Manipulating images
As part of a computer vision pipeline for a self-driving car, with or without deep learning, you might need to process the video stream to make other algorithms work better as part of a preprocessing step.
This section will provide you with a solid foundation to preprocess any video stream.
Flipping an image
OpenCV provides the flip()
method to flip an image, and it accepts two parameters:
- The image
- A number that can be 1 (horizontal flip), 0 (vertical flip), or -1 (both horizontal and vertical flip)
Let's see a sample code:
flipH = cv2.flip(img, 1)flipV = cv2.flip(img, 0)flip = cv2.flip(img, -1)
This will produce the following result:
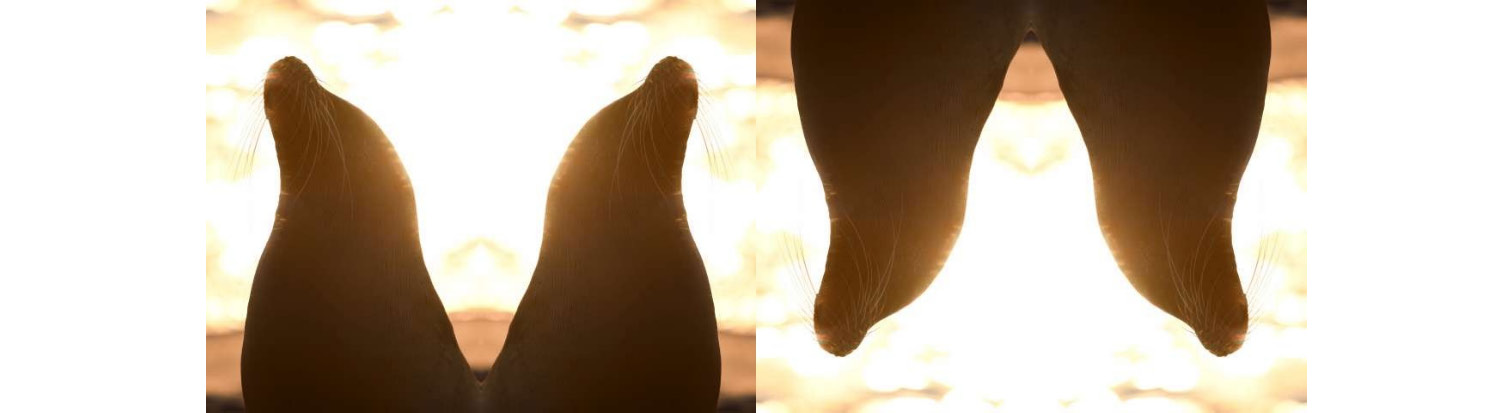
Figure 1.4 – Original image, horizontally flipped, vertically flipped, and both
As you can see, the first image is our original image, which was flipped horizontally and vertically, and then both, horizontally and vertically together.
Blurring an image
Sometimes, an image can be too noisy, possibly because of some processing steps that you have done. OpenCV provides several methods to blur an image, which can help in these situations. Most likely, you will have to take into consideration not only the quality of the blur but also the speed of execution.
The simplest method is blur()
, which applies a low-pass filter to the image and requires at least two parameters:
- The image
- The kernel size (a bigger kernel means more blur):
blurred = cv2.blur(image, (15, 15))
Another option is to use GaussianBlur()
, which offers more control and requires at least three parameters:
- The image
- The kernel size
sigmaX
, which is the standard deviation on X
It is recommended to specify both sigmaX
and sigmaY
(standard deviation on Y, the forth parameter):
gaussian = cv2.GaussianBlur(image, (15, 15), sigmaX=15, sigmaY=15)
An interesting blurring method is medianBlur()
, which computes the median and therefore has the characteristic of emitting only pixels with colors present in the image (which does not necessarily happen with the previous method). It is effective at reducing "salt and pepper" noise and has two mandatory parameters:
- The image
- The kernel size (an odd integer greater than 1):
median = cv2.medianBlur(image, 15)
There is also a more complex filter, bilateralFilter()
, which is effective at removing noise while keeping the edge sharp. It is the slowest of the filters, and it requires at least four parameters:
- The image
- The diameter of each pixel neighborhood
sigmaColor
: Filters sigma in the color space, affecting how much the different colors are mixed together, inside the pixel neighborhoodsigmaSpace
: Filters sigma in the coordinate space, affecting how distant pixels affect each other, if their colors are closer thansigmaColor
:
bilateral = cv2.bilateralFilter(image, 15, 50, 50)
Choosing the best filter will probably require some experiments. You might also need to consider the speed. To give you some ballpark estimations based on my tests, and considering that the performance is dependent on the parameters supplied, note the following:
blur()
is the fastest.GaussianBlur()
is similar, but it can be 2x slower than blur().medianBlur()
can easily be 20x slower thanblur()
.BilateralFilter()
is the slowest and can be 45x slower thanblur()
.
