Confusion Matrix
A confusion matrix describes the performance of the classification model. In other words, confusion matrix is a way to summarize classifier performance. The following figure shows a basic representation of a confusion matrix:
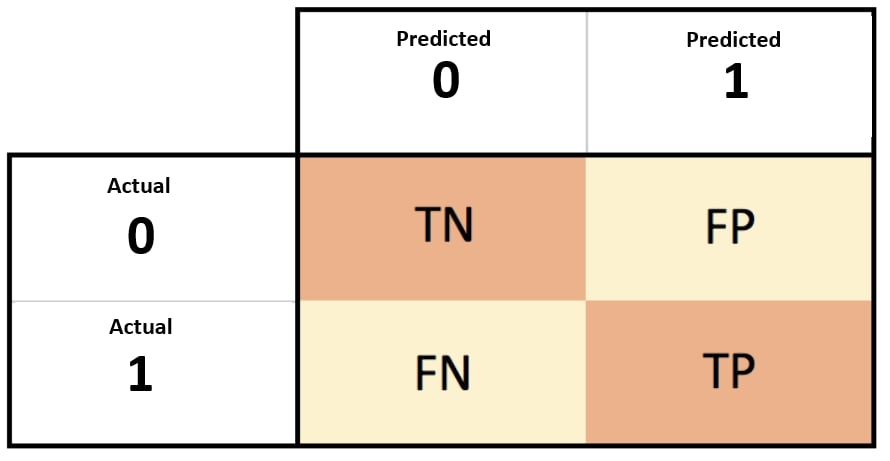
Figure 6.5: Basic representation of a confusion matrix
The following code is an example of a confusion matrix:
from sklearn.metrics import confusion_matrix cm=confusion_matrix(y_test,y_pred_class) print(cm)
The following figure shows the output of the preceding code:

Figure 6.6: Example confusion matrix
These are the meanings of the abbreviations used in the preceding figure:
TN (True negative): This is the count of outcomes that were originally negative and were predicted negative.
FP (False positive): This is the count of outcomes that were originally negative but were predicted positive. This error is also called a type 1 error
FN (False negative): This is the count of outcomes that were originally positive but were predicted negative. This error is also...