Evaluating classification performance
Beyond accuracy, there are several metrics we can use to gain more insight and to avoid class imbalance effects. These are as follows:
- Confusion matrix
- Precision
- Recall
- F1 score
- Area under the curve
A confusion matrix summarizes testing instances by their predicted values and true values, presented as a contingency table:
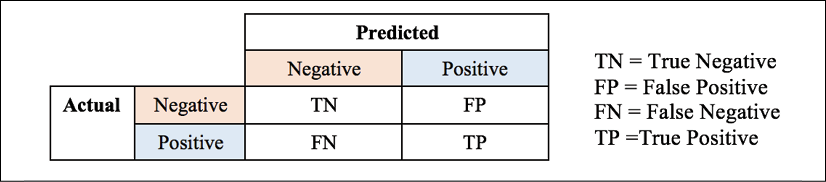
Table 2.3: Contingency table for a confusion matrix
To illustrate this, we can compute the confusion matrix of our Naïve Bayes classifier. We use the confusion_matrix
function from scikit-learn
to compute it, but it is very easy to code it ourselves:
>>> from sklearn.metrics import confusion_matrix
>>> print(confusion_matrix(Y_test, prediction, labels=[0, 1]))
[[ 60 47]
[148 431]]
As you can see from the resulting confusion matrix, there are 47 false positive cases (where the model misinterprets a dislike as a like...