YOLO v2 (also known as YOLO9000) increased YOLO's original input size from 224x224 to 448x448. It was observed that this increase in size resulted in an improved mAP. YOLO v2 also uses batch normalization, which leads to a significant improvement in the accuracy of the model. It also resulted in an improvement in the detection of small objects, which was achieved by dividing the entire image using a 13x13 grid. In order to obtain good priors (anchors) for the model, YOLO v2 runs k-means clustering on the bounding box scale. YOLO v2Â also uses five anchor boxes, as shown in the following image:
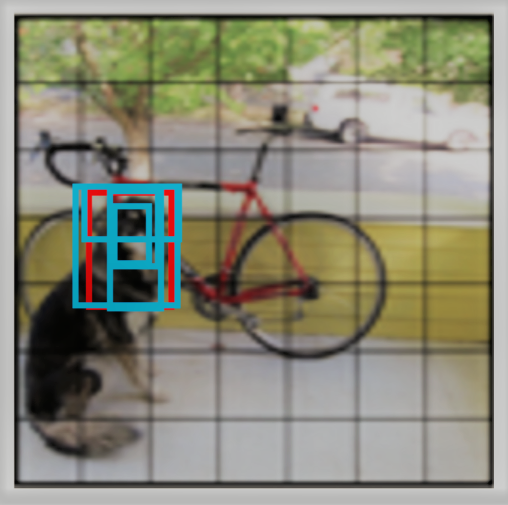
In the preceding image, the boxes in blue are anchor boxes, while the box in red is the ground truth box for the object.
YOLOv2 uses the Darknet architecture for object classification and has 19 convolution layers, five max-pooling layers, and a softmax layer.