. In the end, we will pick the one that worked the best, to perform additional analyses on and present to our client. However, it is also good to present the client with findings from the various options that were explored. This shows that a thorough job was done.
Here, we review the different models that we tried for the case study problem, the hyperparameters that we needed to tune, and results from cross-validation. We only include the work we did using all possible features, not the earlier models where we used only one or two features (e.g. EDUCATION) as a way to learn how to use model-fitting functions in scikit-learn.
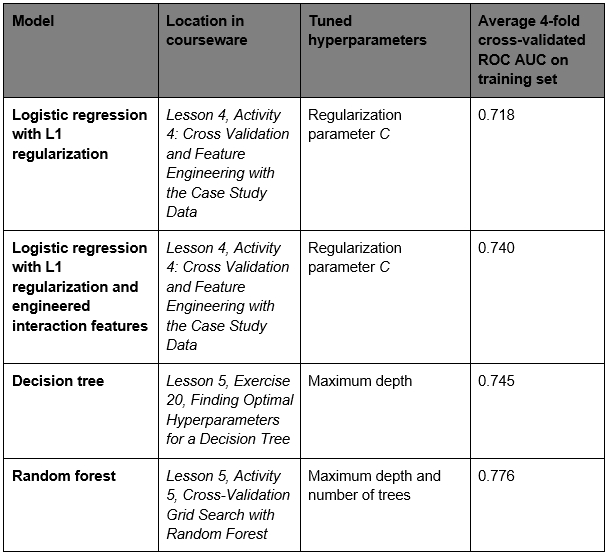
Figure 6.1: Summary of modeling activities with case study data
From Figure 6.1, we can see that for this particular problem, our efforts in creating more complex models,...