



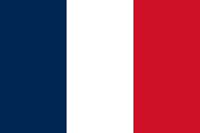


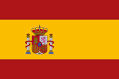




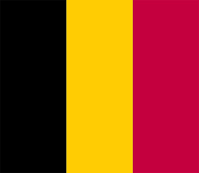
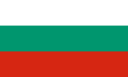
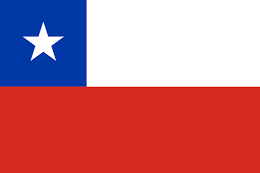
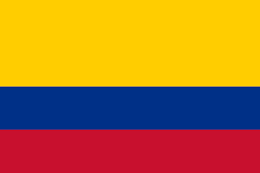
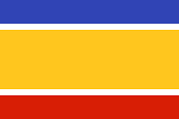

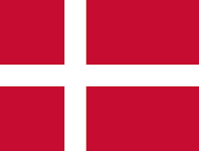



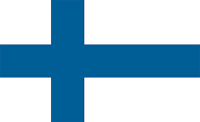

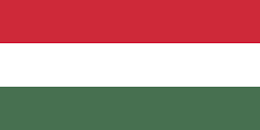






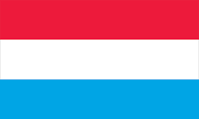
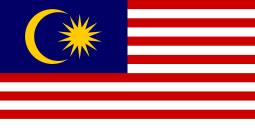

