In this chapter, we explored the fundamental theory behind autoencoders at a high level, and conceptualized the underlying mathematics that permits these models to learn. We saw several variations of the autoencoder architecture, including shallow, deep, undercomplete, and overcomplete models. This allowed us to overview considerations related to the representational power of each type of model and their propensity to overfit given too much capacity. We also explored some regularization techniques that let us compensate for the overfitting problem, such as the sparse and contractive autoencoders. Finally, we trained several different types of autoencoder networks, including shallow, deep, and convolutional networks, for the tasks of image reconstruction and denoising. We saw that with very little learning capacity and training time, convolutional autoencoders outperformed...




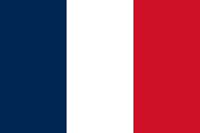


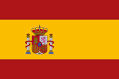



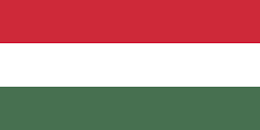

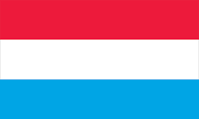





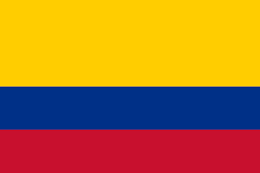
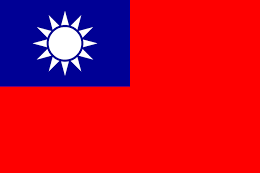
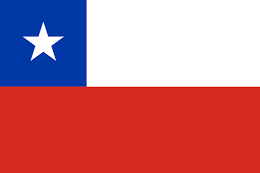



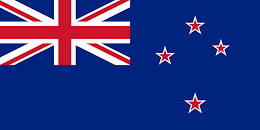
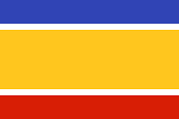
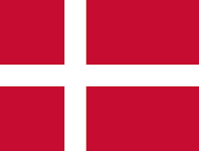
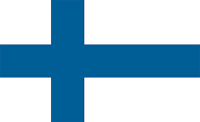







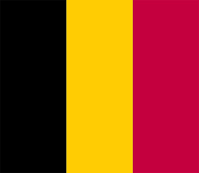

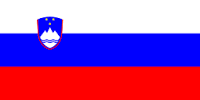





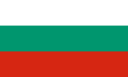


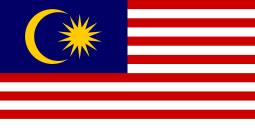



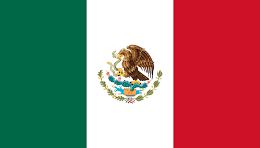
