In this chapter, we're going to introduce some common topic modeling methods, discussing some applications. Topic modeling is a very important NLP section and its purpose is to extract semantic pieces of information out of a corpus of documents. We're going to discuss latent semantic analysis, one of most famous methods; it's based on the same philosophy already discussed for model-based recommendation systems. We'll also discuss its probabilistic variant, PLSA, which is aimed at building a latent factor probability model without any assumption of prior distributions. On the other hand, the Latent Dirichlet Allocation is a similar approach that assumes a prior Dirichlet distribution for latent variables. In the last section, we're going to discuss sentiment analysis with a concrete example based on a Twitter...




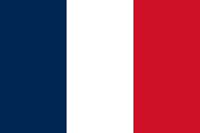


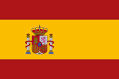




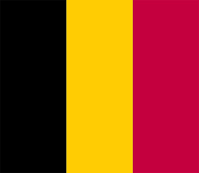
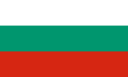
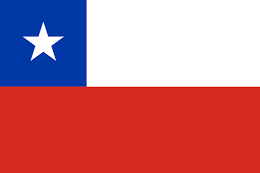
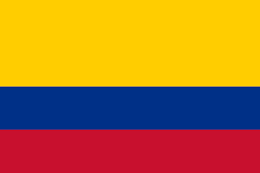
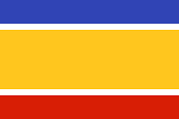

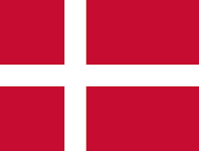



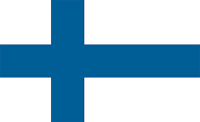

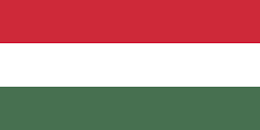






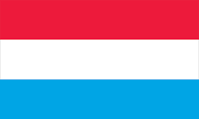
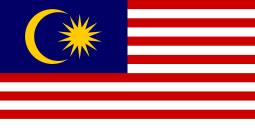

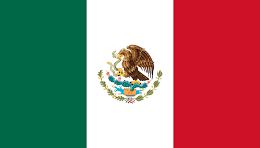

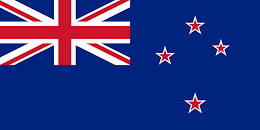







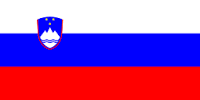




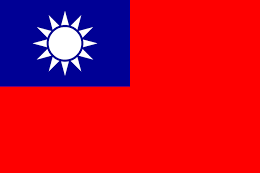


