An easy way to understand this concept is to imagine that you and your friends want to sort out piles of fruit into boxes. For that, you want to assign each person the task of going through one raw basket of fruit (all mixed up) and separating out the fruit into various boxes. Each person then does the same task of separating the fruit into the various types with this basket of fruit. In the end, you end up with a lot of boxes of fruit from all your friends. Then, you can assign a group to put the same kind of fruit together in a box, weigh the box, and seal the box for shipping. A classic example of showing the MapReduce framework at work is the word count example. The following are the various stages of processing the input data, first splitting the input across multiple worker nodes and then finally generating the output, the word counts:
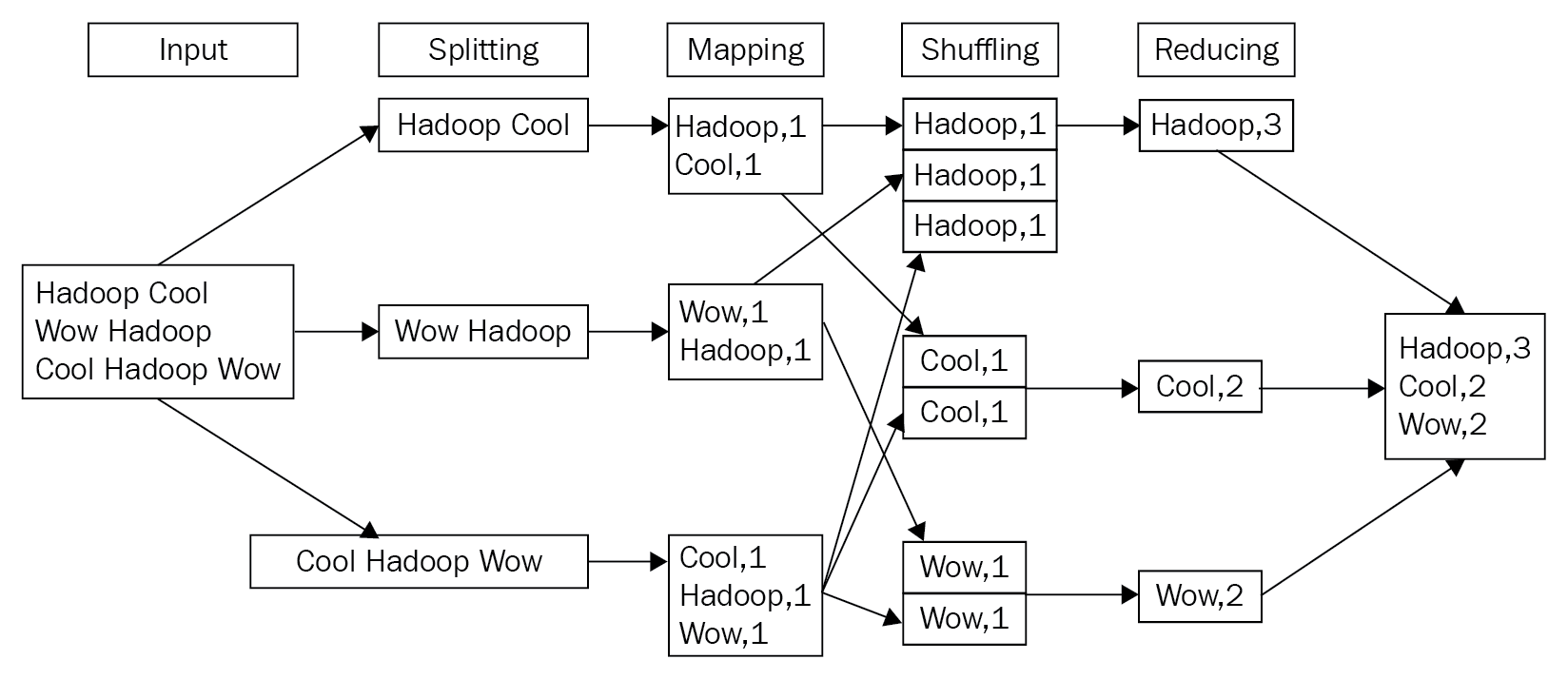
The MapReduce framework consists of a single ResourceManager and multiple NodeManagers (usually, NodeManagers coexist with the DataNodes of HDFS).Â