Examining tabular datasets with fastai
In the previous section, we looked at the whole set of datasets curated by fastai. In this section, we are going to dig into a tabular dataset from the curated list. We will ingest the dataset, look at some example records, and then explore characteristics of the dataset, including the number of records and the number of unique values in each column.
Getting ready
Ensure you have followed the steps in Chapter 1, Getting Started with fastai, to get a fastai environment set up. Confirm that you can open the examining_tabular_datasets.ipynb
notebook in the ch2
directory of your repository.
I am grateful for the opportunity to include the ADULT_SAMPLE dataset featured in this section.
Dataset citation
Ron Kohavi. (1996) Scaling Up the Accuracy of Naive-Bayes Classifiers: a Decision-Tree Hybrid (http://robotics.stanford.edu/~ronnyk/nbtree.pdf).
How to do it…
In this section, you will be running through the examining_tabular_datasets.ipynb
notebook to examine the ADULT_SAMPLE
dataset.
Once you have the notebook open in your fastai environment, complete the following steps:
- Run the first two cells to import the necessary libraries and set up the notebook for fastai.
- Run the following cell to copy the dataset into your filesystem (if it's not already there) and to define the path for the dataset:
path = untar_data(URLs.ADULT_SAMPLE)
- Run the following cell to get the output of
path.ls()
so that you can examine the directory structure of the dataset:Figure 2.8 – Output of path.ls()
- The dataset is in the
adult.csv
file. Run the following cell to ingest this CSV file into a pandas DataFrame:df = pd.read_csv(path/'adult.csv')
- Run the
head()
command to get a sample of records from the beginning of the dataset:Figure 2.9 – Sample of records from the beginning of the dataset
- Run the following command to get the number of records (rows) and fields (columns) in the dataset:
df.shape
- Run the following command to get the number of unique values in each column of the dataset. Can you tell from the output which columns are categorical?
df.nunique()
- Run the following command to get the count of missing values in each column of the dataset. Which columns have missing values?
df.isnull().sum()
- Run the following command to display some sample records from the subset of the dataset for people whose age is less than or equal to 40:
df_young = df[df.age <= 40] df_young.head()
Congratulations! You have ingested a tabular dataset curated by fastai and done a basic examination of the dataset.
How it works…
The dataset that you explored in this section, ADULT_SAMPLE
, is one of the datasets you would have seen in the source for URLs
in the previous section. Note that while the source for URLs
identifies which datasets are related to image or NLP (text) applications, it does not explicitly identify the tabular or recommender system datasets. ADULT_SAMPLE
is one of the datasets listed under main datasets
:
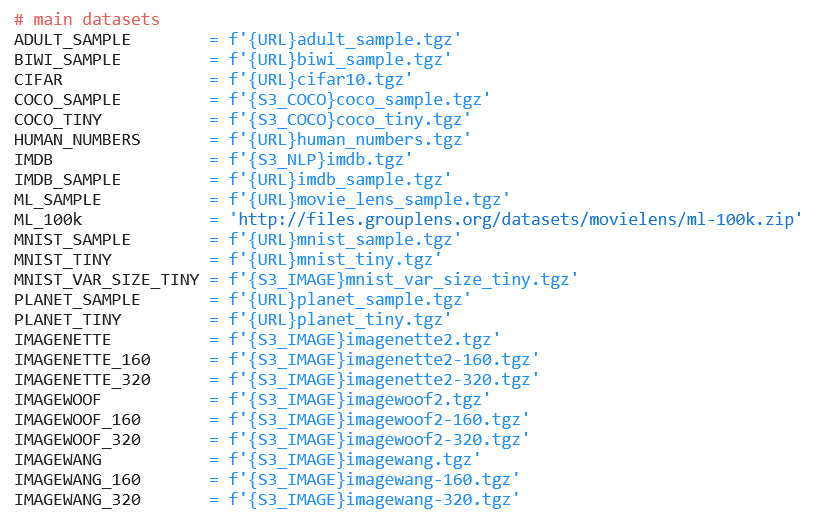
Figure 2.10 – Main datasets from the source for URLs
How did I determine that ADULT_SAMPLE
was a tabular dataset? First, the paper by Howard and Gugger (https://arxiv.org/pdf/2002.04688.pdf) identifies ADULT_SAMPLE
as a tabular dataset. Second, I just had to ingest it and try it out to confirm it could be ingested into a pandas DataFrame.
There's more…
What about the other curated datasets that aren't explicitly categorized in the source for URLs
? Here's a summary of the datasets listed in the source for URLs
under main datasets
:
- Tabular:
a)
ADULT_SAMPLE
- NLP (text):
a)
HUMAN_NUMBERSÂ Â
b)
IMDB
c)
IMDB_SAMPLEÂ Â Â Â Â Â
- Collaborative filtering:
a)
ML_SAMPLEÂ Â Â Â Â Â Â Â Â Â Â Â Â Â Â
b)
ML_100k                             Â
- Image data:Â Â
a) All of the other datasets listed in
URLs
undermain datasets
.