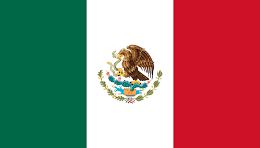

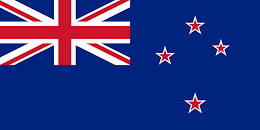







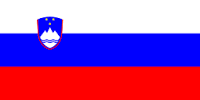




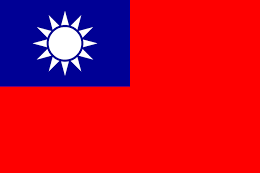



This article by Tarek Ziadé, author of the book Python Microservices Development explains the benefits and implementation of microservices with Python.
While the microservices architecture looks more complicated than its monolithic counterpart, its advantages are multiple. It offers the following benefits.
(For more resources related to this topic, see here.)
First of all, each microservice can be developed independently by a separate team. For instance, building a reservation service can be a full project on its own. The team in charge can make it in whatever programming language and database, as long as it has a well-documented HTTP API.
That also means the evolution of the app is more under control than with monoliths. For example, if the payment system changes its underlying interactions with the bank, the impact is localized inside that service and the rest of the application stays stable and under control.
This loose coupling improves a lot the overall project velocity as we're applying at the service level a similar philosophy than the single responsibility principle.
The single responsibility principle was defined by Robert Martin to explain that a class should have only one reason to change - in other words, each class should be providing a single, well-defined feature. Applied to microservices, it means that we want to make sure that each microservice focuses on a single role.
The second benefit is breaking the complexity of the project. When you are adding a feature to an application like the PDF reporting, even if you are doing it cleanly, you are making the base code bigger, more complicated and sometimes slower. Building that feature in a separate application avoids this problem, and makes it easier to write it with whatever tools you want. You can refactor it often and shorten your release cycles, and stay on the top of things. The growth of the application remains under your control.
Dealing with a smaller project also reduces risks when improving the application: if a team wants to try out the latest programming language or framework, they can iterate quickly on a prototype that implements the same microservice API, try it out, and decide whether or not to stick with it.
One real-life example in mind is the Firefox Sync storage microservice. There are currently some experiments to switch from the current Python+MySQL implementation to a Go based one that stores users data in standalone SQLite databases. That prototype is highly experimental, but since we have isolated the storage feature in a microservice with a well-defined HTTP API, it's easy enough to give it a try with a small subset of the user base.
Last, having your application split into components makes it easier to scale depending on your constraints. Let's say you are starting to get a lot of customers that are booking hotels daily, and the PDF generation is starting to heat up the CPUs. You can deploy that specific microservice in some servers that have bigger CPUs.
Another typical example is RAM-consuming microservices like the ones that are interacting with memory databases like Redis or Memcache. You could tweak your deployments consequently by deploying them on servers with less CPU and a lot more RAM.
To summarize microservices benefits:
Microservices architectures are good at solving a lot of the problems that may arise once your application is starting to grow. Although, we need to be aware of some of the new issues they also bring in practice.
Python is an amazingly versatile language.
As you probably already know, it's used to build many different kinds of applications, from simple system scripts that perform tasks on a server, to large object-oriented applications that run services for millions of users.
According to a study conducted by Philip Guo in 2014, published in the Association for Computing Machinery (ACM) website, Python has surpassed Java in top U.S. universities and is the most popular language to learn Computer Science.
This trend is also true in the software industry. Python sits now in the top 5 languages in the TIOBE index (http://www.tiobe.com/tiobe-index/), and it's probably even bigger in the web development land since languages like C are rarely used as main languages to build web applications.
However, some developers criticize Python for being slow and unfit for building efficient web services. Python is slow, and this is undeniable. But it's still is a language of choice for building microservices, and many major companies are happily using it.
This section will give you some background on the different ways you can write microservices using Python, some insights on asynchronous versus synchronous programming, and conclude with some details on Python performances.
It's composed of 4 parts:
What strikes the most web developers that are starting with Python is how easy it is to get a web application up and running.
The Python web community has created a standard inspired from the Common Gateway Interface (CGI) called Web Server Gateway Interface (WSGI) that simplifies a lot how you can write a Python application which goal is to serve HTTP requests.
When your code is using that standard, your project can be executed by standard web servers like Apache or NGinx, using WSGI extensions like uwsgi or mod_wsgi.
Your application just has to deal with incoming requests and send back JSON responses, and Python includes all that goodness in its standard library.
You can create a fully functional microservice that returns the server's local time with a vanilla Python module of fewer than ten lines:
import JSON
import time
def application(environ, start_response):
headers = [('Content-type', 'application/json')]
start_response('200 OK', headers)
return bytes(json.dumps({'time': time.time()}), 'utf8')
Since its introduction, the WSGI protocol became an essential standard and the Python web community widely adopted it. Developers wrote middlewares, which are functions you can hook before or after the WSGI application function itself, to do something within the environment.
Some web frameworks were created specifically around that standard, like Bottle (http://bottlepy.org) - and soon enough, every framework out there could be used through WSGI in a way or another.
The biggest problem with WSGI though is its synchronous nature. The application function you see above is called exactly once per incoming request, and when the function returns, it has to send back the response. That means that every time you are calling the function, it will block until the response is ready.
And writing microservices means your code will be waiting for responses from various network resources all the time. In other words, your application will idle and just block the client until everything is ready.
That's an entirely okay behavior for HTTP APIs. We're not talking about building bidirectional applications like web socket based ones. But what happens when you have several incoming requests that are calling your application at the same time?
