So far, we have learned to construct CNN architectures by designing the work in isolation to solve specific tasks. Neural network models are depth intensive, require lots of training data, training runs, and expert knowledge of tuning to achieve high accuracy; however as human beings, we don’t learn everything from scratch—we learn from others and we learn from the cloud (internet). Transfer learning is useful when data is insufficient for a new class that we are trying to analyze, but there is a large amount of preexisting data on a similar class. Each of the CNN models (AlexNet, VGG16, ResNet, and inception) have been trained on ImageNet ILSVRC competition datasets. ImageNet is a dataset with over 15 million labeled images in 22,000 categories. ILSVRC uses a subset of ImageNet with around 1,000 images in each of the 1,000 categories...




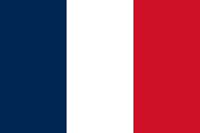


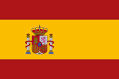



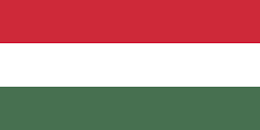

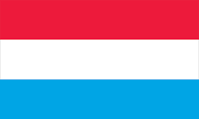





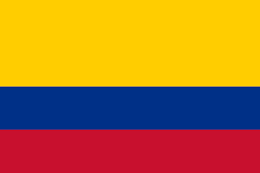
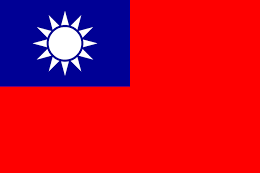
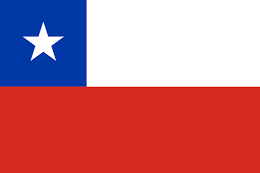



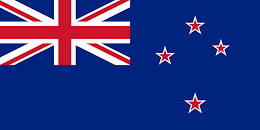
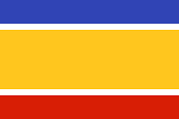
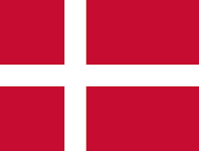
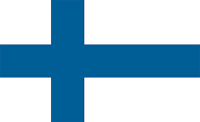







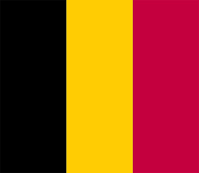

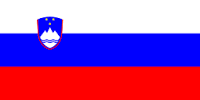





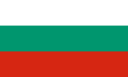


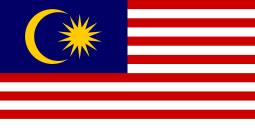



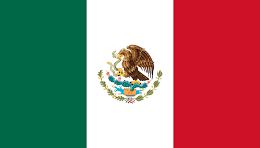
