Introduction
Reinforcement Learning (RL) is an area in machine learning that is inspired by psychology, such as how agents (software programs) can take actions in order to maximize cumulative rewards.
The RL is reward-based learning where the reward comes at the end or is distributed during the learning. For example, in chess, the reward will be assigned to winning or losing the game whereas in games such as tennis, every point won is a reward. Some of the commercial examples of RL are DeepMind from Google uses RL to master parkour. Similarly, Tesla is developing AI-driven technology using RL. An example of reinforcement architecture is shown in the following figure:
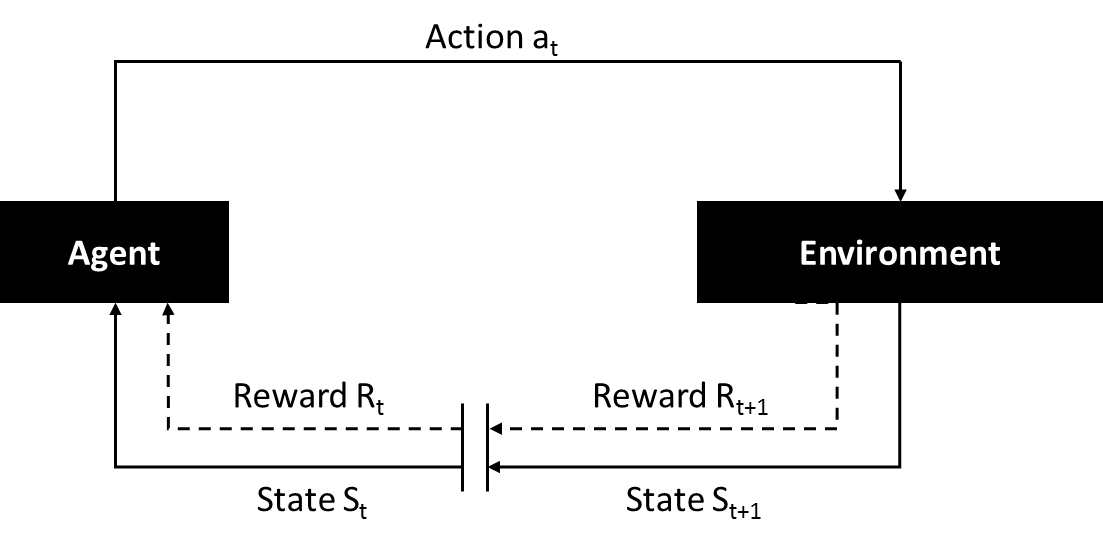
Interaction of an agent with environment in Reinforcement Learning
The basic notations for RL are as follows:
- T(s, a, s'): Represents the transition model for reaching state s' when action a is taken at state s
- : Represents a policy which defines what action to take at every possible state
- R(s): Denotes the reward received by agent...