WSGI servers will let you run a pool of threads to serve several requests concurrently. But you can't run thousands of them, and as soon as the pool is exhausted, the next request will be blocking even if your microservice is doing nothing but idling and waiting for backend services responses.
That's one of the reasons why non-WSGI frameworks like Twisted, Tornado and in Javascript land Node.js became very successful - it's fully async.
When you're coding a Twisted application, you can use callbacks to pause and resume the work done to build a response. That means you can accept new requests and start to treat them. That model dramatically reduces the idling time in your process. It can serve thousands of concurrent requests. Of course, that does not mean the application will return each single response faster. It just means one process can accept more concurrent requests and juggle between them as the data is getting ready to be sent back.
There's no simple way with the WSGI standard to introduce something similar, and the community has debated for years to come up with a consensus - and failed. The odds are that the community will eventually drop the WSGI standard for something else.
In the meantime, building microservices with synchronous frameworks is still possible and completely fine if your deployments take into account the one request == one thread limitation of the WSGI standard.
There's, however, one trick to boost synchronous web applications: greenlets.
The general principle of asynchronous programming is that the process deals with several concurrent execution contexts to simulate parallelism.
Asynchronous applications are using an event loop that pauses and resumes execution contexts when an event is triggered - only one context is active, and they take turns. Explicit instruction in the code will tell the event loop that this is where it can pause the execution.
When that occurs, the process will look for some other pending work to resume. Eventually, the process will come back to your function and continue it where it stopped - moving from an execution context to another is called switching.
The Greenlet project (https://github.com/python-greenlet/greenlet) is a package based on the Stackless project, a particular CPython implementation, and provides greenlets.
Greenlets are pseudo-threads that are very cheap to instantiate, unlike real threads, and that can be used to call python functions. Within those functions, you can switch and give back the control to another function. The switching is done with an event loop and allows you to write an asynchronous application using a Thread-like interface paradigm.
Here's an example from the Greenlet documentation
def test1(x, y):
z = gr2.switch(x+y)
print z
def test2(u):
print u
gr1.switch(42)
gr1 = greenlet(test1)
gr2 = greenlet(test2)
gr1.switch("hello", " world")
The two greenlets are explicitly switching from one to the other.
For building microservices based on the WSGI standard, if the underlying code was using greenlets we could accept several concurrent requests and just switch from one to another when we know a call is going to block the request - like performing a SQL query.
Although, switching from one greenlet to another has to be done explicitly, and the resulting code can quickly become messy and hard to understand. That's where Gevent can become very useful.
The Gevent project (http://www.gevent.org/) is built on the top of Greenlet and offers among other things an implicit and automatic way of switching between greenlets.
It provides a cooperative version of the socket module that will use greenlets to automatically pause and resume the execution when some data is made available in the socket. There's even a monkey patch feature that will automatically replace the standard lib socket with Gevent's version. That makes your standard synchronous code magically asynchronous every time it uses sockets - with just one extra line.
from gevent import monkey; monkey.patch_all()
def application(environ, start_response):
headers = [('Content-type', 'application/json')]
start_response('200 OK', headers)
# ...do something with sockets here...
return result
This implicit magic comes with a price, though. For Gevent to work well, all the underlying code needs to be compatible with the patching Gevent is doing. Some packages from the community will continue to block or even have unexpected results because of this. In particular, if they use C extensions and bypass some of the features of the standard library Gevent patched.
But for most cases, it works well. Projects that are playing well with Gevent are dubbed "green," and when a library is not functioning well, and the community asks its authors to "make it green," it usually happens.
That's what was used to scale the Firefox Sync service at Mozilla for instance.
If you are building microservices where increasing the number of concurrent requests you can hold is important, it's tempting to drop the WSGI standard and just use an asynchronous framework like Tornado (http://www.tornadoweb.org/) or Twisted (https://twistedmatrix.com/trac/).
Twisted has been around for ages. To implement the same microservices you need to write a slightly more verbose code:
import time
from twisted.web import server, resource
from twisted.internet import reactor, endpoints
class Simple(resource.Resource):
isLeaf = True
def render_GET(self, request):
request.responseHeaders.addRawHeader(b"content-type", b"application/json")
return bytes(json.dumps({'time': time.time()}), 'utf8')
site = server.Site(Simple())
endpoint = endpoints.TCP4ServerEndpoint(reactor, 8080)
endpoint.listen(site)
reactor.run()
While Twisted is an extremely robust and efficient framework, it suffers from a few problems when building HTTP microservices:
Tornado is based on a similar model but is doing a better job in some areas. It has a lighter routing system and does everything possible to make the code closer to plain Python. Tornado is also using a callback model, so debugging can be hard.
But both frameworks are working hard at bridging the gap to rely on the new async features introduced in Python 3.
When Guido van Rossum started to work on adding async features in Python 3, part of the community pushed for a Gevent-like solution because it made a lot of sense to write applications in a synchronous, sequential fashion - rather than having to add explicit callbacks like in Tornado or Twisted.
But Guido picked the explicit technique and experimented in a project called Tulip that Twisted inspired. Eventually, asyncio was born out of that side project and added into Python.
In hindsight, implementing an explicit event loop mechanism in Python instead of going the Gevent way makes a lot of sense. The way the Python core developers coded asyncio and how they elegantly extended the language with the async and await keywords to implement coroutines, made asynchronous applications built with vanilla Python 3.5+ code look very elegant and close to synchronous programming.
