What is DQN?
The objective of reinforcement learning is to find the optimal policy, that is, the policy that gives us the maximum return (the sum of rewards of the episode). In order to compute the policy, first we compute the Q function. Once we have the Q function, then we extract the policy by selecting an action in each state that has the maximum Q value. For instance, let's suppose we have two states A and B and our action space consists of two actions; let the actions be up and down. So, in order to find which action to perform in state A and B, first we compute the Q value of all state-action pairs, as Table 9.1 shows:
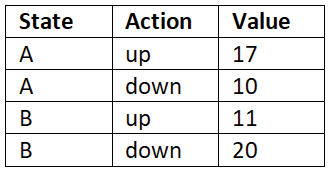
Table 9.1: Q-value of state-action pairs
Once we have the Q value of all state-action pairs, then we select the action in each state that has the maximum Q value. So, we select the action up in state A and down in state B as they have the maximum Q value. We improve the Q function on every iteration and once we have the optimal Q function, then...