Addressing AI and data literacy challenges
What can one do to prepare themselves to survive and thrive in a world dominated by data and math models? Yes, the world will need more data scientists, data engineers, and ML engineers to design, construct, and manage AI models that will permeate society. But what about everyone else? We must train everyone to become Citizens of Data Science.
But what is data science? Data science seeks to identify and validate the variables and metrics that might be better predictors of performance to deliver more relevant, meaningful, and ethical outcomes.
To ensure that data science can adequately identify and validate those different variables and metrics that might be better predictors of performance, we need to ensure that we democratize the power of data science to leverage AI and big data in driving more relevant, meaningful, and ethical outcomes. We must ensure that AI and data don’t create a societal divide where only the high priesthood of data scientists, data engineers, and ML engineers prosper. We must ensure that they don’t benefit just those three-letter government agencies and the largest corporations.
We must prepare and empower everyone who is prepared to participate in and benefit from the business, operational, and societal benefits of AI. We must extend the AI Bill of Rights vision by championing a Citizens of Data Science mandate.
However, do you really understand your obligation to be a Citizen of Data Science? To quote my good friend John Morley on citizens and citizenship:
“Citizenship isn’t something that is bestowed upon us by an external, benevolent force. Citizenship requires action. Citizenship requires stepping up. Citizenship requires individual and collective accountability – accountability to continuous awareness, learning, and adaptation. Citizenship is about having a proactive and meaningful stake in building a better world.”
Building on an understanding of the active participation requirements of citizenship, let’s define the Citizens of Data Science mandate that will build our AI and data literacy journey:
Citizens of Data Science Mandate Ensuring that everyone, of every age and every background, has access to the education necessary to flourish in an age where economic growth and personal development opportunities are driven by AI and data. |
This Citizens of Data Science mandate would provide the training and pragmatic frameworks to ensure that AI and data’s power and potential are accessible and available to everyone, and that the associated risks are understood so that we not only survive but thrive in a world dominated by AI and data.
The AI and Data Literacy Framework
To become a Citizen of Data Science, we need a set of guidelines – a framework – against which to guide our personal and organizational development with respect to understanding how to thrive in a world dominated by AI and data.
A framework is a structured approach that provides guidance, rules, principles, and concepts for solving a specific problem or accomplishing a particular task. Think of a framework as providing guard rails, versus railroad tracks, that proactively guide and benchmark our personal and professional development.
The AI and Data Literacy Framework provides those guard rails – and the associated guidance, rules, principles, and concepts – to ensure that everyone is aware of and educated on their role in ensuring the responsible and ethical definition, design, development, and management of AI models. It is a framework designed to be equally accessible and understandable by everyone.
We will use the AI and Data Literacy Framework shown in Figure 1.2 throughout the book to help readers understand the different components of becoming Citizens of Data Science and ensure everyone has the skills and training to succeed in a world dominated by AI and data.
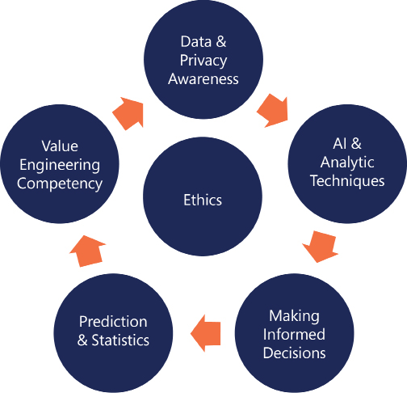
Figure 1.2: AI and Data Literacy Framework
This AI and Data Literacy Framework is comprised of six components:
- Data and privacy awareness discusses how your data is captured and used to influence and manipulate your thoughts, beliefs, and subsequent decisions. This section also covers personal privacy and what governments and organizations worldwide do to protect your data from misuse and abuse.
- AI and analytic techniques focuses on understanding the wide range of analytic algorithms available today and the problems they address. This chapter will explore the traditional, optimization-centric analytic algorithms and techniques so that our Citizens of Data Science understand what each does and when best to use which algorithms. And given the importance of AI, we will also have a separate chapter dedicated to learning-centric AI (Chapter 4). We will discuss how AI works, the importance of determining user intent, and the critical role of the AI utility function in enabling the AI model to continuously learn and adapt. This separate chapter dedicated to AI will also explore AI risks and challenges, including confirmation bias, unintended consequences, and AI model false positives and false negatives.
- Making informed decisions or decision literacy explores how humans can leverage basic problem-solving skills to create simple models to avoid ingrained human decision-making traps and biases. We will provide examples of simple but effective decision models and tools to improve the odds of making more informed, less risky decisions in an imperfect world.