By doing this, Python did a great job at avoiding the callback syntax mess we sometimes see in Node.js or Twisted (Python 2) applications.
And beyond coroutines, Python 3 has introduced a full set of features and helpers in the asyncio package to build asynchronous applications, see https://docs.python.org/3/library/asyncio.html.
Python is now as expressive as languages like Lua to create coroutine-based applications, and there are now a few emerging frameworks that have embraced those features and will only work with Python 3.5+ to benefit from this.
KeepSafe's aiohttp (http://aiohttp.readthedocs.io) is one of them, and building the same microservice, fully asynchronous, with it would simply be these few elegant lines.
from aiohttp import web
import time
async def handle(request):
return web.json_response({'time': time.time()})
if __name__ == '__main__':
app = web.Application()
app.router.add_get('/', handle)
web.run_app(app)
In this small example, we're very close to how we would implement a synchronous app. The only hint we're async is the async keyword marking the handle function as being a coroutine.
And that's what's going to be used at every level of an async Python app going forward. Here's another example using aiopg - a Postgresql lib for asyncio. From the project documentation:
import asyncio
import aiopg
dsn = 'dbname=aiopg user=aiopg password=passwd host=127.0.0.1'
async def go():
pool = await aiopg.create_pool(dsn)
async with pool.acquire() as conn:
async with conn.cursor() as cur:
await cur.execute("SELECT 1")
ret = []
async for row in cur:
ret.append(row)
assert ret == [(1,)]
loop = asyncio.get_event_loop()
loop.run_until_complete(go())
With a few async and await prefixes, the function that's performing a SQL query and send back the result looks a lot like a synchronous function.
But asynchronous frameworks and libraries based on Python 3 are still emerging, and if you are using asyncio or a framework like aiohttp, you will need to stick with particular asynchronous implementations for each feature you need.
If you require using a library that is not asynchronous in your code, using it from your asynchronous code means you will need to go through some extra and challenging work if you want to prevent blocking the event loop.
If your microservices are dealing with a limited number of resources, it could be manageable. But it's probably a safer bet at this point (2017) to stick with a synchronous framework that's been around for a while rather than an asynchronous one. Let's enjoy the existing ecosystem of mature packages, and wait until the asyncio ecosystem gets more sophisticated.
And there are many great synchronous frameworks to build microservices with Python, like Bottle, Pyramid with Cornice or Flask.
In the previous sections we've been through the two different ways to write microservices - asynchronous vs. synchronous, and whatever technique you are using, the speed of Python is directly impacting the performance of your microservice.
Of course, everyone knows Python is slower than Java or Go - but execution speed is not always the top priority. A microservice is often a thin layer of code that is sitting most of its life waiting for some network responses from other services. Its core speed is usually less important than how fast your SQL queries will take to return from your Postgres server because the latter will represent most of the time spent to build the response.
But wanting an application that's as fast as possible is legitimate.
One controversial topic in the Python community around speeding up the language is how the Global Interpreter Lock (GIL) mutex can ruin performances because multi-threaded applications cannot use several processes.
The GIL has good reasons to exist. It protects non thread-safe parts of the CPython interpreter and exists in other languages like Ruby. And all attempts to remove it so far have failed to produce a faster CPython implementation.
For microservices, besides preventing the usage of multiple cores in the same process, the GIL will slightly degrade performances on high load, because of the system calls overhead introduced by the mutex.
Although, all the scrutiny around the GIL had one beneficial impact: some work has been done in the past years to reduce its contention in the interpreter, and in some area, Python performances have improved a lot.
But bear in mind that even if the core team removes the GIL, Python is an interpreted language and the produced code will never be very efficient at execution time. Python provides the dis module if you are interested to see how the interpreter decomposes a function.
In the example below, the interpreter will decompose a simple function that yields incremented values from a sequence in no less than 29 steps!
>>> def myfunc(data):
... for value in data:
... yield value + 1
...
>>> import dis
>>> dis.dis(myfunc)
2 0 SETUP_LOOP 23 (to 26)
3 LOAD_FAST 0 (data)
6 GET_ITER
>> 7 FOR_ITER 15 (to 25)
10 STORE_FAST 1 (value)
3 13 LOAD_FAST 1 (value)
16 LOAD_CONST 1 (1)
19 BINARY_ADD
20 YIELD_VALUE
21 POP_TOP
22 JUMP_ABSOLUTE 7
>> 25 POP_BLOCK
>> 26 LOAD_CONST 0 (None)
29 RETURN_VALUE
A similar function written in a statically compiled language will dramatically reduce the number of operations required to produce the same result.
There are ways to speed up Python execution, though.
One is to write part of your code into compiled code by building C extensions or using a static extension of the language like Cython (http://cython.org/) - but that makes your code more complicated.
Another solution, which is the most promising one, is by simply running your application using the PyPy interpreter (http://pypy.org/).
PyPy implements a Just-In-Time compiler (JIT). This compiler is directly replacing at run time pieces of Python with machine code that can be directly used by the CPU. The whole trick for the JIT is to detect in real time, ahead of the execution, when and how to do it.
Even if PyPy is always a few Python versions behind CPython, it reached a point where you can use it in production, and its performances can be quite amazing. In one of our projects at Mozilla that needs fast execution, the PyPy version was almost as fast as the Go version, and we've decided to use Python there instead.
However, if your program uses C extensions, you will need to recompile them for PyPy, and that can be a problem. In particular, if other developers maintain some of the extensions you are using.
But if you are building your microservice with a standard set of libraries, the chances are that will it work out of the box with the PyPy interpreter, so that's worth a try.
In any case, for most projects, the benefits of Python and its ecosystem largely surpasses the performances issues described in this section because the overhead in a microservice is rarely a problem.
In this article we saw that Python is considered to be one of the best languages to write web applications, and therefore microservices - for the same reasons, it's a language of choice in other areas and also because it provides tons of mature frameworks and packages to do the work.
Further resources on this subject: