PV-DBOW
Figure 8.2 is the neural network for PV-DBOW that has an input layer, a hidden layer, and an output layer. The input layer is a vector of the paragraph IDs. Assume a corpus has 500 paragraphs. Each of the paragraph IDs is one-hot encoded and the length of each paragraph vector is 500. For example, Paragraph “1” is a 1 x 500 vector where only the position of “1” is 1 and the rest are zeros.
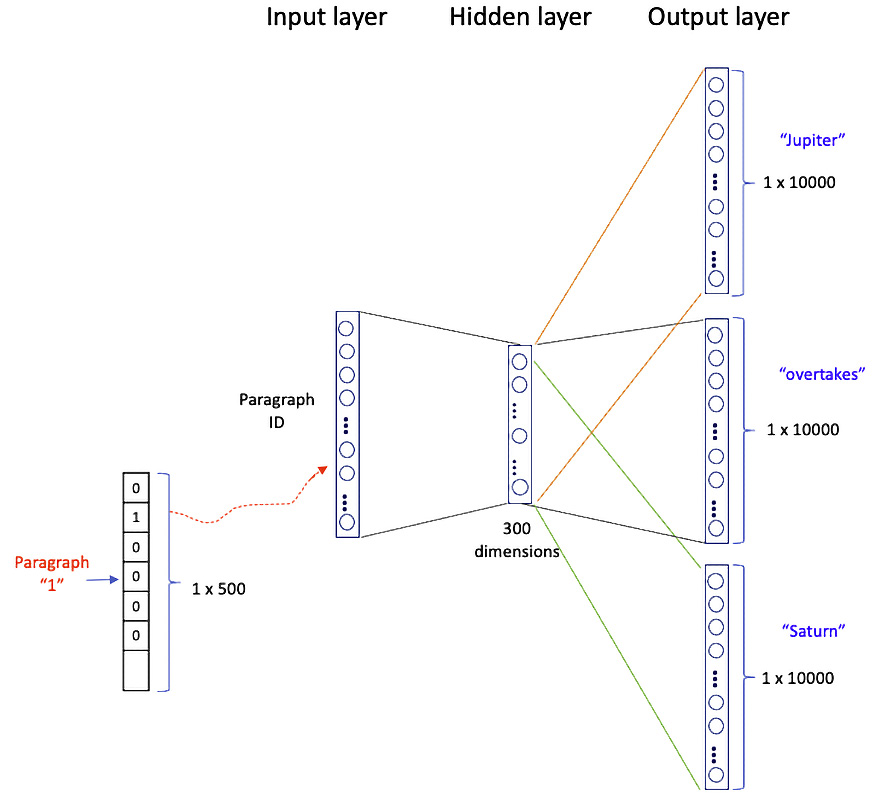
Figure 8.2 – PV-DBOW
Let’s see how a paragraph is prepared to feed into the neural network model.
The neural network requires data to follow the (input, output) format. Let’s first see how to do this. In Word2Vec, we organize texts into word pairs to feed into its neural network model. Its format is (word, adjacent word) for the input and output layer. In Doc2Vec, the format for the word pairs is (paragraph ID, word) for the input and output layer. Assume we have two paragraphs:
- Paragraph 1: “...