As this book is aimed at developers, we think that the approach of explaining the mathematical concepts using real code comes naturally.
When choosing the programming language for the code examples, the first approach was to use multiple technologies, including some cutting-edge libraries. After consulting the community, it was clear that a simple language would be preferable when explaining the concepts.
Among the options, the ideal candidate would be a language that is simple to understand, with real-world machine learning adoption, and that is also relevant.
The clearest candidate for this task was Python, which fulfils all these conditions, and especially in the last few years has become the go-to language for machine learning, both for newcomers and professional practitioners.
In the following graph, we compare the previous star in the machine learning programming language field, R, and we can clearly conclude the huge, favorable tendency towards using Python. This means that the skills you acquire in this book will be relevant now and in the foreseeable future:
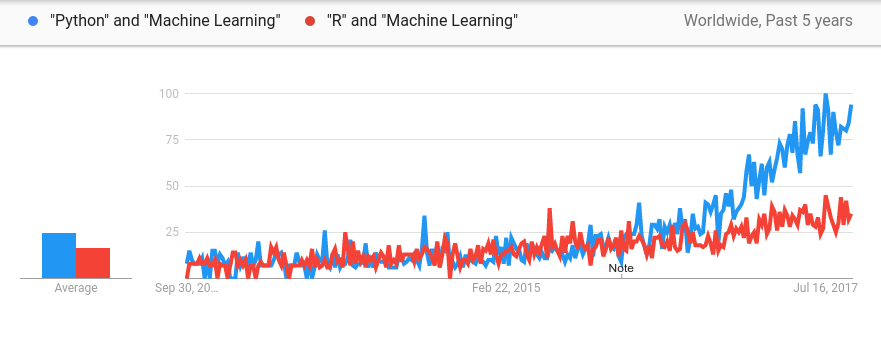
In addition to Python code, we will have the help of a number of the most well-known numerical, statistical, and graphical libraries in the Python ecosystem, namely pandas, NumPy, and matplotlib. For the deep neural network examples, we will use the Keras library, with TensorFlow as the backend.