2. Least-squares GAN (LSGAN)
LSGAN proposes the least squares loss. Figure 5.2.1 demonstrates why the use of a sigmoid cross-entropy loss in GANs results in poorly generated data quality:
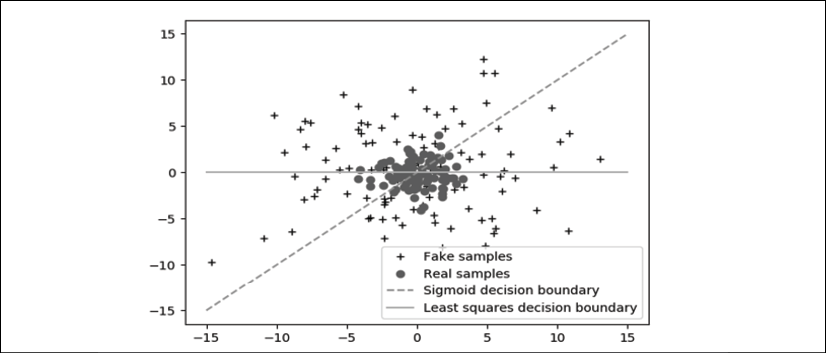
Figure 5.2.1: Both real and fake sample distributions divided by their respective decision boundaries: sigmoid and least squares
Ideally, the fake sample distribution should be as close as possible to the true samples' distribution. However, for GANs, once the fake samples are already on the correct side of the decision boundary, the gradients vanish.
This prevents the generator from having enough motivation to improve the quality of the generated fake data. Fake samples far from the decision boundary will no longer attempt to move closer to the true samples' distribution. Using the least squares loss function, the gradients do not vanish as long as the fake sample distribution is far from the real samples' distribution. The generator will strive to improve...