Here are the resultant images:
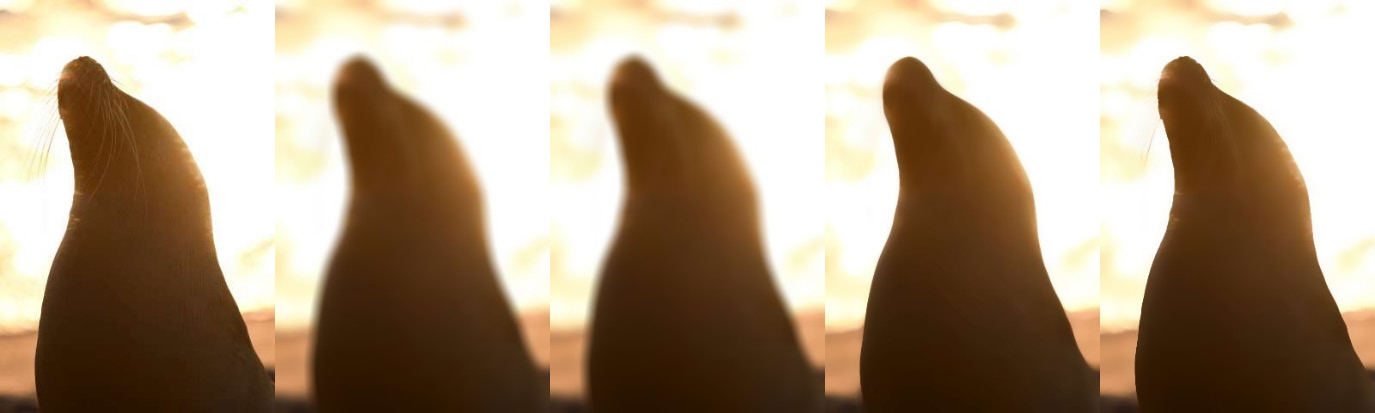
Figure 1.5 – Original, blur(), GaussianBlur(), medianBlur(), and BilateralFilter(), with the parameters used in the code samples
Changing contrast, brightness, and gamma
A very useful function is convertScaleAbs()
, which executes several operations on all the values of the array:
- It multiplies them by the scaling parameter,
alpha
. - It adds to them the delta parameter,
beta
. - If the result is above 255, it is set to 255.
- The result is converted into an unsigned 8-bit int.
