Unsupervised Learning versus Supervised Learning
Unsupervised learning is the field of practice that helps find patterns in cluttered data and is one of the most exciting areas of development in machine learning today. If you have explored machine learning bookwork before, you are probably familiar with the common breakout of problems in either supervised or unsupervised learning. Supervised learning encompasses the problem set of having a labeled dataset that can be used to either classify data (for example, predicting smokers and non-smokers, if you're looking at a lung health dataset) or finding a pattern in clearly defined data (for example, predicting the sale price of a home based on how many bedrooms it has). This model most closely mirrors an intuitive human approach to learning.
For example, if you wanted to learn how to not burn your food with a basic understanding of cooking, you could build a dataset by putting your food on the burner and seeing how long it takes (input) for your food to burn (output). Eventually, as you continue to burn your food, you will build a mental model of when burning will occur and how to avoid it in the future. Development in supervised learning was once fast paced and valuable, but it has simmered down in recent years. Many of the obstacles around getting to know your data have already been tackled and are listed in the following image:
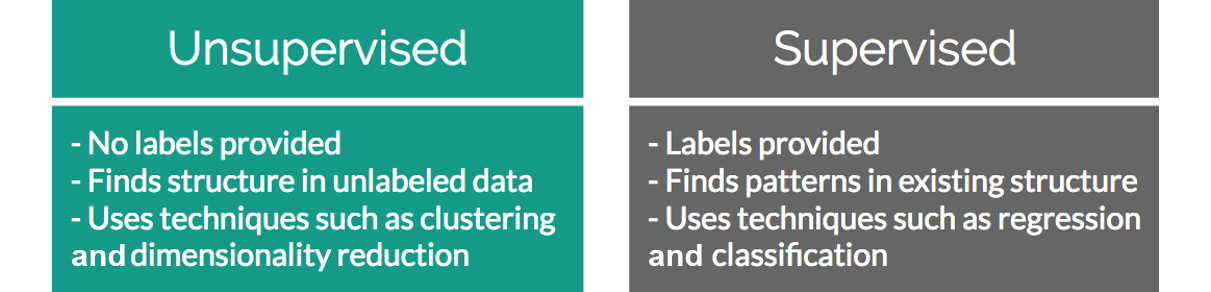
Figure 1.1: Differences between unsupervised and supervised learning
Conversely, unsupervised learning encompasses the problem set of having a tremendous amount of data that is unlabeled. Labeled data, in this case, would be data that has a supplied "target" outcome that you are trying to find the correlation to with supplied data. For instance, in the preceding example, you know that your "target outcome" is whether your food was burned; this is an example of labeled data. Unlabeled data is when you do not know what the "target" outcome is, and you have only supplied input data.
Building upon the previous example, imagine you were just dropped on planet Earth with zero knowledge of how cooking works. You are given 100 days, a stove, and a fridge full of food without any instructions on what to do. Your initial exploration of a kitchen could go in infinite directions. On day 10, you may finally learn how to open the fridge; on day 30, you may learn that food can go on the stove; and after many more days, you may unwittingly make an edible meal. As you can see, trying to find meaning in a kitchen devoid of adequate informational structure leads to very noisy data that is completely irrelevant to actually preparing a meal.
Unsupervised learning can be an answer to this problem. Looking back at your 100 days of data, you can use clustering to find patterns of similar attributes across days and deduce which foods are similar and may lead to a "good" meal. However, unsupervised learning isn't a magical answer. Simply finding clusters can be just as likely to help you find pockets of similar, yet ultimately useless, data. Expanding on the cooking example, we can illustrate this shortcoming with the concept of the "third variable". Just because you have a cluster of really great recipes doesn't mean they are infallible. During your research, you may have found a unifying factor that all good meals were cooked on a stove. This does not mean that every meal cooked on a stove will be good, and you cannot easily jump to that conclusion for all future scenarios.
This challenge is what makes unsupervised learning so exciting. How can we find smarter techniques to speed up the process of finding clusters of information that are beneficial to our end goals? The following sections would help us answer this question.