Image Augmentation
Image augmentation is the process of modifying images to increase the number of training examples available. This process can include zooming in on the image, rotating the image, or flipping the image vertically or horizontally. This can be performed if the augmentation process does not change the context of the image. For example, an image of a banana, when flipped horizontally, is still recognizable as a banana, and new images of bananas are likely to be of either orientation. In this case, providing a model for both orientations during the training process will help build a robust model.
However, if you have an image of a boat, it may not be appropriate to flip it vertically, as this does not represent how boats commonly exist in images, upside-down. Ultimately the goal of image augmentation is to increase the number of training images that resemble the object in its everyday occurrence, preserving the context. This will help the trained model perform well on new, unseen images. An example of image augmentation can be seen in the following figure, in which an image of a banana has been augmented three times; the left image is the original image, and those on the right are the augmented images.
The top-right image is the original image flipped horizontally, the middle-right image is the original image zoomed in by 15%, and the bottom-right image is the original image rotated by 10 degrees. After this augmentation process, you have four images of a banana, each of which has the banana in different positions and orientations:
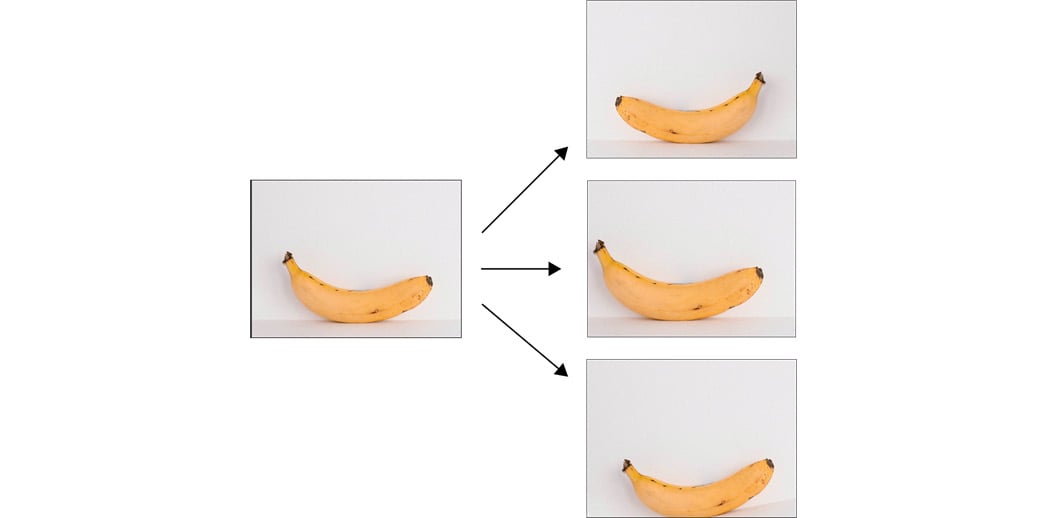
Figure 2.13: An example of image augmentation
Image augmentation can be achieved with TensorFlow's ImageDataGenerator
class when the images are loaded with each batch. Similar to image rescaling, various image augmentation processes can be applied. The arguments for common augmentation processes include the following:
horizontal_flip
: Flips the image horizontally.vertical_flip
: Flips the image vertically.rotation_range
: Rotates the image up to a given number of degrees.width_shift_range
: Shifts the image along its width axis up to a given fraction or pixel amount.height_shift_range
: Shifts the image along its height axis up to a given fraction or pixel amount.brightness_range
: Modifies the brightness of the image up to a given amount.shear_range
: Shears the image up to a given amount.zoom_range
: Zooms in the image up to a given amount.
Image augmentation can be applied when instantiating the ImageDataGenerator
class, as follows:
datagenerator = ImageDataGenerator(rescale = 1./255,\ shear_range = 0.2,\ rotation_range= 180,\ zoom_range = 0.2,\ horizontal_flip = True)
In the following activity, you perform image augmentation using TensorFlow's ImageDataGenerator
class. The process is as simple as passing in parameters. You will use the same dataset that you used in Exercise 2.03, Loading Image Data for Batch Processing, which contains images of boats and airplanes.
Activity 2.02: Loading Image Data for Batch Processing
In this activity, you will load image data for batch processing and augment the images in the process. The image_data
folder contains a set of images of boats and airplanes. You are required to load in image data for batch processing and adjust the input data with random perturbations such as rotations, flipping the image horizontally, and adding shear to the images. This will create additional training data from the existing image data and will lead to more accurate and robust machine learning models by increasing the number of different training examples even if only a few are available. You are then tasked with printing the labeled images of a batch from the data generator.
The steps for this activity are as follows:
- Open a new Jupyter notebook to implement this activity.
- Import the
ImageDataGenerator
class fromtensorflow.keras.preprocessing.image
. - Instantiate
ImageDataGenerator
and set therescale=1./255
,shear_range=0.2
,rotation_range=180
,zoom_range=0.2
, andhorizontal_flip=True
arguments. - Use the
flow_from_directory
method to direct the data generator to the images while passing in the target size as64x64
, a batch size of25
, and the class mode asbinary
. - Create a function to display the first 25 images in a 5x5 array with their associated labels.
- Take a batch from the data generator and pass it to the function to display the images and their labels.
Note
The solution to this activity can be found via this link.
In this activity, you augmented images in batches so they could be used for training ANNs. You've seen that when images are used as input, they can be augmented to generate a larger number of effective training examples.
You learned how to load images in batches, which enables you to train on huge volumes of data that may not fit into the memory of your machine at one time. You also learned how to augment images using the ImageDataGenerator
class, which essentially generates new training examples from the images in your training set.
In the next section, you will learn how to load and preprocess text data.