Data Preprocessing
Data preprocessing refers to the process in which raw data is converted into a form that is appropriate for machine learning models to use as input. Each different data type will require different preprocessing steps, with the minimum requirement that the resulting tensor is composed solely of numerical elements, such as integers or decimal numbers. Numerical tensors are required since models rely on linear transformations such as addition and multiplication, which can only be performed on numerical tensors.
While many datasets exist with solely numerical fields, many do not. They may have fields that are of the string, Boolean, categorical, or date data types that must all be converted into numerical fields. Some may be trivial; a Boolean field can be mapped so that true
values are equal to 1
and false
values are equal to 0
. Therefore, mapping a Boolean field to a numerical field is simple and all the necessary information is preserved. However, when converting other data types, such as date fields, you may lose information when converting into numerical fields unless it's explicitly stated otherwise.
One example of a possible loss of information occurs when converting a date field into a numerical field by using Unix time. Unix time represents the number of seconds that have elapsed since the Unix epoch; that is, 00:00:00 UTC on January 1, 1970, and leap seconds are ignored. Using Unix time removes the explicit indication of the month, day of the week, hour of the day, and so on, which may act as important features when training a model.
When converting fields into numerical data types, it is important to preserve as much informational context as possible as it will aid any model that is trained to understand the relationship between the features and the target. The following diagram demonstrates how a date field can be converted into a series of numerical fields:
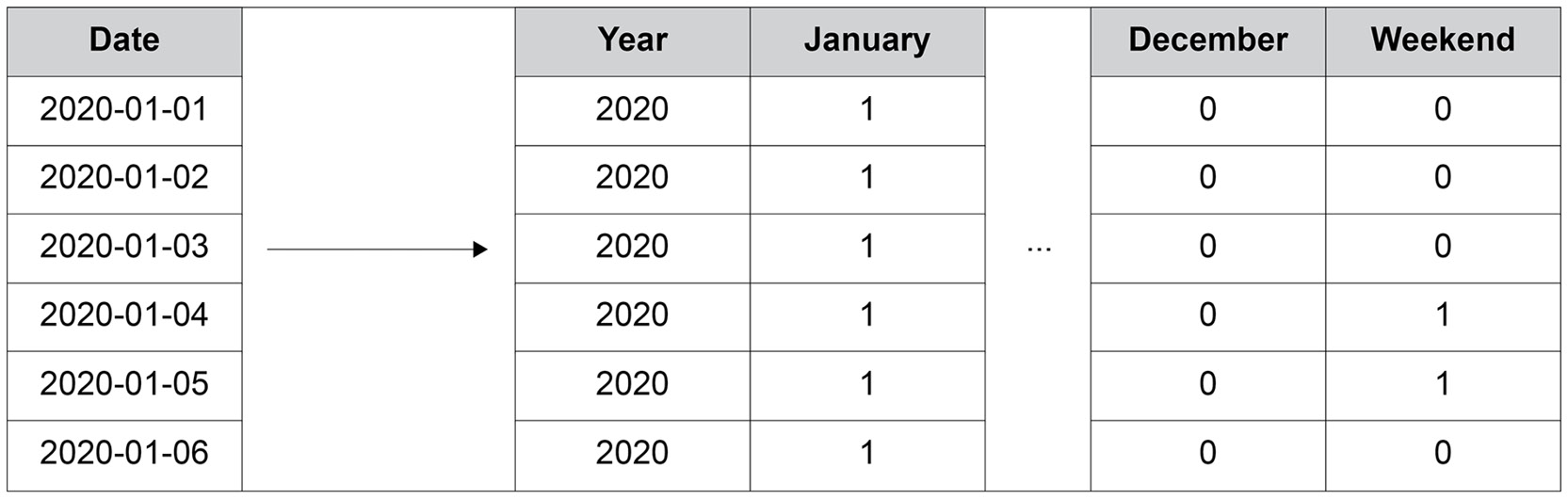
Figure 2.4: A numerical encoding of a date column
As shown in the preceding diagram, on the left, the date field represents a given date, while on the right, there is a method providing numerical information:
- The year is extracted from the date, which is an integer.
- The month is one-hot encoded. There is a column for each month of the year and the month is binary encoded, if the date's month corresponds with the column's name.
- A column is created indicating whether the date occurs on a weekend.
This is just a method to encode the date
column here; not all the preceding methods are necessary and there are many more that can be used. Encoding all the fields into numerical fields appropriately is important to create performant machine learning models that can learn the relationships between the features and the target.
Data normalization is another preprocessing technique used to speed up the training process. The normalization process rescales the fields so that they are all of the same scale. This will also help ensure that the weights of the model are of the same scale.
In the preceding diagram, the year
column has the order of magnitude 10
3, and the other columns have the order 10
0. This implies there are three orders of magnitude between the columns. Fields with values that are very different in scale will result in a less accurate model as the optimal weights to minimize the error function may not be discovered. This may be due to the tolerance limits or the learning rate that are defined as hyperparameters prior to training not being optimal for both scales when the weights are updated. In the preceding example, it may be beneficial to rescale the year
column so that it has the same order of magnitude as the other columns.
Throughout this chapter, you will explore a variety of methods that can be used to preprocess tabular data, image data, text data, and audio data so that it can be used to train machine learning models.