




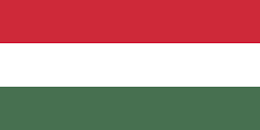

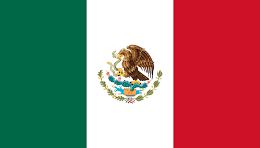


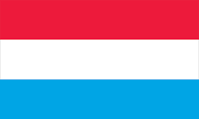



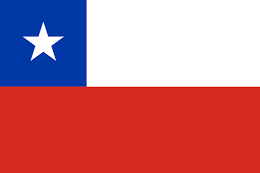



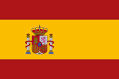


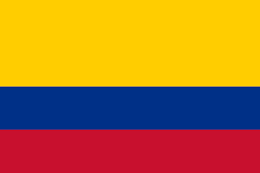
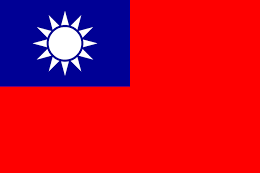


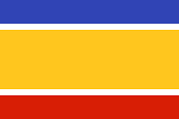
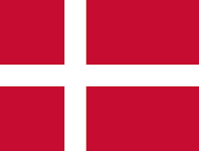
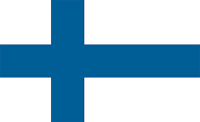



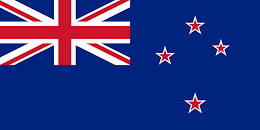


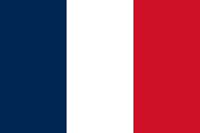



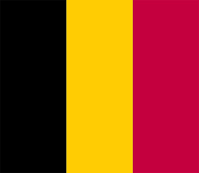

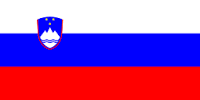




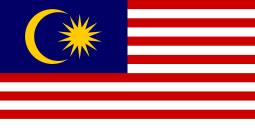


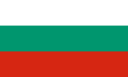




This session explains what Fairness, Accountability, and Transparency means in the context of recommendation. The session also includes a paper that talks about predictive policing, which is defined as ‘Given historical crime incident data for a collection of regions, decide how to allocate patrol officers to areas to detect crime.’
The Conference on Fairness, Accountability, and Transparency (FAT), which would be held on the 23rd and 24th of February, 2018 is a multi-disciplinary conference that brings together researchers and practitioners interested in fairness, accountability, and transparency in socio-technical systems.
The FAT 2018 conference will witness 17 research papers, 6 tutorials, and 2 keynote presentations from leading experts in the field. This article covers research papers pertaining to the 5th session that is dedicated to FAT Recommenders, etc.
Predictive policing systems are increasingly being used to determine how to allocate police across a city in order to best prevent crime. To update the model, discovered crime data (e.g., arrest counts) are used. Such systems have been empirically shown to be susceptible to runaway feedback loops, where police are repeatedly sent back to the same neighborhoods regardless of the true crime rate.
This paper is in response to this system, where the authors have developed a mathematical model of predictive policing that proves why this feedback loop occurs.The paper also empirically shows how this model exhibits such problems, and demonstrates ways to change the inputs to a predictive policing system (in a black-box manner) so the runaway feedback loop does not occur, allowing the true crime rate to be learned.
Key takeaways:
There have been many advances in the information retrieval evaluation, which demonstrate the importance of considering the distribution of effectiveness across diverse groups of varying sizes. This paper addresses this question, ‘do users of different ages or genders obtain similar utility from the system, particularly if their group is a relatively small subset of the user base?’
The authors have applied this consideration to recommender systems, using offline evaluation and a utility-based metric of recommendation effectiveness to explore whether different user demographic groups experience similar recommendation accuracy. The paper shows that there are demographic differences in measured recommender effectiveness across two data sets containing different types of feedback in different domains; these differences sometimes, but not always, correlate with the size of the user group in question. Demographic effects also have a complex— and likely detrimental—interaction with popularity bias, a known deficiency of recommender evaluation.
Key takeaways:
In this paper the authors have showcased new methods that can deal with variance of recommendation outcomes without increasing the computational complexity. These methods can more strictly remove the sensitive information, and experimental results demonstrate that the new algorithms can more effectively eliminate the factors that undermine fairness. Additionally, the paper also explores potential applications for independence enhanced recommendation, and discuss its relation to other concepts, such as recommendation diversity.
Key takeaways from the paper:
In this paper, the authors examine two different cases of fairness-aware recommender systems: consumer-centered and provider-centered. The paper explores the concept of a balanced neighborhood as a mechanism to preserve personalization in recommendation while enhancing the fairness of recommendation outcomes. It shows that a modified version of the Sparse Linear Method (SLIM) can be used to improve the balance of user and item neighborhoods, with the result of achieving greater outcome fairness in real-world datasets with minimal loss in ranking performance.
Key takeaways:
If you’ve missed our summaries on the previous sessions, visit the article links to be on track.
Session 1: Online Discrimination and Privacy
Session 2: Interpretability and Explainability