The principles of text-to-video generation
The Stable Diffusion UNet, while effective for generating single images, falls short when it comes to generating consistent images due to its lack of contextual awareness. Researchers have proposed solutions to overcome this limitation, such as incorporating temporal information from the preceding one or two frames. However, this approach still fails to ensure pixel-level consistency, leading to noticeable differences between consecutive images and flickering in the generated video.
To address this inconsistency problem, the authors of AnimateDiff trained a separated motion model – a zero-initialized convolution side model – similar to the ControlNet model. Further, rather than controlling an image, the motion model is applied to a series of continuous frames, as shown in Figure 14.1:
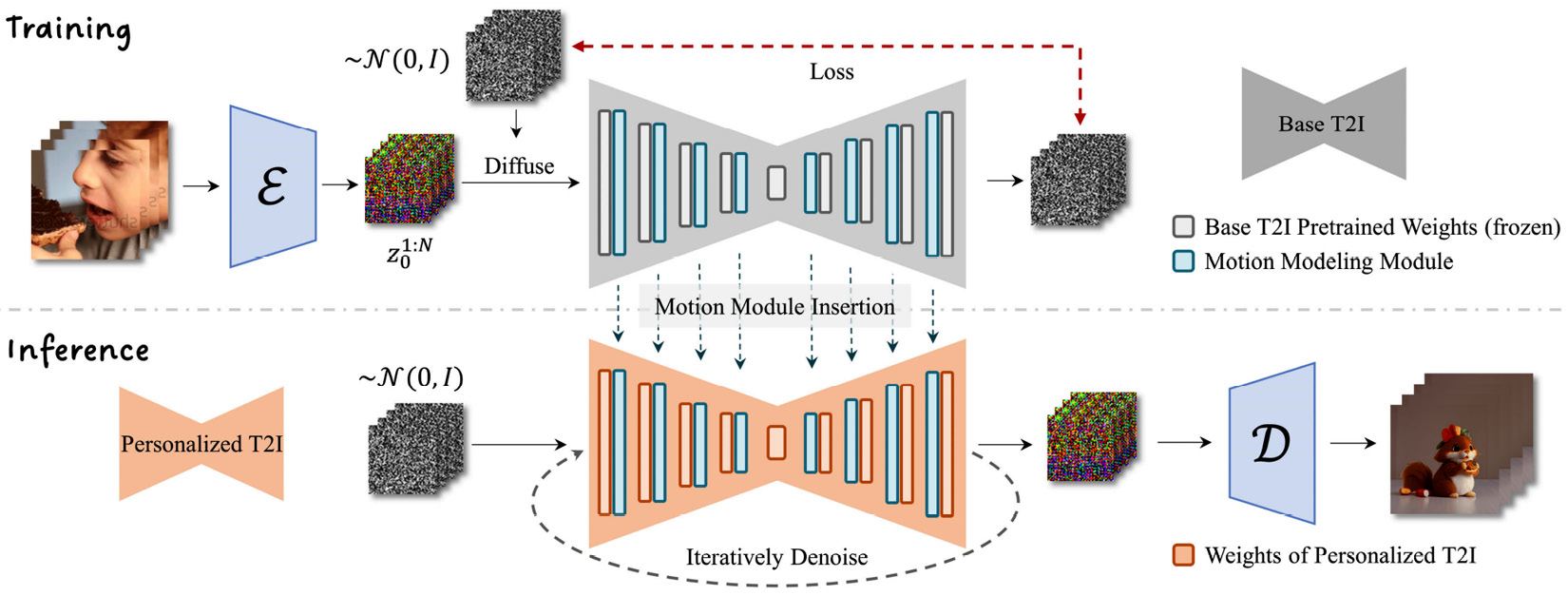
Figure 14.1: The architecture of AnimatedDiff
The process involves training a motion modeling module on video...