Training the network
Stochastic gradient descent (SGD) is an effective way of training deep neural networks. SGD seeks such parameters Θ of the network, which minimize the loss function ℒ.
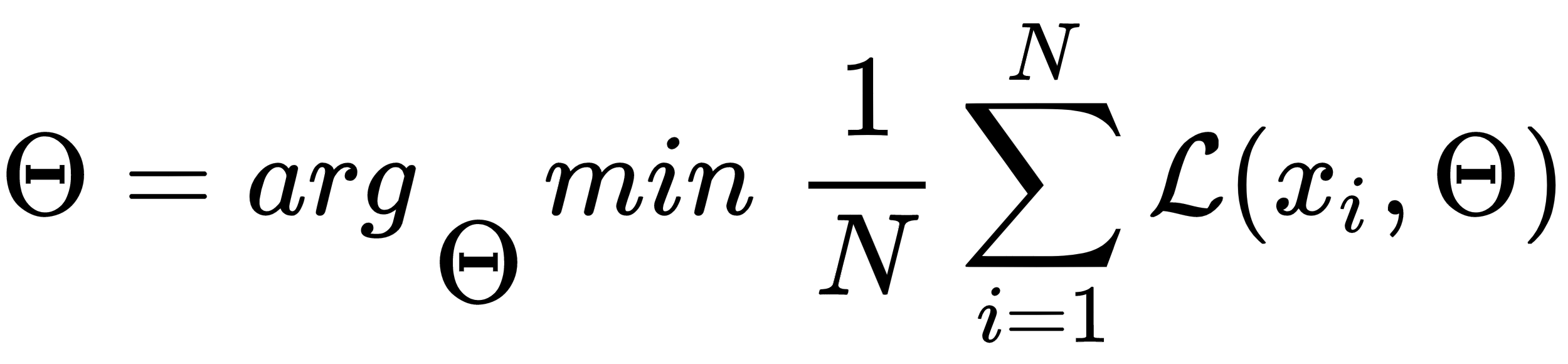
Where
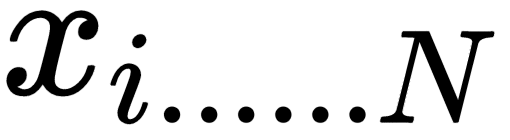
is a training dataset.
Training happens in steps. At every step, we choose a subset of our training set of size m (mini-batch) and use it to approximate loss function gradient with respect to parameters Θ:
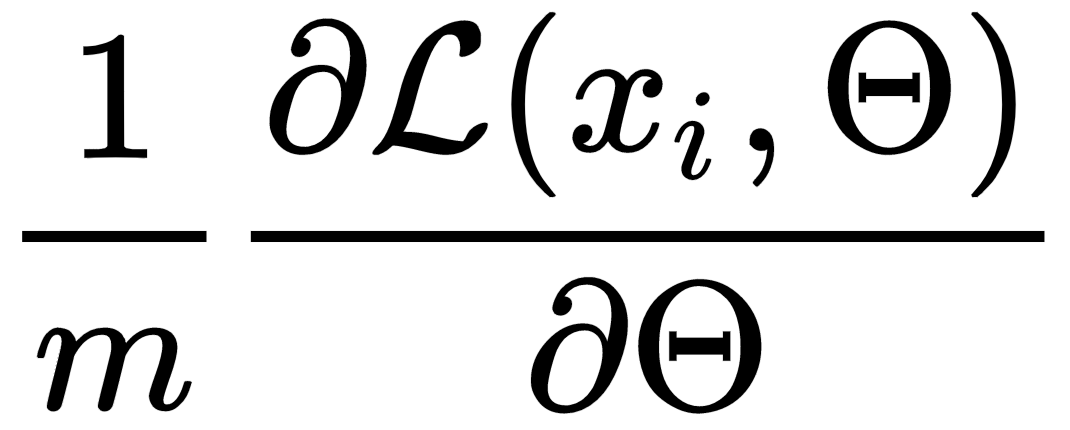
Mini-batch training advantages are as follows:
- Gradient of the loss function over a mini-batch is a better approximation of the gradient over the whole training set then calculated over only one sample
- Thanks to the GPU you can perform computations in parallel on every sample in the batch, which is faster, then processing them one-by-one