The function accepts four parameters:
- The source image
- The destination (optional)
- The
alpha
parameter used for the scaling - The
beta
delta parameter
convertScaleAbs()
can be used to affect the contrast, as an alpha
scaling factor above 1 increases the contrast (amplifying the color difference between pixels), while a scaling factor below one reduces it (decreasing the color difference between pixels):
cv2.convertScaleAbs(image, more_contrast, 2, 0)cv2.convertScaleAbs(image, less_contrast, 0.5, 0)
It can also be used to affect the brightness, as the beta
delta factor can be used to increase the value of all the pixels (increasing the brightness) or to reduce them (decreasing the brightness):
cv2.convertScaleAbs(image, more_brightness, 1, 64) cv2.convertScaleAbs(image, less_brightness, 1, -64)
Let's see the resulting images:
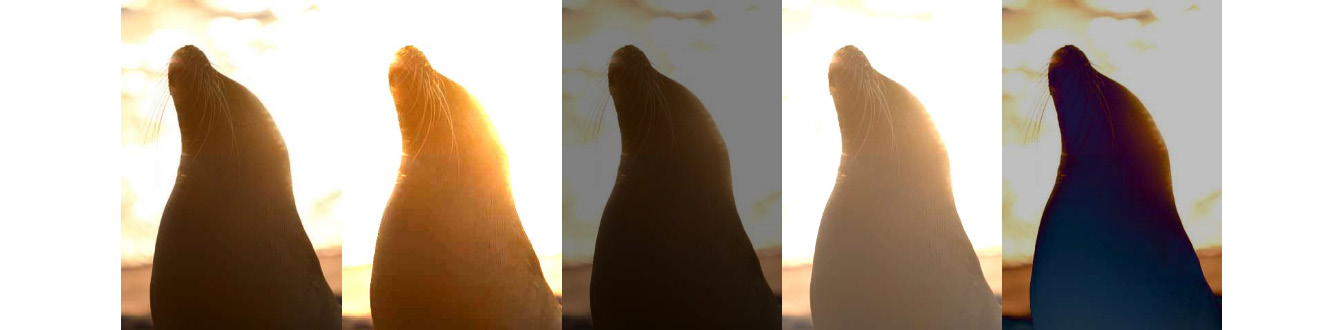
Figure 1.6 – Original, more contrast (2x), less contrast (0.5x), more brightness (+64), and less brightness (-64)
A more sophisticated method to change the brightness is to apply gamma correction. This can be done with a simple calculation using NumPy. A gamma value above 1 will increase the brightness, and a gamma value below 1 will reduce it:
Gamma = 1.5 g_1_5 = np.array(255 * (image / 255) ** (1 / Gamma), dtype='uint8') Gamma = 0.7 g_0_7 = np.array(255 * (image / 255) ** (1 / Gamma), dtype='uint8')
The following images will be produced:
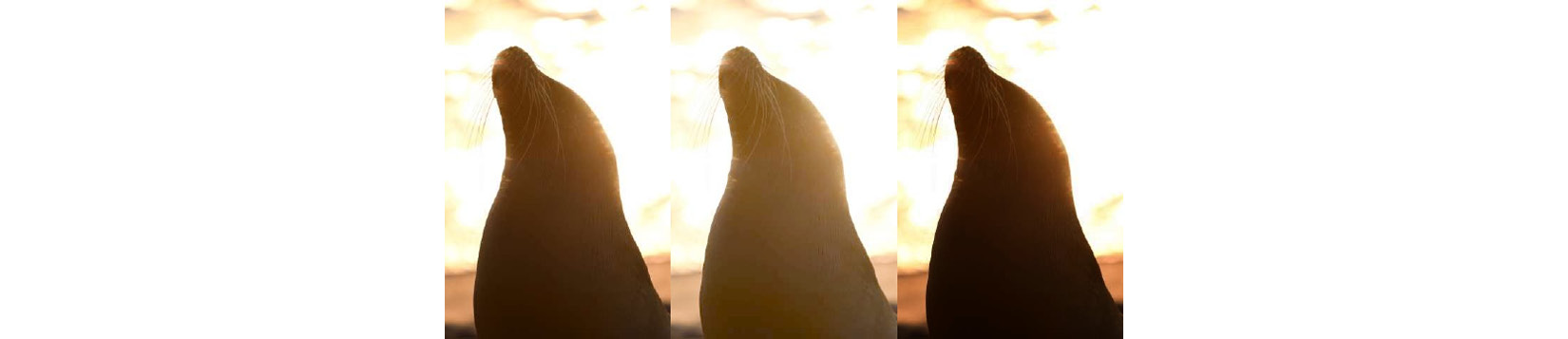
Figure 1.7 – Original, higher gamma (1.5), and lower gamma (0.7)
You can see the effect of different gamma values in the middle and right images.
Drawing rectangles and text
When working on object detection tasks, it is a common need to highlight an area to see what has been detected. OpenCV provides the rectangle()
function, accepting at least the following parameters:
- The image
- The upper-left corner of the rectangle
- The lower-right corner of the rectangle
- The color to use
- (Optional) The thickness:
cv2.rectangle(image, (x, y), (x + w, y + h), (255, 255, 255), 2)
To write some text in the image, you can use the putText()
method, accepting at least six parameters:
- The image
- The text to print
- The coordinates of the bottom-left corner
- The font face
- The scale factor, to change the size
- The color:
cv2.putText(image, 'Text', (x, y), cv2.FONT_HERSHEY_PLAIN, 2, clr)