- Prediction and statistics explains basic statistical concepts (probabilities, averages, variances, and confidence levels) that everyone should understand (if you watch sports, you should already be aware of many of these statistical concepts). We’ll then examine how simple stats can create probabilities that lead to more informed, less risky decisions.
- Value engineering competency provides a pragmatic framework for organizations leveraging their data with AI and advanced analytic techniques to create value. We will also provide tools to help identify and codify how organizations create value and the measures against which value creation effectiveness will be measured across a diverse group of stakeholders and constituents.
- AI ethics is the foundation of the AI and Data Literacy Framework. This book will explore how we integrate ethics into our AI models to ensure the delivery of unbiased, responsible, and ethical outcomes. We will explore a design template for leveraging economics to codify ethics that can then be integrated into the AI utility function, which guides the performance of AI models.
This book will explore each subject area as we progress, with a bonus chapter at the end! But before we begin the journey, we have a little homework assignment.
Assessing your AI and data literacy
No book is worth its weight if it doesn’t require its readers to participate.
Improving our AI and data literacy starts by understanding where we sit concerning the six components of our AI and data literacy educational framework. To facilitate that analysis, I’ve created the AI and data literacy radar chart. This chart can assess or benchmark your AI and data literacy and identify areas where you might need additional training.
A radar chart is a graphical method of capturing, displaying, and assessing multiple dimensions of information in a two-dimensional graph. A radar chart is simple in concept but very powerful in determining one’s strengths and weaknesses in the context of a more extensive evaluation. We will use the following AI and data literacy radar chart to guide the discussions, materials, and lessons throughout the book:
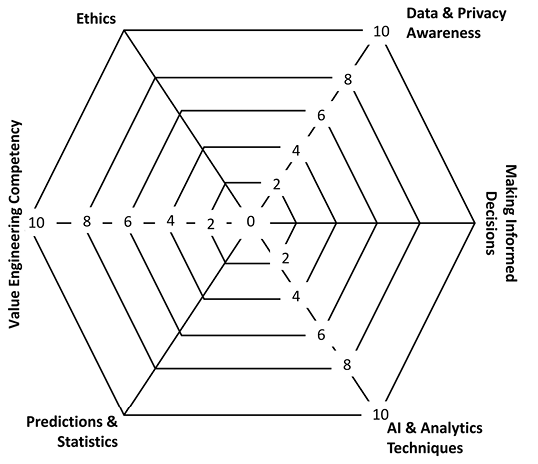
Figure 1.3: AI and data literacy radar chart
This assignment can also be done as a group exercise. A manager or business executive could use this exercise to ascertain their group’s AI and data literacy and use the results to identify future training and educational needs.
You will be asked to again complete the AI and data literacy radar chart at the end of the book to provide insights into areas of further personal exploration and learning.
In the following table are some guides to help you complete your AI and data literacy radar chart :
Category |
Low |
Medium |
High |
Data and privacy awareness |
Just click and accept the website and mobile app’s terms and conditions without reading |
Attempt to determine the credibility of the site or app before accepting the terms and downloading |
Read website and mobile app privacy terms and conditions and validate app and site credibility before engaging |
Informed decision-making |
Depend on their favorite TV channel, celebrities, or website to tell them what to think; prone to conspiracy theories |
Research issues before making a decision, though still overweigh the opinions of people who “think like me” |
Create a model that considers false positives and false negatives before making a decision; practice critical thinking |
AI and analytic techniques |
Believe that AI is something only applicable to large organizations and three-letter government agencies |
Understand how AI is part of a spectrum of analytics, but not sure what each analytic technique can do |
Understand how to collaborate to identify KPIs and metrics across a wide variety of value dimensions that comprise the AI utility function |
Predictions and statistics |
Don’t seek to understand the probabilities of events happening; blind to unintended consequences of decisions |
Do consider probabilities when making decisions but carry out a thorough assessment of the potential unintended consequences |
Actively seek out information from credible sources to improve the odds of making an informed decision |
Value engineering competency |
Don’t understand the dimensions of “value” |
Understand the value dimensions but haven’t identified the KPIs and metrics against which value creation effectiveness is measured |
Understand the value dimensions and have identified the KPIs and metrics against which value creation effectiveness is measured |
Ethics |
Think ethics is something that only applies to “others” |
Acknowledge the importance of ethics but are not sure how best to address it |
Proactively contemplate different perspectives to ensure ethical decisions and actions |
Table 1.1: AI and data literacy benchmarks
We are already using data to help us make more informed decisions!