Once you are familiar with the topic of social media data, let us proceed to the next phase. The first step is to understand the process involved in exploitation of data present on social networks. A proper execution of the process, with attention to small details, is the key to good results. In many computer science domains, a small error in code will lead to a visible or at least correctable dysfunction, but in data science, it will produce entirely wrong results, which in turn will lead to incorrect conclusions.
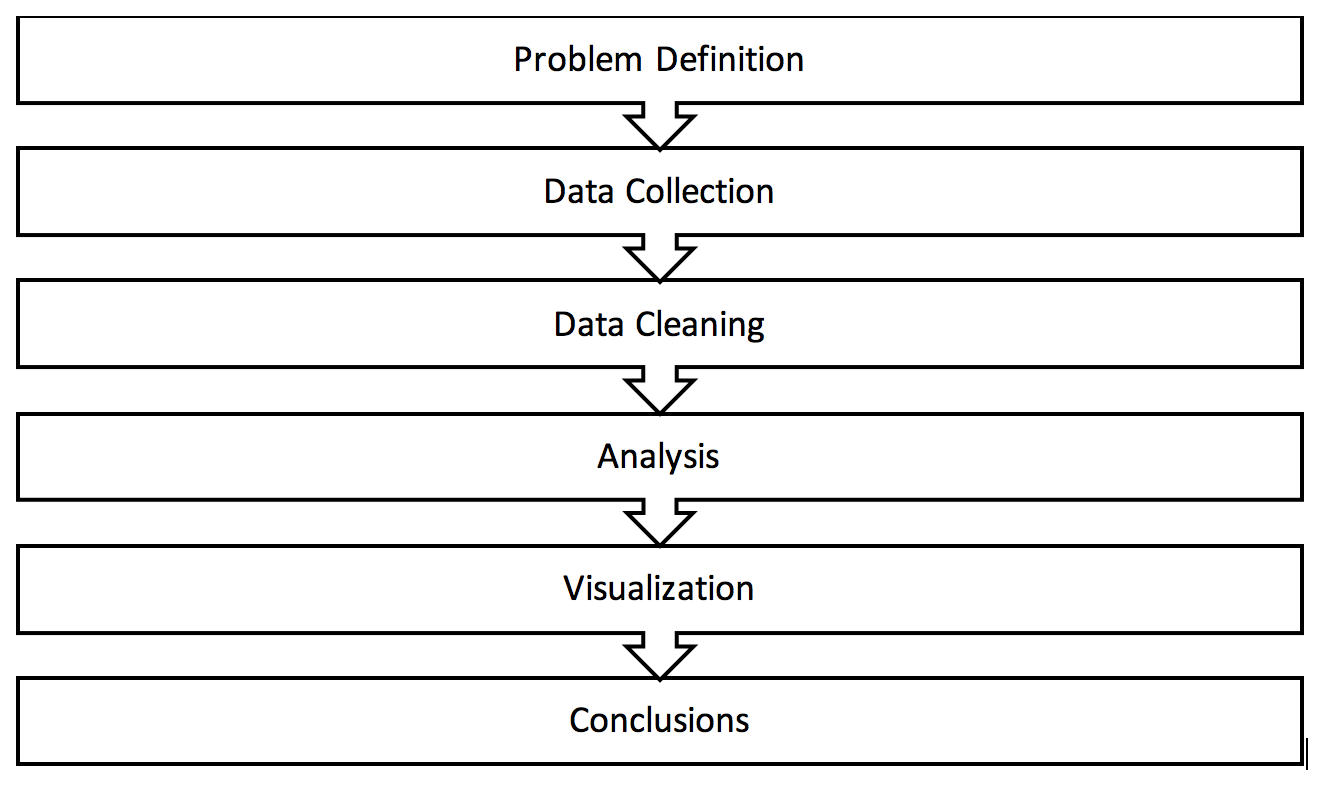
The very first step of data analysis is always problem definition. Understanding the problem is crucial for choosing the right data sources and the methods of analysis. It also helps to realize what kind of information and conclusions we can infer from the data and what is impossible to derive. This part is very often underestimated while it is key to successful data analysis.
Any question that we try to answer in a data science project has to be very precise. Some people tend to ask very generic questions, such as I want to find trends on Twitter. This is not a correct problem definition and an analysis based on such statement can fail in finding relevant trends. By a naive analysis, we can get repeating Twitter ads and content generated by bots. Moreover, it raises more questions than it answers. In order to approach the problem correctly, we have to ask in the first step: what is a trend? what is an interesting trend for us? and what is the time scope? Once we answer these questions, we can break up the problem in multiple sub problems: I'm looking for the most frequent consumer reactions about my brand on Twitter in English over the last week and I want to know if they were positive or negative. Such a problem definition will lead to a relevant, valuable analysis with insightful conclusions.
The next part of the process consists of getting the right data according to the defined problem. Many social media platforms allow users to collect a lot of information in an automatized way via APIs (Application Programming Interfaces), which is the easiest way to complete the task. However, other platforms, such as forums or blogs, usually require a customized programming approach (scraping), which will be explained in later chapters.
Once the data is stored in a database, we perform the cleaning. This step requires a precise understanding of the project's goals. In many cases, it will involve very basic tasks such as duplicates removal, for example, retweets on Twitter, or more sophisticated such as spam detection to remove irrelevant comments, language detection to perform linguistic analysis, or other statistical or machine learning approaches that can help to produce a clean dataset.
When the data is ready to be analyzed, we have to choose what kind of analysis and structure the data accordingly. If our goal is to understand the sense of the conversations, then it only requires a simple list of verbatims (textual data), but if we aim to perform analysis on different variables, like number of likes, dates, number of shares, and so on, the data should be combined in a structure such as data frame, where each row corresponds to an observation and each column to a variable.
The choice of the analysis method depends on the objectives of the study and the type of data. It may require statistical or machine learning approach, or a specific approach to time series. Different approaches will be explained on the examples of Facebook, Twitter, YouTube, GitHub, Pinterest, and Forum data, subsequently in the book.
Once the analysis is done, it's time to infer conclusions. We can derive conclusions based on the outputs from the models, but one of the most useful tools is visualization technique. Data and output can be presented in many different ways, starting from charts, plots, and diagrams through more complex 2D charts, to multidimensional visualizations. These techniques are shown in example chapters as well as the reasoning process to infer insightful conclusions.
Once the process is clear enough, we can start setting up the programming environment.