We have just installed and configured our Keras environment, and we can now focus on the implementation of our model based on deep neural networks. When developing a deep learning application, we follow a general pipeline characterized by the following steps:
- Collecting the data: Everything starts from the data, no doubt about it, but one might wonder where so much data comes from. In practice, it is collected through lengthy procedures that may, for example, derive from measurement campaigns or face-to-face interviews. In all cases, the data is collected in a database so that it can then be analyzed to derive knowledge.
If we do not have specific requirements, to save time and effort we can use publicly available data. In this regard, a large collection of data is available in the UCI Machine Learning Repository at the following link: https://archive.ics.uci.edu/ml/index.php.
- Preparing the data: We have collected the data; now we have to prepare it for the next step. Once we have this data, we must make sure it is in a format usable by the algorithm we want to use. To do this, you may need to do some formatting. Recall that some algorithms need data in an integer format, whereas others require data in the form of strings. Finally, others need to be in a special format. We will get to this later, but the specific formatting is usually simple compared to data collection.
The following diagram shows the deep learning process workflow:
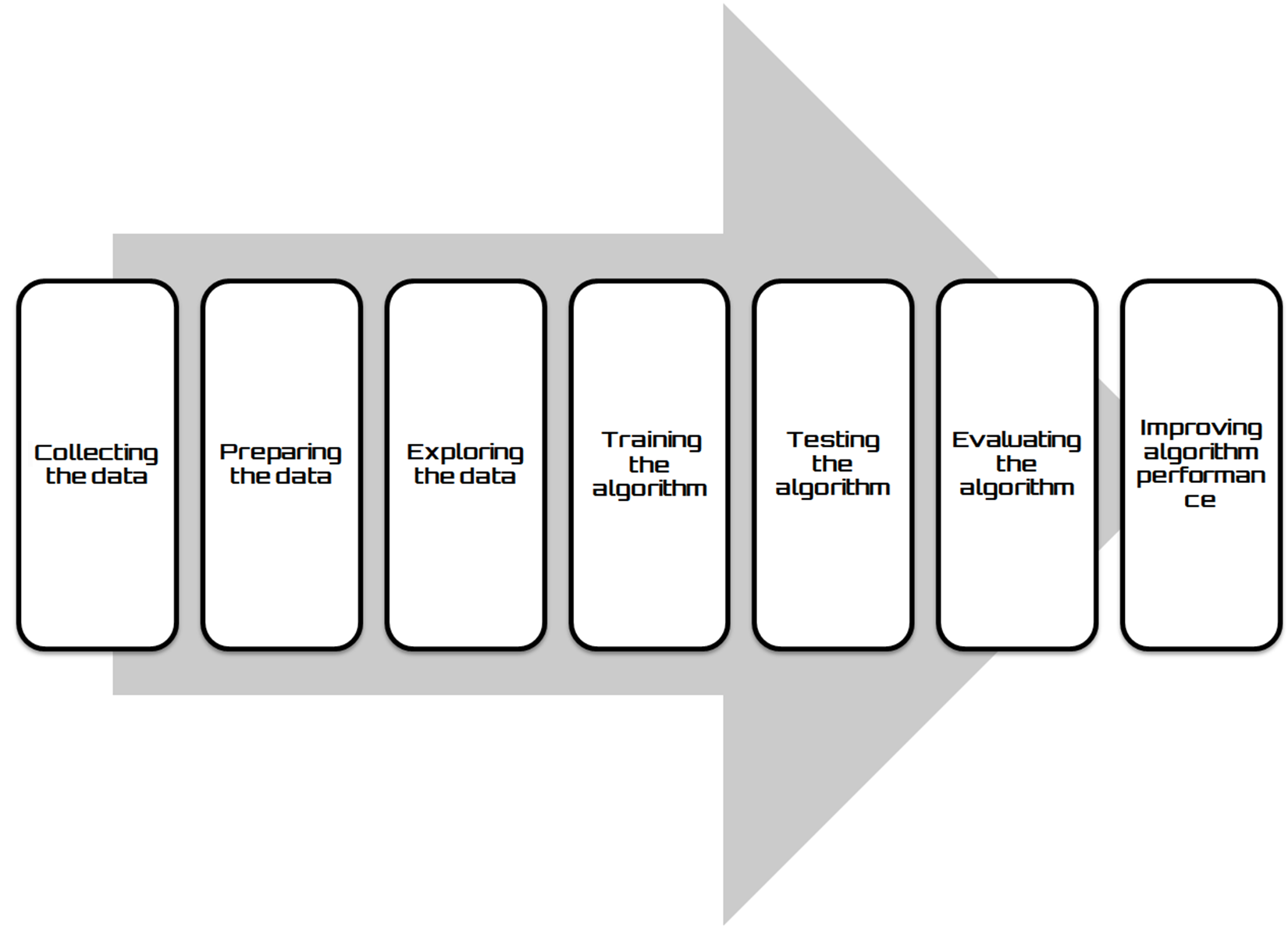
- Exploring the data: At this point, we can look at data to verify that it is actually working and we do not have a bunch of empty values. In this step, through the use of plots, we can recognize patterns or whether there are some data points that are vastly different from the rest of the set. Plotting data in one, two, or three dimensions can also help.
- Training the algorithm: Now, let's get serious. In this step, the deep learning begins to work with the definition of the model and the next training round. The model starts to extract knowledge from large amounts of data that we had available. For unsupervised learning, there's no training step because you don't have a target value.
- Testing the algorithm: In this step, we use the information learned in the previous step to see if the model actually works. The evaluation of an algorithm verifies how well the model approximates the real system. In the case of supervised learning, we have some known values that we can use to evaluate the algorithm. In unsupervised learning, we may need to use some other metrics to evaluate success. In both cases, if we are not satisfied, we can return to the previous steps, change some things, and retry the test.
- Evaluating the algorithm: We have reached the point where we can apply what has been done so far. We can assess the approximation ability of the model by applying it to real data. The model, preventively trained and tested, is then valued in this phase.
- Improving algorithm performance: Finally, we can focus on the finishing steps. We've verified that the model works, we have evaluated the performance, and now we are ready to analyze the whole process to identify any possible room for improvement.
In Keras, there are two ways to define a model—sequential, and functional API. The sequential model lets you create layer-by-layer models for most problems. Limits are dictated by the inability to create models that share levels or that have multiple inputs or outputs. Alternatively, the functional API allows you to create models with greater flexibility. We can easily define models in which the levels are connected in different ways and not just from the previous level to the next. In fact, we can link a layer to any other level, thus creating complex networks.