



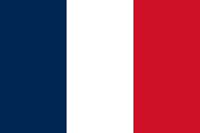


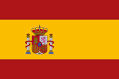



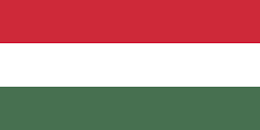

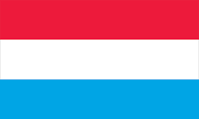





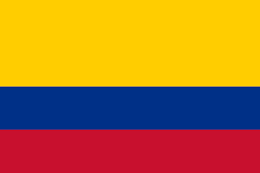
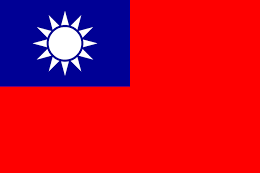
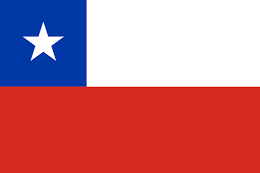



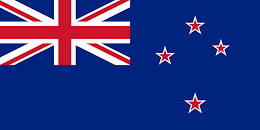
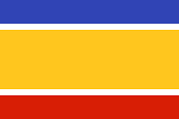
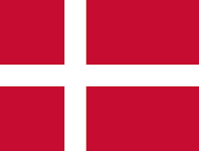
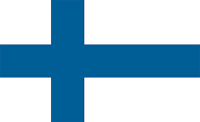







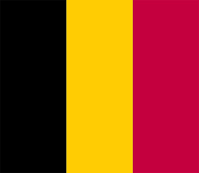

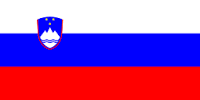





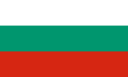


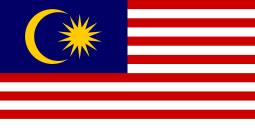



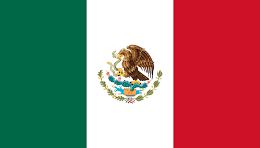

[box type="note" align="" class="" width=""]The following article is an excerpt taken from the book Statistics for Data Science, authored by James D. Miller. The book dives into the different statistical approaches to discover hidden insights and patterns from different kinds of data.[/box]
Being a data scientist is undoubtedly a very lucrative career prospect. In fact, it is one of the highest paying jobs in the world right now. That said, transitioning from a data developer role to a data scientist role needs careful planning and a clear understanding of what the role is all about. In this interesting article, the author highlights six key skills must give special attention to, during this transition.
Let's start by taking a moment to state what I consider to be a few generally accepted facts about transitioning to a data scientist. We'll reaffirm these beliefs as we continue through this book:
Okay, having made the previous declarations, let's also be realistic. As always, there is an entry-point for everything in life, and, to give credit where it is due, the more credentials you can acquire to begin out with, the better off you will most likely be. Nonetheless, (as we'll see later in this chapter), there is absolutely no valid reason why you cannot begin understanding, using, and being productive with data science and statistics immediately.
[box type="info" align="" class="" width=""]As with any profession, certifications and degrees carry the weight that may open the doors, while experience, as always, might be considered the best teacher. There are, however, no fake data scientists but only those with currently more desire than practical experience.[/box]
If you are seriously interested in not only understanding statistics and data science but eventually working as a full-time data scientist, you should consider the following common themes (you're likely to find in job postings for data scientists) as areas to focus on:
Common fields of study here are Mathematics and Statistics, followed by Computer Science and Engineering (also Economics and Operations research). Once more, there is no strict requirement to have an advanced or even related degree. In addition, typically, the idea of a degree or an equivalent experience will also apply here.
You will hear SAS and R (actually, you will hear quite a lot about R) as well as Python, Hadoop, and SQL mentioned as key or preferable for a data scientist to be comfortable with, but tools and technologies change all the time so, as mentioned several times throughout this chapter, data developers can begin to be productive as soon as they understand the objectives of data science and various statistical mythologies without having to learn a new tool or language.
[box type="info" align="" class="" width=""]Basic business skills such as Omniture, Google Analytics, SPSS, Excel, or any other Microsoft Office tool are assumed pretty much everywhere and don't really count as an advantage, but experience with programming languages (such as Java, PERL, or C++) or databases (such as MySQL, NoSQL, Oracle, and so on.) does help![/box]
The ability to understand data and deal with the challenges specific to the various types of data, such as unstructured, machine-generated, and big data (including organizing and structuring large datasets).
[box type="info" align="" class="" width=""]Unstructured data is a key area of interest in statistics and for a data scientist. It is usually described as data having no redefined model defined for it or is not organized in a predefined manner. Unstructured information is characteristically text-heavy but may also contain dates, numbers, and various other facts as well.[/box]
I love this. This is perhaps well defined as a character trait that comes in handy (if not required) if you want to be a data scientist. This means that you have a continuing need to know more than the basics or want to go beyond the common knowledge about a topic (you don't need a degree on the wall for this!)
To be a data developer or a data scientist you need a deep understanding of the industry you're working in, and you also need to know what business problems your organization needs to unravel. In terms of data science, being able to discern which problems are the most important to solve is critical in addition to identifying new ways the business should be leveraging its data.
All companies look for individuals who can clearly and fluently translate their findings to a non-technical team, such as the marketing or sales departments. As a data scientist, one must be able to enable the business to make decisions by arming them with quantified insights in addition to understanding the needs of their non-technical colleagues to add value and be
successful. This article paints a much clearer picture on why soft skills play an important role in becoming a better data scientist.
So why should you, a data developer, endeavor to think like (or more like) a data scientist? Specifically, what might be the advantages of thinking like a data scientist? The following are just a few notions supporting the effort:
If you found this article to be useful, make sure you check out the book Statistics for Data Science, which includes a comprehensive list of tips and tricks to becoming a successful data scientist by mastering the basic and not-so-basic concepts of statistics.